Abstract
Managing nitrogen (N) fertilizer applied in agricultural fields is important for increasing crop productivity while limiting the environmental contamination caused by release of reactive N, especially for crops with high N demand (e.g., corn, Zea mays L.). However, for given soil properties, the optimum amount of N applied depends on climatic conditions. The central question to N management is then what should be the recommended N rate for given soil and climate that would minimize the release of reactive N while maintaining the crop productivity. To address this central challenge of N management, we used a recently developed model-based methodology (called “Identifying NEMO”), which was proved to be effective in identifying ecophysiological optimum N rate and optimum nitrogen use efficiency (NUEopt). We performed modeling for dominant soils and various agroclimatic conditions in five regions along the Mixedwood Plains ecozone, where more than 90% of Canadian corn production takes place. Here, we analyzed for the first time the effect of soil and climate on ecophysiological optimum N rate in an ecozone where there exists a significant agroclimatic gradient. Our results indicated that there were some commonalities among all soils and regions, which we could classify them into two groups with NUEopt ranging from 10 to 17 kg dry yield kg−1 N. For cases with low NUEopt, the recommended N for an expected dry yield of 8 t ha−1 varied from 115 to 199 kg ha−1, whereas they were much lower (79–154 kg ha−1) for cases with high NUEopt. These recommendations were 20–40 kg ha−1 lower than provincial recommendations. Moreover, we found that the different behavior of the two groups was due to soil textures and soils available water holding capacity. For most locations, soils with intermediate available water holding capacity (i.e., 12–15%v) had relatively higher expected yield and lower recommended N.
Similar content being viewed by others
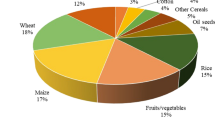
Avoid common mistakes on your manuscript.
1 Introduction
The use of N fertilizer in agricultural systems has been rapidly increasing in the last few decades to supply globally the increasing food demand (Foley et al. 2011; Zhang et al. 2015; Sela et al. 2016). The amount of N not taken up by plants may contribute to off-site deposition and contamination of other ecosystems. It creates a sequence of effects referred to as “nitrogen cascade.” These effects could be local such as off-site ammonia deposition, regional such as particulate matter formation and their long range transportation, or global such as an increase in N2O greenhouse gas emissions (Galloway et al. 2003; Erisman et al. 2007). The effects could be partly mitigated by application of optimum N rate on cultivated crops. However, the identification of optimum N rate is challenging as it varies by climatic conditions and soil properties. In a region with given soil properties, the climate is the dominant factor for deriving optimum N rate especially for high N demand crops such as corn (Zea mays L.) (Hyytiäinen et al. 2011; Tremblay et al. 2012; N’Dayegamiye et al. 2015).
Corn is the most produced cereal crop worldwide with 885.3 million t produced in 2011 (FAOSTAT 2011). In Canada, corn is the third largest grain crop produced after wheat and canola, and the most important crop in Eastern Canada, particularly in the Mixedwood Plains ecozone where more than 90% of corn production takes place (Statistics Canada 2015). Rainfed corn requires high N fertilization and thus identifying optimum N fertilizer rate is highly important to optimize corn productivity and limit N loss. However, the optimum amount of N fertilizer is highly variable as it is influenced by the interaction between soil and climate conditions. Seasonal temperature, precipitation, and soil texture are keys to availability of water which directly affects plant growth, N uptake, and optimum N. Moreover, climate conditions can affect optimum N through various processes in soil such as nitrification, denitrification, leaching, and mineralization, which modulate N availability to the crops. (Melkonian et al. 2007; van Es et al. 2007; Zhu et al. 2009; Tremblay et al. 2012; Moebius-Clune et al. 2013). Nevertheless, these effects have been neglected in many N management decisions (Raun et al. 2005; Melkonian et al. 2007; Shanahan et al. 2008). Tremblay et al. (2012) performed a meta-analysis combining studies on 51 corn production fields in North America and found that early-season precipitation is the most dominant factor affecting corn response to N rates.
A common practice to identify a recommended N rate is to perform an analysis of variance on data collected from factorial plot experiments under which yield response is often measured to large incremental N, e.g., 50 kg N ha −1. This analysis determines an N rate beyond which there is no more statistically meaningful increase in yield and yield is maximized. This approach does not account for the costs associated with N fertilizer and thus does not recommend an economically optimized N rate. The economic optimum N rate is defined as an N application rate, at which the net return of N is maximized and the ratio of unit cost of N application to the price of yield is equal to the ratio of change in yield to change in N rate (Mamo et al. 2003). The ratio is highly important in determination of optimum rate, and is referred to as nitrogen use efficiency (NUE) or incremental physiological efficiency (Bock et al. 1984; Doberman 2005). The economic approach to optimize N rate is based on external economic factors only and thus is not optimized to reduce any adverse environmental impact. The magnitudes of these adverse impacts are highly dependent on climatic conditions, and thus it is important to study such impacts over a long time period to identify an environmentally friendly optimum N rate. While experimentations over a long time period is highly costly, modeling is envisioned as an effective tool to address the impact of climate variations on identifying N recommended rates.
Crop models have been used within multi-criteria approaches under which a combination of net economic return and environmental impacts is optimized (Dumont et al. 2016; Basso et al. 2011). However, these approaches often consider N leaching as the only adverse environmental impact of N application. To account for a global estimate of reactive N losses, Mesbah et al. (2017) proposed a methodology to identify an optimum N rate (Nopt) based on crop ecophysiology, called Identifying NEMO (N Ecophysiologically Modelled Optimum). At this ecophysiological optimum N rate, there is no N excess with little reduction in yield compared to maximum achievable yield. This approach uses a process-based crop model, the Mitscherlich-Baule Plateau (MB-P) yield function, and long time series of climate data, and provides selection criteria to identify optimum NUE (NUEopt), and corresponding climate-dependent Nopt values. The MB-P function was selected as it outperformed two commonly used yield functions (the linear-plateau and MB) in mimicking modeled yield response to N rate. The performance evaluation was shown via a case study for 48 to 61 years of daily climate data and soils with contrasting properties in five regions along the Mixedwood Plains ecozone in Canada (42.3o N 83o W–46.8o N 71o W) with significant agroclimatic gradient. The method was then examined for one soil only (i.e., sandy loam), as an example to better elaborate the steps of Identifying NEMO. While the example elaborated how the method could be implemented, it did not provide any insight on how N recommendations vary by the existing agroclimatic gradient in Mixedwood Plain ecozone and by dominant soils in each region. This is addressed in the current study, which aims (1) to further investigate how N recommendations vary by soil and climate along the Mixedwood Plains ecozone with significant agroclimatic gradient, (2) to investigate whether there is any commonality in N recommendation results for different soils and regions, and (3) to identify the most fertile conditions for cultivating rainfed corn in the predominant Canadian corn production ecozone. The results could be used for further environmental assessment of reducing reactive N caused by the adoption of recommended N based on Identifying NEMO.
2 Material and methods
2.1 Proposed steps to identify recommended N rate using Identifying NEMO
In this study, a slope-based method proposed by Mesbah et al. (2017) is used to identify NUEopt, which is then used to determine climate-dependent Nopt values, i.e., one Nopt per growing season. This NUEopt is identified by evaluating the linearity of the relationship between dry yield and Nopt, and the reduction in yield compared to maximum achievable yield for a given soil and various growing seasons. Note that the dry yield can be converted to yield at 15.5% moisture (Y15.5%) when multiplied by 1.15. In this paper, the results are based on dry yield at 0% grain moisture (referred to yield from now on for simplicity purpose). The steps taken to determine the recommended N rate are:
-
1.
Select a process-based crop model adapted for the region of interest to simulate the response of dry yield to N rate with a good precision.
-
2.
Simulate the yield response to various N application rates using increments of 10 kg N ha−1 for a long time series of climatic data (e.g., 40 to 60 years).
-
3.
Individually fit an MB-P function (Eq. 1) to the year-specific data of N rates and corresponding dry yields predicted by the crop model.
$$ \mathrm{MB}-P:Y=\left\{\begin{array}{c}{Y}_{\mathrm{max}}\left(1-{\mathrm{e}}^{-{\mathrm{b}}_{\mathrm{N}}\left(N+{N}_{\mathrm{in}}\right)}\right)\kern3em N<{N}_{\mathrm{P}}\\ {}{Y}_{\mathrm{max}}\left(1-{\mathrm{e}}^{-{\mathrm{b}}_{\mathrm{N}}\left({N}_{\mathrm{P}}+{N}_{\mathrm{in}}\right)}\right)\kern2em N>{N}_{\mathrm{P}}\end{array}\right. $$(1)where Y represents the dry yield in t ha−1; Ymax is a coefficient representing the maximum achievable yield under the MB-P function if there was no constraint on maximum yield imposed by the plateau function; bN is a coefficient directly affecting the slope of MB-P function; NP is the minimum N rate value (kg N ha−1) resulting in maximum yield achieved at plateau; Nin is the initial amount of nitrogen in the soil (kg N ha−1).
-
4.
Select an NUE from a predefined NUE range (e.g., 10–20 kg yield kg−1 N) and extract YNopt–Nopt data using the fitted functions in step 3. For each NUE (slope of yield function), the number of Nopt values are equal to the number of climatic years. Repeat the process to generate YNopt–Nopt datasets for all NUE values in the predefined range.
-
a.
Perform a linear regression using NUE-specific YNopt–Nopt data and report R2 and RMSE values, which are used as criteria for selection of NUEopt.
-
b.
Calculate YNopt/Ymax and Ymax–YNopt values for each growing season. Then, calculate the mean values of the two criteria for high and low yield years (e.g., Ymax < 8 t dry yield ha−1) separately, which are also criteria for selection of NUEopt.
-
c.
Repeat steps 4.a and 4.b for all NUEs from the predefined range. Note that the YNopt–Nopt data are for a given soil and region, or for one soil merged over multiple regions only if they have similar agroclimatic conditions.
-
a.
-
5.
Construct a tradeoff plot showing the change in mean values of YNopt/Ymax and Ymax–YNopt as well as R2 and RMSE of linear fit with change in NUE. This tradeoff plot is used to identify NUEopt which leads to a fairly good R2 and RMSE and little reduction in yield compared to Ymax.
-
6.
Select an expected yield, and use the derived linear function fitted to YNopt–Nopt for the NUEopt, and calculate an N application rate, which we refer to recommended N for the expected yield. Furthermore, calculate the probability of achieving the expected yield using the time series of predicted yields.
2.2 Climate variation in the Mixedwood Plains ecozone
The proposed methodology was implemented in five regions along the Mixedwood Plains ecozone, which extends over 4.5o of latitude and 12o of longitude (Fig. 1). This ecozone is the major corn production ecozone in Canada and extends from southern Ontario to southwestern Quebec (42.3o N 83o W–46.8o N 71o W). The growing season starts in early May/June and ends in October. Crop heat units (CHU) in the growing season are often used for rating corn cultivars (Brown and Bootsma 1993). Cumulative CHU is calculated by summation of daily values from May 1st to the date when temperature drops below − 2 °C for the first time. Calculation of this value for 48 to 68 years in the ecozone shows a large variation from 2200 to 4350. The CHU is in the range of 3330–4350 for Windsor, 2750–3690 for London, 2510–3720 for Ottawa, 2640–3580 for Saint-Hubert, and 2180–3230 for Quebec. This variation in CHU requires the use of multiple cultivars to accurately predict biomass, leaf area index, and yield across the ecozone. The lowest CHU belongs to Quebec (2200 to 3200), which is located at the margin of the ecozone where CHU is a major limitation on corn growth. Cumulative precipitation is relatively high in the ecozone, which allows for rainfed cropping. Nonetheless, uneven distribution of precipitation and its inter-annual variability might lead to days with water stress during some growing seasons. The cumulative precipitation from May to August is in the range of 110–450 mm for Windsor, 106–409 mm for London, 143–445 mm for Ottawa, 160–459 mm for Saint-Hubert, and 149–496 mm for Quebec. Regions in eastern part of the ecozone (Quebec and Saint-Hubert) tend to have more frequent water excess, whereas regions in the middle and western part of the ecozone (Ottawa, Windsor, and London) tend to have more episodes of water deficit. The variations in CHU and cumulative precipitation highlight the importance of using of a long time series of climate data to evaluate the performance of crop models in simulating crop growth in response to climate variation.
Cultivated corn lands in the predominant corn production ecozone of Canada are shown in dotted green. The cultivated lands are extracted from the Annual Space-Based Crop Inventory for Canada, 2013, Centre for Agroclimate, Geomatics and Earth Observation, Science and Technology Branch, Agriculture and Agri-Food Canada
2.3 Soil selection for simulations along the Mixedwood Plains ecozone
For each region along the ecozone identified in Fig. 1, a 40 × 40-km area was selected to overlay soil data with corn cultivated lands extracted from the 2013 space-based crop inventory (Fig. 1), and identify the most dominant soil textures. The properties of three contrasting soil types in each region are presented in Table 1.
The dominant soils were identified in two steps. First, the soils were identified within soil polygons using site-specific data and conventional soil survey data (e.g., Canadian Soil Information Service; CanSIS). There existed more than one soil texture within each soil polygon, and the soil textures were identified based on the highest (acreage) percentage of the soil(s) mapped within a polygon. Second, the soil with highest areas covered by corn fields (based on 2013 data) within the selected 40 × 40-km area was selected as the most dominant soil in each region. The percentage of areas covered with corn fields varied from 8 to 63% in the 5 regions (Table 1). The Saxton pedotransfer function was used to calculate the soil moistures at field capacity and wilting point as well as their difference representing the available water capacity (AWC) (Saxton et al. 1986). These soil textures cover a wide range from 7 to 56% in clay content and 4 to 68% in sand content, as well as a relatively wide range of AWC from 10 to 17%. Three dominant soils with contrasting textures were selected from each region and used as inputs for simulations. In total, there were 819 soil-region-years for simulation, which was performed using the STICS soil-crop model (Brisson et al. 2003; Brisson et al. 2008). The STICS model was previously adapted for corn cultivars properties for the Mixedwood Pains ecozone (Jégo et al. 2011), and proven to be effective in simulating yield response to N rate. For N fertilization rates ranging from 118 to 188 kg N ha−1, the model performed well in predicting shoot biomass and dry yield with normalized root mean square errors (NRMSE) of 17.5 and 10.1% for biomass and yield respectively. The NRMSE was defined as: \( \frac{100}{\overline{\mathrm{O}}}\sqrt{1/n{\sum}_{i=1}^n{\left({\mathrm{O}}_i-{\mathrm{P}}_i\right)}^2} \), where n is the total number of observations, Oi is the observed value, Pi is the simulated value, and \( \overline{O} \) is the mean observed value. Further details on modeling inputs can be found in Mesbah et al. (2017).
3 Results and discussion
3.1 NUE selection for contrasting soils in Mixedwood Plains ecozone
To help identifying NUEopt, which is used for the determination of climate-dependent Nopt, the region and soil-specific tradeoff plots can be used to represent how NUE values affect different criteria. These criteria represent (1) how environmentally friendly the selected NUE is; which is presented by high R2 (low RMSE) of a linear fit to YNopt–Nopt data, and (2) how much reduction in predicted yield occurs with the selected NUE. The reduction in yield can be presented in percentage (YNopt/Ymax) or absolute value (Ymax–YNopt). The RMSE curves were almost the mirror image of R2 curves (Fig. 2), and R2 curves reached a plateau when RMSE reached minimum values. While R2 increases with a decreasing rate as NUE increases, there exist some differences in R2 curves for different soils. The shape of R2 curves falls into two groups based on the NUE plateau (NUEP), which is the lowest NUE at which there is no significant increase in R2. These groups are (1) regions and soils for which NUEP is smaller than 12 kg yield kg−1 N, which we refer to as low NUEP group, and (2) regions and soils for which plateaus of R2 curves occur at an NUE greater or equal to 12 kg yield kg−1 N, which we refer to as high NUEP group.
The R2 (left panels) and RMSE (right panels) of the linear fit between optimum N and dry yield at optimum N as a function of nitrogen use efficiency (NUE) for cases reaching plateau at low NUE values based on R2 criterion (a), and for cases reaching plateau at high NUE values (b). Each line represents one soil and region-specific information
The minimum values of RMSE occur at smaller NUEs for the low NUEP group in comparison to those for the high NUEP group. The low NUEP group consists of all silty clay, silty clay loam, and clay, whereas the high NUEP group includes most sandy loam and loam soils with the exception of Quebec. Clay loam is the soil texture that belongs to the first group in Ottawa, and second group in Saint-Hubert. The soil texture was a dominant factor in the determination of NUEP, which is supported by the study of Tremblay et al. (2012). While loam is common to all cases in high NUEP, clay is common to all cases in low NUEP group (excluding Quebec). Moreover, the soils belonging to each NUEP group have similar AWC. All soils with an AWC of less than 12%v belong to the high NUEP group, while the soils with an AWC higher than 15%v belong to the low NUEP group. These findings indicate that AWC and soil textures play key roles in identifying optimum N rate and N recommendations.
The results presented in Fig. 2 indicate that the selection criteria for NUE were affected by both soil texture and climate. For example, for the same climatic time series in London, R2 of the linear fit between YNopt and Nopt reached plateau at values between 12 and 18 kg yield kg−1 N with lower NUEP value for silty clay loam, and higher NUEP values for loam and sandy loam. Another example highlighting the effect of soil is Windsor where the silty clay and silty clay loam soils led to low NUEP of 11 kg yield kg−1 N, while sandy loam resulted in a higher NUEP of about 16 kg yield kg−1 N.
On the other hand, NUEP, which directly affects the NUEopt and climate-dependent Nopt values, varies by regions with different climatic conditions even for the same soil texture. This finding reveals that one must not merge soil data over multiple regions for N recommendation as in doing so some information will be lost. For instance, the example provided in Mesbah et al. (2017) merged data for the sandy loam soil (excluding Quebec), and provided identical N recommendations for all five regions along the ecozone. This in turn led to an NUEP of 16 kg yield kg−1 N, which is different than NUEP value provided in this study obtained by analyzing region-specific data for sandy loam soil, i.e., NUEP ranging from 14 to 16 kg yield kg−1 N with the exception of Quebec. The shape of R2 curves for London and Windsor was very similar leading to an NUEP of about 15 and 16 kg yield kg−1 N, respectively. For the sandy loam soil in Saint-Hubert, NUEP was also about 15 kg yield kg−1 N, while the R2 curve was very different and sharply rose with an increase in NUE. On the other hand, R2 curve for the sandy loam soil in Ottawa increased with a smaller rate and reached plateau at a smaller NUE of 14 kg yield kg−1 N. In Quebec, NUEP occurred at a small NUE of 10 kg yield kg−1 N. This is because of the CHU limitation on growth in this region.
An economic optimum NUE represents the ratio of N price to crop price, which was estimated as 10 kg yield kg−1 N for corn production in eastern Canada (Nyiraneza et al. 2010). While for the low NUEP group, the selection of an economic NUE of 10 kg yield kg−1 N seems a reasonable choice, for the high NUEP group, the selection of an NUE could be eased with additional yield loss criteria, i.e., percentage reduction in yield (YNopt/Ymax) and absolute yield reduction (Ymax–YNopt), the latter is presented in Fig. 3. In years with yield higher than 8 t ha−1 (Y15.5%: 9.2 t ha−1), the yield loss was highest for sandy loam soils in Windsor ranging from 0.2 to 1.2 t ha−1 on average, and lowest for sandy loam soil in Saint-Hubert ranging from 0 to 0.7 t ha−1. This might be due to the larger and more frequent rainfall deficit observed in Ontario. In high yield years, the yield losses showed a nonlinear response to change in NUE. The loss was small at low NUE and sharply increased at higher values. However, the value of losses at highest NUE in high yield years were still smaller than those in low yield years at which yield losses showed a linear response to change in NUE.
Average difference in maximum dry yield (Ymax) and dry yield at Nopt (YNopt) for various soils along the Mixedwood Plains ecozone calculated for low yield years (left panels) and high yield years (right panels) for the high NUEP group. Each line represents one soil and region specific information. The highlighted point on each line corresponds to the optimum nitrogen use efficiency
The higher NUE values led to more environmentally friendly choices, which in turn resulted in more reduction in yield. The selection of NUE depends on the prioritized aspects of growing crops, either on maximizing the economic return or on minimizing the impact on the environment. The NUEopt, highlighted by points in Fig. 3, were selected equal to NUEP (based on the R2 criterion) values if the reduction in yield did not exceed 0.5 t ha−1, or 5% of maximum achievable yield in high yield years. This threshold on maximum allowable yield loss was also checked in low yield years at regions where the chance of achieving a yield of 8 t ha−1 was low (i.e., less than 40%). If the reduction in yield exceeded the threshold, an NUE corresponding to the maximum allowable yield loss was selected as NUEopt. For example, for the loam soil texture in London, the R2 curve reached plateau at an NUE of about 18 kg yield kg−1 N and corresponding R2 of 0.84 (Fig. 2). However, at this NUE, the dry yield loss for high yield was 0.6 t ha−1 and exceeded the threshold. Therefore, an NUE of 16 kg yield kg−1 N was selected as NUEopt. At this NUE, the R2 is 0.81, which is slightly smaller than the R2 at plateau.
3.2 Recommended N, N excess, and deficit
The selected NUEopt could be used to identify a recommended N ( Nrec) rate for each soil using the following equation derived from the linear function fitted to YNopt–Nopt data:
where Yexp is the expected yield, and aNUE and bNUE are the intercept and slope of linear fit to the data, respectively.
For a given expected yield, the average amount N excess/deficit and reduction in yield if Nrec is applied can be calculated using Eqs. (3) and (4), respectively. Note that for a given NUE, there exist as many Nopt values as the number of climate years, i.e., one Nopt per growing season.
where \( \overline{\Delta \mathrm{Y}} \) is the average reduction in yield if Nrec is applied, and Ymax, i is the maximum achievable yield for climatic year i, \( \overline{\Delta \mathrm{N}} \) is the average value of N deficit if negative and N excess if positive. For each region, two \( \overline{\Delta \mathrm{N}} \) values are calculated: (1) for years for which yield is less than expected yield at Nopt (Yi(Nopt) < Yexp), and (2) for years (i) for which yield is more than Yexp. The Nrec, \( \overline{\Delta \mathrm{N}} \), and \( \overline{\Delta \mathrm{Y}} \) for the contrasting soils in the Mixedwood Plains ecozone are summarized for low and high NUEP groups in Table 2.
The N rate generating minimum N excess or deficit varies by region’s climatic condition and soil texture. Such N rates correspond to expected yields slightly lower than maximum achievable yields. This information along with the chance of achieving a yield for a region are keys for a risked informed decision making on the amount of recommended N. The chance of achieving higher yield is higher in London and Windsor compared to other regions. For example, if 139 kg N ha−1 is applied in London for silty clay loam soil, there is 60% chance to reach a yield of 9 t ha−1 (Y15.5%: 10.35 t ha−1) with little N excess of 1 kg N ha−1 on average in years with yields greater than expected yield and an average yield loss of 0.4 t ha−1. In this case, N excess in years with yields smaller than expected yield is relatively large (i.e., 50 kg N ha−1) on average. The effect of soil texture is also pronounced in this region. For example, for the silty clay loam soil in Windsor, the chance of reaching a yield of 10 t ha−1 (if 182 kg N ha−1 applied) is lower (22%), and this expected yield is associated with smaller N excess (2 kg ha−1) and reduction in yield (0.07 t ha−1) on average. Comparing these values with those in London and for the same soil (i.e., silty clay loam soil) highlights the impact of climatic conditions. The yield decreases by moving towards northeast within the Mixedwood Plains ecozone. The expected yield corresponding to no N excess was lower for Ottawa with clay loam soil and Saint-Hubert with clay soil. For these cases, the chance of achieving a dry yield higher than 8 t ha−1 (Y15.5%: 9.2 t ha−1) was 27% in Saint-Hubert, and 41% in Ottawa leading to an N deficit of 4 and 3 kg N ha−1, and a yield loss of 0.10 and 0.19 t ha−1, respectively. The expected yield in Quebec was least compared to other regions. In this region, no N deficit occurred for an expected yield between 5 and 6 t ha−1 for sandy loam and loam soils, and between 6 and 7 t ha−1 for silty clay soil. To achieve such low yield in this region, relatively high amount of N was required. For example, 163 kg N ha−1 was required to reach a yield of 6 t ha−1 in Quebec, which confirms that this is a marginal region for grain corn production.
While the NUEopt for all cases in low NUEP group was selected as 10 kg dry yield kg−1 N, the selection of NUEopt for high NUEP group was based on an additional constraint limiting the reduction in yield to 0.5 t ha−1 or 5% of maximum achievable yield. Out of 7 cases in this group, 3 cases were evaluated by setting a yield loss threshold on low yield years (i.e., Ottawa-sandy loam, Saint-Hubert-clay loam, and Saint-Hubert-sandy loam) as they had low chance of achieving a yield of 8 t ha−1. For London-sandy loam, the average yield loss in high yield years at NUEP was below the threshold and thus NUEP was selected as NUEopt (Fig. 3). For the other cases, the NUEopt was slightly reduced from NUEP to meet the second criteria. For these cases, an expected yield of 9 t ha−1 led to least N deficit/excess in Windsor and London, while a lower expected yield of 7 t ha−1 led to least N excess/deficit in Ottawa (Table 2). In Saint-Hubert, while the least N excess/deficit belonged to an expected yield of 9 t ha−1 for clay loam, and 7.5 t ha−1 for sandy loam soil, the amount of recommended N for both soil was similar (e.g., about 140 kg N ha−1). The recommended N values for loam soils were significantly smaller as compared to sandy loam soils. For instance, a yield of 9 t ha−1 could be achieved in London by applying a recommended N of 138 kg N ha−1 in sandy loam soil, or by a significantly lower N rate of 95 kg N ha−1 in loam soil. In London, while the chance of achieving a yield of 9 t ha−1 yield in loam was 40%, which is higher than that in sandy loam soil (29%), the fact that sandy loam soil needed about 60 kg N ha−1 more N fertilizer for the same yield suggested that loam soil might be a more environmentally friendly option for corn cultivation. This is due to the fact that loam is a soil with intermediate AWC (i.e., between 12 and 15%v). Our results show that for all locations, except Quebec, soils with intermediate AWC are better for corn cultivation because in these soils the chance of achieving a given yield is highest while the N recommendations are lowest compared to soils with high or low AWC (i.e., AWC higher than 15%v or lower than 12%v). For example in Saint-Hubert, the likelihood of achieving an expected yield of 8 t ha−1 is 52% for clay loam soil (intermediate AWC), whereas this likelihood is lower in clay soil (27%) with high AWC, and sandy loam soil (17%) with low AWC. Moreover, the recommendation is 121 kg N ha−1 for clay loam soil (intermediate AWC), whereas the recommendations are higher for high and low AWC soils, i.e., 199 kg N ha−1 in clay soil and 152 kg N ha−1 sandy clay soil.
We state that the results presented in this paper are proof-of-concept based on a process-based model accounting for major mechanisms of crop growth and ecophysiology. The STICS model which was used for derivation of recommended N by soil and region was adapted and tested extensively in Eastern Canada. The range of predicted yield by the STICS model in various regions is in agreement with county-level measured yield in Ontario and Quebec (CRAAQ, 2010; OMAFRA, 2010). The results also show the good performance of the proposed model-based methodology to identify optimum N rate, which is in part due to the capability of crop models in simulating yield response to small increment of N with high accuracy. Future research should design factorial experiments with small incremental N application rates, and verify the model-based N recommendations. The next step would be to predict the potential reduction of nitrous oxide and ammonia emissions, and nitrate leaching brought by adopting those N recommendations, using verified process-based models. It should be noted that the proposed method did not account for split N application; however, our simulations (not shown) indicated that split application had minor effect on the results presented in this paper. It is worth mentioning that N recommendations presented in this paper are different than tactical approaches such as Adapt-N (Moebius-Clune et al. 2013; Sela et al. 2016) under which N is recommended within season, based on the time and location of N deficit occurrence. Identifying NEMO is rather a strategic approach, which looks at the historical variations in daily climatic data; predicts the probability of achieving any expected yield; and provides general guidelines for N recommendations for the region of interest, given soil and expected yield. It should be noted that these strategic N recommendations are for the beginning of the season when the upcoming weather condition is uncertain. While this uncertainty could make the decision-making process very challenging, our approach could partly reduce such uncertainty by looking at the general trends in weather conditions for a given region. The proposed methodology could be implemented in other regions and for other rainfed crops provided that a crop model is adapted and could predict the yield response to N with good accuracy.
4 Conclusion
The ecophysiological optimum N rates vary by soil texture and along the agroclimatic gradient. The inter-annual climate variations dominate the amount of N application rates needed by the crop for each growing season. An important question is then at what N rate the ecophysiological optima occur. Because the growing season weather is not known for a given season, a recently developed model-based methodology was used for an in-depth analysis on the impact of soils and climate on optimum N rate along the Mixedwood Plains ecozone of Eastern Canada where there exists a gradient of agroclimatic conditions.
Application of Identifying NEMO in predominant Canadian corn production ecozone revealed some new insights on sustainable N recommendations. It was found that N recommendation must be region and soil specific as soil and climate have interactive impacts on the optimum N rate. Our results indicated that the NUEopt was often higher for soils containing loam, and lower for soil containing clay. Another important factor was the AWC of soils. NUEopt was higher for soils with AWC of less than 12%v, and lower for soils with AWC higher than 15%v. The predictions also indicated that N rate leading to minimum N excess or deficit vary by region and soil, which highlights that the expected yield must be selected based on region and soil. The soils showing intermediate AWC needed the lowest recommended N while having high expected yields. This study is a first step using model-based N rate recommendations accounting for climate variations, and the conclusions need to be further verified with experimental studies to confirm the trends.
References
Basso B, Ritchie JT, Cammarano D, Sartori L (2011) A strategic and tactical management approach to select optimal N fertilizer rates for wheat in a spatially variable field. Eur J Agron 35(4):215–222. https://doi.org/10.1016/j.eja.2011.06.004
Bock BR (1984) Efficient use of nitrogen in cropping systems. In: Hauck RD (ed), Nitrogen in crop production. Am Soc Agron, Madison WI, pp 273–294
Brisson N, Gary C, Justes E, Roche R (2003) An overview of the crop model STICS. Eur J Agron 18:309–332. https://doi.org/10.1016/S1161-0301(02)00110-7
Brisson N, Launay M, Mary B, Beaudoin N (2008) Conceptual basis, formalisations and parameterization of the STICS crop model. Editions Quae, Versailles
Brown D, Bootsma A (1993) Crop heat units for corn and other warm season crops in Ontario. Publ. 111/31, Ontario Ministry of Agriculture, Food and Rural Affairs, Ottawa ON
CRAAQ (2010) Guide de référence en fertilisation. 2nd ed. (In French.) Centre de référence en Agriculture et Agroalimentaire du Québec, Québec QC
Dobermann A (2005) Nitrogen use efficiency—state of the art. IFA international workshop on enhanced-efficiency fertilizers, Frankfurt Germany
Dumont B, Basso B, Bodson B, Destain JP, Destain MF (2016) Assessing and modeling economic and environmental impact of wheat nitrogen management in Belgium. Environ Model Softw 79:184–196. https://doi.org/10.1016/j.envsoft.2016.02.015
Erisman JW, Bleeker A, Galloway J, Sutton MS (2007) Reduced nitrogen in ecology and the environment. Environ Pollut 150:140–149. https://doi.org/10.1016/j.envpol.2007.06.033
Van Es HM, Kay BD, Melkonian JJ, Sogbedji JM (2007) Nitrogen management for maize in humid regions: Case for a dynamic modeling approach. In: Bruulsema TW (ed) Managing Crop Nitrogen for Weather: Proceedings of the Symposium “Integrating Weather Variability into Nitrogen Recommendations,.” Int. Plant Nutr. Inst., Norcross, GA., N. 15 Nov. 2006, pp 6–13
FAOSTAT (2011) Food and Agriculture Organization of the United Nations
Foley JA, Ramankutty N, Brauman KA, Cassidy ES, Gerber JS, Johnston M et al (2011) Solutions for a cultivated planet. Nature 478:337–342. https://doi.org/10.1038/nature10452
Galloway JN, Aber JD, Erisman JW, Seitzinger SP, Howarth RW, Cowling EB, Cosby BJ (2003) The nitrogen cascade. Bioscience 53:341–356. https://doi.org/10.1017/CBO9781107415324.004
Hyytiäinen K, Niemi JK, Koikkalainen K, Palosuo T, Salo T (2011) Adaptive optimization of crop production and nitrogen leaching abatement under yield uncertainty. Agric Syst 104:634–644. https://doi.org/10.1016/j.agsy.2011.06.006
Jégo G, Pattey E, Bourgeois G, Drury CF, Tremblay N (2011) Evaluation of the STICS crop growth model with maize cultivar parameters calibrated for Eastern Canada. Agron Sustain Dev 31:557–570. https://doi.org/10.1007/s13593-011-0014-4
Mamo M, Malzer GL, Mulla DJ, Huggins DR, Strock J (2003) Spatial and temporal variation in economically optimum nitrogen rate for corn. Agron J 95:958–964. https://doi.org/10.2134/agronj2003.9580
Melkonian J, van Es HM , DeGaetano AT, Sogbedji JM, Joseph L, Bruulsema T (2007) Application of dynamic simulation modeling for nitrogen management in maize. In: Bruulsema TW (ed) Managing crop nitrogen for weather: proceedings of the symposium “integrating weather variability into nitrogen recommendations,.” Int. Plant Nutr. Inst., Indianapolis IN.5 Nov. 2006, pp 14–22
Mesbah M, Pattey E, Jégo G (2017) A model-based methodology to derive optimum nitrogen rates for rainfed crops—a case study for corn using STICS in Canada. Comput Electron Agric 142:572–584. https://doi.org/10.1016/j.compag.2017.11.011
Moebius-Clune BN, Van Es HM, Melkonian J (2013) Adapt-N uses models and weather data to improve nitrogen management for corn. Better Crops 97:7–9
N’Dayegamiye A, Whalen JK, Tremblay G, Nyiraneza J, Grenier M, Drapeau A, Bipfubusa M (2015) The benefits of legume crops on corn and wheat yield, nitrogen nutrition, and soil properties improvement. Agron J 107:1653–1665. https://doi.org/10.2134/agronj14.0416
Nyiraneza J, N’Dayegamiye A, Gasser MO, Giroux M, Grenier M, Landry C, Guertin S (2010) Soil and crop parameters related to corn nitrogen response in eastern Canada. Agron J 102:1478–1490. https://doi.org/10.2134/agronj2009.0458
OMAFRA (2010) Corn nitrogen rate worksheet: general recommended nitrogen rates for corn. Ontario Ministry of Agriculture, Food, and Rural Aff airs, Guelph, ON. http://www.gocorn.net/v2006/Ncalc/General%20Recommended%20Nitrogen%20Rates%20for%20Corn%202010.pdf (accessed 24 Nov. 2017)
Raun WR, Solie JB, Stone ML, Martin KL, Freeman KW, Mullen RW et al (2005) Optical sensor-based algorithm for crop nitrogen fertilization. Commun Soil Sci Plant Anal 36:2759–2781. https://doi.org/10.1080/00103620500303988
Saxton KE, Rawls WJ, Romberger JS, Papendick RI (1986) Estimating generalized soil-water characteristics from texture. Soil Sci Soc Am J 50:1031–1036. https://doi.org/10.2136/sssaj1986.03615995005000040054x
Sela S, Van Es HM, Moebius-Clune BN et al (2016) Adapt-N outperforms grower-selected nitrogen rates in Northeast and Midwest USA strip trials. Agron J 108:1726–1734. https://doi.org/10.2134/agronj2015.0606
Shanahan JF, Kitchen NR, Raun WR, Schepers JS (2008) Responsive in-season nitrogen management for cereals. 61:51–62. doi: https://doi.org/10.1016/j.compag.2007.06.006
Statistics Canada (2015) Corn: Canada’s third most valuable crop. Canadian agriculture at a glance. In: Report 96–325-X
Tremblay N, Bouroubi YM, Bélec C, Mullen RW, Kitchen NR, Thomason WE (2012) Corn response to nitrogen is influenced by soil texture and weather. Agron J 104:1658–1671. https://doi.org/10.2134/agronj2012.0184
Zhang X, Davidson EA, Mauzerall DL, Searchinger TD, Dumas P, Shen Y (2015) Managing nitrogen for sustainable development. Nature 528:51–59. https://doi.org/10.1038/nature15743
Zhu Q, Schmidt JP, Lin HS, Sripada RP (2009) Hydropedological processes and their implications for nitrogen availability to corn. Geoderma 154:111–122. https://doi.org/10.1016/j.geoderma.2009.10.004
Acknowledgements
The authors thank René Morissette for his assistance in processing of simulation results.
Funding
The study was funded by Agriculture and Agri-Food Canada Sustainable Science and Technology Advancement initiative project J-001318 - Improve N sustainability of field crops and J-000106 Development of decision-support components for optimal N fertilizer applications at the field scale.
Author information
Authors and Affiliations
Corresponding author
Ethics declarations
Conflict of interest
The authors declare that they have no conflict of interest.
About this article
Cite this article
Mesbah, M., Pattey, E., Jégo, G. et al. New model-based insights for strategic nitrogen recommendations adapted to given soil and climate. Agron. Sustain. Dev. 38, 36 (2018). https://doi.org/10.1007/s13593-018-0505-7
Accepted:
Published:
DOI: https://doi.org/10.1007/s13593-018-0505-7