Abstract
The aim of this study is to make a comparison of the performances of two machine-learning algorithms that support vector machine (SVM) and random forest (RF) for landslide susceptibility mapping. The study makes use of a sampling strategy called two-level random sampling (2LRS). During landslide susceptibility mapping, training and testing samples must be collected from different landslide seed cells, which are then put through a fully independent sampling using the 2LRS algorithm. This approach requires fewer samples for the improvement of the computation time of both machine-learning classifications. The proposed approach was tested in the Alakir catchment area (Western Antalya, Turkey) which features numerous active deep-seated rotational landslides. In order to compare the performance of the machine-learning algorithms, three random sets were generated for SVM and three random sets generated for 10, 100, 1000 and 10,000-tree size RF. A total of 15 models were generated for comparison, and their spatial performances were performed by the area under the receiver-operating characteristic curves, which ranged between 0.82 and 0.87. The highest and lowest performances were recorded from two models in SVM and two models from the 1000-tree and 10,000-tree sized RF, respectively. These results were confirmed the landslide happened just after producing the susceptibility maps in the field.







Similar content being viewed by others
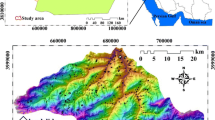
References
Abrams M (2000) The advanced spaceborne thermal emission and reflection radiometer (ASTER): data products for the high spatial resolution imager on NASA’s Terra platform. Int J Remote Sens 21:847–859
Akgun A, Sezer EA, Nefeslioglu HA, Gokceoglu C, Pradhan B (2012) An easy-touse MATLAB program (MamLand) for the assessment of landslide susceptibility using a Mamdani fuzzy algorithm. Comput Geosci 38:23–34
Ayalew L, Yamagishi H (2005) The application of GIS-based logistic regression for landslide susceptibility mapping in the Kakuda-Yahiko Mountains, Central Japan. Geomorphology 65:15–31
Baeza C, Corominas J (2001) Assessment of shallow landslide susceptibility by means of multivariate statistical techniques. Earth Surf Proc Land 26:1251–1263
Beguería S (2006) Validation and evaluation of predictive models in hazard assessment and risk management. Nat Hazards 37:315–329
Breiman L (2001) Random forests. Mach Learn 45(1):5–32
Brenning A (2005) Spatial prediction models for landslide hazards: review, comparison and evaluation. Nat Hazards Earth Syst Sci 5:853–862
Bui D, Pradhan B, Lofman O, Revhaug I, Dick O (2012) Landslide susceptibility mapping at Hoa Binh province (Vietnam) using an adaptive neuro fuzzy inference system and GIS. Comput Geosci 45:199–211
Can T, Nefeslioglu HA, Gokceoglu C, Sonmez H, Duman TY (2005) Susceptibility assessments of shallow earthflows triggered by heavy rainfall at three subcatchments by logistic regression analyses. Geomorphology 72:250–271
Chen CW, Chen H, Oguchi T (2016) Distributions of landslides, vegetation, and related sediment yields during typhoon events in northwestern Taiwan. Geomorphology 273:1–13
Chen W, Xie X, Wang J, Pradhan B, Hong H, Bui DT, Duan Z, Ma J (2017) A comparative study of logistic model tree, random forest, and classification and regression tree models for spatial prediction of landslide susceptibility. CATENA 151:147–160
CNNTurk, (2017) Landslide in Antalya destroyed 8 houses, http://www.cnnturk.com/video/turkiye/antalyada-heyelan-8-evi-yikti. Accessed 20 March 2017 (in Turkish)
Dagdelenler G, Nefeslioglu HA, Gokceoglu C (2016) Modification of seed cell sampling strategy for landslide susceptibility mapping: an application from the Eastern part of the Gallipoli Peninsula (Canakkale, Turkey). Bull Eng Geol Env 75:575–590
Das I, Sahoo S, van Westen C, Stein A, Hack R (2010) Landslide susceptibility assessment using logistic regression and its comparison with a rock mass classification system, along a road section in the northern Himalayas (India). Geomorphology 114:627–637
Das I, Stein A, Kerle N, Dadhwal VK (2012) Landslide susceptibility mapping along road corridors in the Indian Himalayas using Bayesian logistic regression models. Geomorphology 179:116–125
Domínguez-Cuesta M, Jiménez-Sánchez M, Berrezueta E (2007) Landslides in the Central Coalfield (Cantabrian Mountains, NW Spain): geomorphological features, conditioning factors and methodological implications in susceptibility assessment. Geomorphology 89:358–369
Duman TY, Can T, Emre Ö, Kecer M, Dogan A, Ates S, Durmaz S (2005) Landslide inventory of northwestern Anatolia, Turkey. Eng Geol 77:99–114
Duman TY, Can T, Gokceoglu C, Nefeslioglu HA, Sonmez H (2006) Application of logistic regression for landslide susceptibility zoning of Cekmece Area, Istanbul, Turkey. Environ Geol 51:241–256
Duman TY, Olgun S, Can T, Nefeslioglu HA, Hamzacebi S, Elmaci H, Durmaz S, Corekcioglu S (2009) 1:500,000 Scaled Landslide Inventory Maps of Turkey, Konya Quarter. General Directorate of Mineral Research and Exploration, Ankara, p 22
Ercanoglu M, Gokceoglu C (2004) Use of fuzzy relations to produce landslide susceptibility map of a landslide prone area (West Black Sea Region, Turkey). Eng Geol 75:229–250
Foody GM, Mathur A, Sanchez-Hernandez C, Boyd DS (2006) Training set size requirements for the classification of a specific class. Remote Sens Environ 104:1–14
Gillespie A, Kahle A, Walker R (1987) Color enhancement of highly correlated images. I. Decorrelation and HSI contrast stretches. Remote Sens Environ 20:209–235
Gislason PO, Benediktsson JA, Sveinson JR (2006) Random forests for land cover classification. Pattern Recogn Lett 27(4):294–300
Goetz JN, Brenning A, Petschko H, Leopold P (2015) Evaluating machine learning and statistical prediction techniques for landslide susceptibility modeling. Comput Geosci 81:1–11
Gökce O, Özden S, Demir A (2008) Spatial and statistical distribution of disasters in Turkey. In: Inventory of disaster data. The Ministry of Public Works and Settlement, General Directorate of Disaster Affairs, Department of Disaster Investigation and Damage Assessment, Ankara, p 126 (in Turkish)
Gokceoglu C, Ercanoglu M (2001) Heyelan duyarlilik haritalarinin hazirlanmasinda kullanilan parametrelere iliskin belirsizlikler. Yerbilimleri 23:189–206 (in Turkish)
Gomez H, Kavzoglu T (2005) Assessment of shallow landslide susceptibility using artificial neural networks in Jabonosa River Basin, Venezuela. Eng Geol 78:11–27
Gonzalez-Ollauri A, Mickovski SB (2017) Hydrological effect of vegetation against rainfall-induced landslides. J Hydrol 549:374–387
Guzzetti F, Carrarra A, Cardinali M, Reichenbach P (1999) Landslide hazard evaluation: a review of current techniques and their application in a multi-scale study, Central Italy. Geomorphology 31:181–216
Heakmann T, Gegg K, Gegg A, Becht M (2014) Sample size matters: investigating the effect of sample size on a logistic regression susceptibility model for debris flows. Nat Hazards Earth Syst Sci 14:259–278
Hirano A, Welcha R, Langb H (2003) Mapping from ASTER stereo image data: dEM validation and accuracy assessment. ISPRS J Photogramm Remote Sens 57(5–6):356–370
Hussin HY, Zunpano V, Reichenbach P, Sterlacchini S, Micu M, Van Westen C, Bălteanu D (2016) Different landslide sampling strategies in a grid-based bi-variate statistical susceptibility mode. Geomorphology 253:508–523
Kiss R (2004) Determination of drainage network in digital elevation model, utilities and limitations. J Hung Geomath 2:16–29
Koc-San D (2013) Evaluation of different classification techniques for the detection of glass and plastic greenhouses from WorldView-2 satellite imagery. J Appl Remote Sens 7:073553-1–073553-20
Lee S, Dan NT (2005) Probabilistic landslide susceptibility mapping in the Lai Chau province of Vietnam: focus on the relationship between tectonic fractures and landslides. Environ Geol 48:778–787
Lee S, Min K (2001) Statistical analysis of landslide susceptibility at Yongin, Korea. Environ Geol 40(1095–1113):263
Lee S, Pradhan B (2007) Landslide hazard mapping at Selangor, Malaysia using frequency ratio and logistic regression models. Landslides 4:33–41
Lee S, Ryu J, Won J, Park H (2004) Determination and application of the weights for landslide susceptibility mapping using an artificial neural network. Eng Geol 71:289–302
Marjanovic M, Kovacevic M, Bajat B, Vozenílek V (2011) Landslide susceptibility assessment using SVM machine learning algorithm. Eng Geol 123:225–234
Mathur A, Foody GM (2007) Crop classification by support vector machine with intelligently selected training data for an operational application. Int J Remote Sens 29(8):2227–2240
MGM (2017) General directorate of meteorology, Turkey. On-line Meteorological Access https://mgm.gov.tr/veridegerlendirme/il-ve-ilceler-istatistik.aspx?m=ANTALYA. Accessed 17 Aug 2017
Milliyet (2017a) 12 houses evacuated due to landslide in Kumluca (County), http://www.milliyet.com.tr/kumluca-da-heyelan-nedeniyle-12-ev-gundem-2417651/. Accessed 21 March 2017 (in Turkish)
Milliyet (2017b) The landslide’s hurt in Kumluca County, http://www.milliyet.com.tr/kumluca-daki-heyelanin-yaralari-sariliyor-antalya-yerelhaber-1925025/. Accessed 22 March 2017 (in Turkish)
Moore ID, Burch GJ (1986) Sediment transport capacity of sheet and rill flow: application of unit stream power theory. Water Resour Res 22:1350–1360
Moore ID, Wilson JP (1992) Length-slope factors for the revised universal soil loss equation: simplified method for estimation. J Soil Water Conserv 47:423–428
Moore ID, Grayson RB, Ladson A (1991) Digital terrain modeling: a review of hydrological, geomorphological, and biological applications. Hydrol Process 5:3–30
Mountrakis G, Im J, Ogole C (2011) Support vector machines in remote sensing: a review. ISPRS J Photogramm Remote Sens 66:247–259
Nefeslioglu HA, Gokceoglu C, Sonmez H (2008) An assessment on the use of logistic regression and artificial neural networks with different sampling strategies for the preparation of landslide susceptibility maps. Eng Geol 97:171–191
Nefeslioglu HA, Sezer E, Gokceoglu C, Bozkir AS, Duman TY (2010) Assessment of landslide susceptibility by decision trees in the metropolitan area of Istanbul, Turkey. Math Probl Eng 2010:1–15
Nefeslioglu HA, Gokceoglu C, Sonmez H, Gorum T (2011) Medium-scale hazard mapping for shallow landslide initiation: the Buyukkoy catchment area (Cayeli, Rize, Turkey). Landslides 8:459–483
Nefeslioglu HA, San BT, Gokceoglu C, Duman TY (2012) An assessment on the use of Terra ASTER L3A data in landslide susceptibility mapping. Int J Appl Earth Obs Geoinf 14:40–60
Oh HJ, Pradhan B (2011) Application of a neuro-fuzzy model to landslide susceptibility mapping for shallow landslides in a tropical hilly area. Comput Geosci 37:1264–1276
Olaya V (2004) A Gentle introduction to SAGA GIS. Reference list. http://www.saga-gis.org/en/about/references.html. Accessed 21 March 2017
Petschko H, Bell R, Leopold P, Heiss G, Glade T (2013) Landslide inventories for reliable susceptibility maps. In: Margottini C, Canuti P, Sassa K (eds) Landslide science and practice, vol. 1: landslide inventory and susceptibility and hazard zoning. Springer, Berlin
Petschko H, Brenning A, Bell R, Goetz J, Glade T (2014) Assessing the quality of landslide susceptibility maps—case study Lower Austria. Nat Hazards Earth Syst Sci 14:95–118. doi:10.5194/nhess-14-95-2014
Pham BT, Pradhan B, Bui DT, Prakash I, Dholakia MB (2016) A comparative study of different machine learning methods for landslide susceptibility assessment: a case study of Uttarakhand area (India). Environ Model Softw 84:240–250
Pour AB, Hashim M (2011) Application of advanced spaceborne thermal emission and reflection radiometer (ASTER) data in geological mapping. Int J Phys Sci 6(33):7657–7668
Pourghasemi HR, Mohammady M, Pradhan B (2012) Landslide susceptibility mapping using index of entropy and conditional probability models in GIS: Safarood Basin, Iran. Catena 97:71–84
Pradhan B (2013) A comparative study on the predictive ability of the decision tree, support vector machine and neuro-fuzzy models in landslide susceptibility mapping using GIS. Comput Geosci 51:350–365
Pradhan B, Lee S (2010) Landslide susceptibility assessment and factor effect analysis: backpropagation artificial neural networks and their comparison with frequency ratio and bivariate logistic regression modelling. Environ Model Softw 25:747–759
Pradhan B, Lee S, Buchroithner M (2009) Use of geospatial data for the development of fuzzy algebraic operators to landslide hazard mapping: a case study in Malaysia. Appl Geomat 1:3–15
Rabe A, Van der Linden S, Hostert P (2010) imageSVM, Version 2.1. www.hu-geomatics.de. Accessed 23 Aug 2013
Rodriguez-Galiano VF, Ghimire B, Rogan B, Chica-Olmo M, Rigol-Sanchez JP (2012) An assessment of the effectiveness of a random forest classifier for land-cover classification. ISPRS J Photogramm Remote Sens 67:93–104
Rowan L, Mars J (2003) Lithologic mapping in the Mountain Pass, California area using advanced spaceborne thermal emission and reflection radiometer (ASTER) data. Remote Sens Environ 84:350–366
Saito H, Nakayama D, Matsuyama H (2009) Comparison of landslide susceptibility based on a decision-tree model and actual landslide occurrence: the Akaishi Mountains, Japan. Geomorphology 109:108–121
San BT (2014) An evaluation of SVM using polygon-based random sampling in landslide susceptibility mapping: the Candir catchment area (western Antalya, Turkey). Int J Appl Earth Obs Geoinf 26:399–412
San BT, Süzen ML (2005) Digital elevation model (DEM) generation and accuracy assessment from ASTER stereo data. Int J Remote Sens 26(22):5013–5027
San BT, Sumer EO, Gurcay B (2004) Comparison of band ratioing and spectral indices methods for detecting alunite and kaolinite minerals using aster data in Biga region, Turkey. In: XXth ISPRS Congress, July 12–23 2004 Istanbul, Turkey, Commission 7, pp 77–82
Santini M, Grimaldi S, Nardi F, Petroselli A, Rulli MC (2009) Pre-processing algorithms and landslide modelling on remotely sensed DEMs. Geomorphology 113:110–125
Senel M (1997) 1:100,000 scaled geological maps of Turkey, No. 7, Antalya—L10 Map. General Directorate of Mineral Research and Exploration, Ankara, Turkey (in Turkish)
Shen P, Zhang LM, Chen HX, Gao L (2017) Role of vegetation restoration in mitigating hillslope erosion and debris flows. Eng Geol 216:122–133
Süzen ML, Doyuran V (2004) Data driven bivariate landslide susceptibility assessment using geographical information systems: a method and application to Asarsuyu catchment, Turkey. Eng Geol 71:303–321
Süzen ML, Kaya BS (2012) Evaluation of environmental parameters in logistic regression models for landslide susceptibility mapping. Int J Dig Earth 5:338–355
Tax D, Duin E (1999) Support vector domain description. Pattern Recogn Lett 20:1191–1199
Trigila A, Iadanza C, Esposito C, Scarascia-Mugnozza G (2015) Comparison of Logistic Regression and Random Forests techniques for shallow landslide susceptibility assessment in Giampilieri (NE Sicily, Italy). Geomorphology 249:119–136
Tsangaratos P, Ilia I (2016) Comparison of a logistic regression and Naïve Bayes classifier in landslide susceptibility assessments: the influence of models complexity and training dataset size. CATENA 145:164–179
Turker M, Koc-San D (2010) Building detection from pan-sharpened IKONOS imagery through support vector machines classification. Int Arch Photogramm Remote Sens Spat Inf Sci XXXVIII(Part 8):841–846
Turker M, Koc-San D (2015) Building extraction from high-resolution optical spaceborne images using the integration of support vector machine (SVM) classification, Hough transformation and perceptual grouping. Int J Appl Earth Obs Geoinf 34:58–69
Van der Linden S, Rabe A, Wirth F, Suess S, Okujeni A, Hostert P (2010) imageSVM regression, application manual: imageSVM Version 2.1. Humboldt Universität zu Berlin, Berlin
Van der Meer FD, Van der Werff HMA, Van Ruitenbeek FJA, Hecker CA, Bakker WH, Noomen MF, Van der Meijde M, Carranza EJM, Smeth JB, Woldai T (2012) Multi- and hyperspectral geologic remote sensing: a review. Int J Appl Earth Obs Geoinf 14:112–128
Vapnik V (1995) Nature of statistical learning theory. Wiley, New York
Varnes D (1978) Landslides types and processes, landslides and engineering practice. In: Eckel E (ed) Highway research board spec report. National Research Council, Washington, pp 20–47
Wan S (2009) A spatial decision support system for extracting the core factors and thresholds for landslide susceptibility map. Eng Geol 108:237–251
Waske B, van der Linden S, Oldenburg O, Jakimow B, Rabe A, Hostert P (2012) ImageRF—a user-oriented implementation for remote sensing image analysis with random forests. Environ Model Softw 35:192–193
Wilson J, Gallant J (2000) Terrain analysis principles and applications. Wiley, Canada
Yamaguchi Y, Fujisada H, Kudoh M, Kawakami T, Tsu H, Kahle A, Pniel M (1999) ASTER Instrument characterization and operation scenario. Adv Space Res 23:1415–1424
Yao X, Tham LG, Dai FC (2008) Landslide susceptibility mapping based on support vector machine: a case study on natural slopes of Hong Kong, China. Geomorphology 101:572–582
Yeon YK, Han JG, Ryu KH (2010) Landslide susceptibility mapping in Injae, Korea, using a decision tree. Eng Geol 116:274–283
Yesilnacar E, Topal T (2005) Landslide susceptibility mapping: a comparison of logistic regression and neural networks methods in a medium scale study, Hendek region (Turkey). Eng Geol 79:251–266
Yilmaz I (2009) Landslide susceptibility mapping using frequency ratio, logistic regression, artificial neural networks and their comparison: a case study from Kat landslides (Tokat, Turkey). Comput Geosci 35:1125–1138
Acknowledgements
This study is part of a scientific research project supported by the Scientific Research Projects Coordination Unit of Akdeniz University (Project No. FYL-2016-1732). The authors would like to thank Assoc. Prof. Dr. Hakan A. Nefeslioglu (Akdeniz University) and Murat GULER (Turkish State Meteorological Service, Antalya Regional Directorate) for their valuable comments. Finally the authors would like to thank three anonymous reviewers for their valuable comments and suggestions to improve the quality of the paper.
Author information
Authors and Affiliations
Corresponding author
Rights and permissions
About this article
Cite this article
Ada, M., San, B.T. Comparison of machine-learning techniques for landslide susceptibility mapping using two-level random sampling (2LRS) in Alakir catchment area, Antalya, Turkey. Nat Hazards 90, 237–263 (2018). https://doi.org/10.1007/s11069-017-3043-8
Received:
Accepted:
Published:
Issue Date:
DOI: https://doi.org/10.1007/s11069-017-3043-8