Abstract
A contaminated industrial waste site in Washington State (USA) containing buried, metallic-waste storage tanks, pipes, and wells, was evaluated to determine the feasibility of monitoring groundwater remediation activities associated with an underlying perched aquifer system using electrical resistivity tomography. The perched aquifer, located ~65 m below ground surface and ~10 m above the regional water table, contains high concentrations of nitrate, uranium, and other contaminants of concern from past tank leaks and intentional releases of wastes to surface disposal sites. The extent of the perched water aquifer is not well known, and the effectiveness of groundwater extraction for contaminant removal is uncertain, so supplemental characterization and monitoring technologies are being evaluated. Numerical simulations of subsurface flow and contaminant transport were performed with a highly resolved model of the hydrogeologic system and waste site infrastructure, and these simulations were used as the physical basis for electrical resistivity tomography modeling. The modeling explicitly accounted for metallic infrastructure at the site. The effectiveness of using surface electrodes versus surface and horizontal subsurface electrodes, for imaging groundwater extraction from the perched water aquifer, was investigated. Although directional drilling is a mature technology, its use for electrode emplacement in the deep subsurface under a complex industrial waste site via horizontal wells has not yet been demonstrated. Results from this study indicate that using horizontal subsurface electrode arrays could significantly improve the ability of electrical resistivity tomography to image deep subsurface features and monitor remediation activities under complex industrial waste sites.
Résumé
Un site de déchets industriels contaminé, dans l’État de Washington (États-Unis d’Amérique), contenant des réservoirs de stockage de déchets métalliques enterrés, des tuyaux et des puits a été évalué pour déterminer la faisabilité de la surveillance des activités de dépollution des eaux souterraines, associées à un système aquifère perché sous-jacent, à l’aide de la tomographie de résistivité électrique. L’aquifère perché, situé à environ 65 m sous la surface du sol et à environ 10 m au-dessus de la nappe souterraine régionale, contient de fortes concentrations en nitrate, uranium et autres contaminants préoccupants provenant d’anciennes fuites de réservoirs et de lixiviats des sites de stockage en surface. L’étendue de l’aquifère perché n’est pas bien connue, et l’efficacité des pompages d’eau souterraine pour l’élimination des contaminants est incertaine, de sorte que des technologies de caractérisation et de surveillance supplémentaires sont en cours d’évaluation. Des simulations numériques de l’écoulement souterrain et du transport des contaminants ont été effectuées avec un modèle haute résolution du système hydrogéologique et de l’infrastructure du site de déchets, et ces simulations ont été utilisées comme base physique pour la modélisation par tomographie de résistivité électrique. La modélisation tient explicitement compte de l’infrastructure métallique du site. L’efficacité de l’utilisation d’électrodes de surface, par rapport aux électrodes de surface et horizontales de sub-surface, pour l’imagerie de l’extraction des eaux souterraines de l’aquifère d’eau perché a été étudiée. Bien que le forage directionnel soit une technologie mature, son utilisation pour la mise en place d’électrodes dans le sous-sol profond sous un site de déchets industriels complexes via des puits horizontaux n’a pas encore été démontrée. Les résultats de cette étude indiquent que l’utilisation de réseaux d’électrodes horizontales souterraines pourrait améliorer considérablement la capacité de la tomographie de résistivité électrique à imager les caractéristiques profondes de la sub-surface et à surveiller les activités de dépollution sous des sites de déchets industriels complexes.
Resumen
Se evaluó un lugar de desechos industriales contaminados en el Estado de Washington (EE.UU.) que contenía tanques de almacenamiento de residuos metálicos enterrados, tuberías y pozos para determinar la viabilidad de monitorear las actividades de remediación de las aguas subterráneas asociadas a un sistema acuífero colgado subyacente utilizando una tomografía de resistividad eléctrica. El acuífero colgado, situado a ~65 m bajo la superficie del suelo y ~10 m sobre el nivel freático regional, contiene altas concentraciones de nitrato, uranio y otros contaminantes peligrosos procedentes de fugas de tanques anteriores y de liberaciones intencionadas de residuos a los lugares de eliminación en la superficie. No se conoce bien la extensión del acuífero colgado y la eficacia de la extracción de aguas subterráneas para la eliminación de contaminantes es incierta, por lo que se están evaluando tecnologías suplementarias de caracterización y monitoreo. Se realizaron simulaciones numéricas del flujo subsuperficial y del transporte de contaminantes con un modelo de alta resolución del sistema hidrogeológico y de la infraestructura de los sitios de vertido, y esas simulaciones se utilizaron como base física para la elaboración de modelos de tomografía de resistividad eléctrica. La modelización tuvo en cuenta explícitamente la infraestructura metálica del emplazamiento. Se investigó la eficacia de utilizar electrodos de superficie frente a electrodos de superficie y de subsuelo horizontal, para obtener imágenes de la extracción de agua subterránea del acuífero colgado. Aunque la perforación direccional es una tecnología avanzada, todavía no se ha demostrado su uso para la colocación de electrodos en el subsuelo profundo bajo un complejo vertedero de desechos industriales mediante pozos horizontales. Los resultados de este estudio indican que el uso de las baterías de electrodos en el subsuelo horizontal podría mejorar significativamente la capacidad de la tomografía de resistividad eléctrica para obtener imágenes de las características del subsuelo profundo y supervisar las actividades de rehabilitación bajo los complejos vertederos de desechos industriales.
摘要
对美国华盛顿州一个受污染的工业废弃场进行了评估,该工业废弃场包含埋置的金属废料储罐、管道和水井,以确定利用电阻层析成像技术监测与下伏上层滞水层系统相关的地下水修复活动的可行性。上层滞水层位于地面以下约65 m,区域地下水位以上约10 m,含有高浓度的硝酸盐、铀和其他有关污染物,这些污染物来自过去的储罐泄漏和有意向地表处置场排放废物。上层滞水层的范围尚不清楚,地下水开采对污染物去除的有效性也尚不确定,因此对补充的场地特征和监测技术进行评估。利用水文地质系统和废物场基础设施的高分辨模型对地下水流动和污染物迁移进行了数值模拟,并将这些模拟作为电阻率层析成像建模的物理基础。模型明确说明了现场的金属基础设施。研究了利用地表电极与地表和水平地下电极对从上层滞水层抽取地下水进行成像的有效性。虽然定向钻井是一项成熟的技术,但它通过水平井在复杂工业废弃场下的深层地下安置电极的应用尚未得到证实。本研究结果表明,利用水平地下电极阵列可以显著提高电阻率层析成像技术在复杂工业废弃场下对深层地下特征进行成像和监测修复活动的能力。
Resumo
Um local contaminado por resíduos industriais no estado de Washington (EUA), contendo tanques, tubos e poços enterrados de resíduos metálicos, foi avaliado para determinar a viabilidade de monitorar a remediação das águas subterrâneas, associadas a um sistema aquífero suspenso subjacente, usando tomografia de resistividade elétrica. O aquífero suspenso, localizado a ~65 m abaixo da superfície do solo e ~10 m acima do lençol freático regional, contém altas concentrações de nitrato, urânio e outros contaminantes preocupantes de vazamentos anteriores de tanques e liberações intencionais de resíduos nos locais de descarte de superfície. A extensão do aquífero suspenso aquoso não é bem conhecida, e a eficácia da extração de águas subterrâneas para remoção de contaminantes é incerta; portanto, tecnologias complementares de caracterização e monitoramento tem sido avaliadas. Simulações numéricas de fluxo subsuperficial e transporte de contaminantes foram realizadas com um modelo altamente resolvido do sistema hidrogeológico e da infraestrutura do local de resíduos, e essas simulações foram usadas como base física para a modelagem da tomografia por resistividade elétrica. A modelagem explicou explicitamente a infraestrutura metálica no local. A eficácia do uso de eletrodos de superfície versus eletrodos de superfície e horizontais de subsuperfície, para geração de imagens de extração de águas subterrâneas do aquífero suspenso, foi investigada. Embora a perfuração direcional seja uma tecnologia mais antiga, seu uso para a colocação de eletrodos na subsuperfície profunda sob um complexo local de resíduos industriais através de poços horizontais ainda não foi demonstrado. Os resultados deste estudo indicam que o uso de fileiras horizontais de eletrodo de subsuperfície poderia melhorar significativamente a capacidade da tomografia de resistividade elétrica para gerar imagens de características profundas do subsolo e monitorar as atividades de remediação em locais contaminados por resíduos industriais.
Similar content being viewed by others
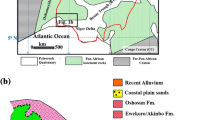
Introduction
Contamination of unsaturated sediments and underlying aquifer systems is a problem at many industrial waste sites (Oostrom et al. 2017; Kuras et al. 2016). The interactions between biophysical and geochemical processes that occur within the highly heterogeneous vadose zone are difficult to visualize, which limits our understanding of how subsurface features and processes affect contaminant transport and fate (Binley et al. 2015). The problem is often worse for sites located in arid and semi-arid regions where the unsaturated zone is relatively thick and the water table is deep (Cassiani and Binley 2005; Oostrom et al. 2013; Wellman et al. 2013). Characterization of subsurface features and properties and monitoring of the transport of fluids and contaminants at such sites are crucial for identifying the subsurface hydrogeochemical processes that affect contaminant transport and fate. However, characterization and remediation are complicated by limited accessibility to the deep and often complex subsurface environments, as well as shortcomings of available technologies (Wellman et al. 2013).
In addition to limited accessibility, subsurface hydrological studies in arid regions of much of the western United States are often complicated by features such as perched aquifers (Nimmo et al. 2004; Oostrom et al. 2013; Robinson et al. 2012). A perched aquifer is defined as a saturated subsurface region that is above the regional water table, which defines the deep saturated aquifer (Freeze and Cherry 1979). Such perched aquifers (hereafter referred to as PA), may exist as either permanent or temporary features created by a permeable layer overlying a relatively impermeable layer, or in a well-connected fractured unit overlying an unfractured or poorly connected fractured unit (Oostrom et al. 2013; Wu et al. 1999). Both vertical and horizontal flow through a PA may be affected by the adjacent low-permeability layers, which may cause increased lateral flow. Sparsity of well data may also make it difficult to delineate the extent of a PA, and to monitor remediation efforts (Carroll et al. 2009; Oostrom et al. 2017).
Hydrogeological monitoring and remediation are typically expensive, time consuming, and require the use of many wells to adequately define the extent of contaminant plumes, implement remediation strategies, and monitor remediation progress. The traditional in situ sampling methods of characterizing subsurface properties are mostly based on invasive drilling approaches to obtain small-scale measurements (Binley et al. 2015; Cassiani and Binley 2005). These traditional characterization and monitoring methods based on well drilling can be augmented at many sites using electrical resistivity tomography (ERT), which is a geophysical method for imaging the subsurface based on measurement of electrical resistivity. Raw resistivity data for ERT are determined from measured electrical potential differences between pairs of electrodes normalized by the current injected. These data are processed and inverted to produce a distribution of electrical resistivity, or its inverse, electrical conductivity.
Electrical resistivity measurements and ERT have been used in a wide variety of applications. Daily et al. (1992) describe one of the early applications of ERT in studying water flow dynamics, where hydraulic properties were determined from images of wetting fronts obtained during water infiltration. Dahlin (2001) provides a review of direct current resistivity imaging techniques and various applications, including aquifer mapping, delineation of subsurface contamination, and evaluating ground stability around construction sites. Chambers et al. (2006) describes applications of ERT to characterize site geology, evaluate the geometry of a buried quarry, and map subsurface contamination associated with a landfill in Scotland. Chambers et al. (2012) used ERT for mapping the depth to bedrock underlying fluvial sand and gravel sediments in the UK.
High-resolution images of the subsurface obtained from ERT can be used for visualizing underground features, subsurface heterogeneity, water flow processes (Binley et al. 2002, 2015), and for monitoring contaminant plume dynamics in the vadose zone when used in conjunction with flow and transport models (Cassiani and Binley 2005; Robinson et al. 2019; Johnson et al. 2017). ERT can also be used to assess the spatial and/or temporal variability of subsurface properties by translating the raw geophysical measurement into the spatial pattern of desired parameters using an inversion process (Binley and Kemna 2005). Hydrological quantities (e.g. moisture content or solute concentration) can be obtained from petro-physical relations (e.g., Archie 1942) and can be used to calibrate hydraulic parameters (Binley et al. 2002; Cassiani and Binley 2005). Recently, ERT and other geophysical tools have been used to augment the study of ecosystem science (Binley et al. 2015). Practical use of ERT has been increasing in hydrogeological applications for characterization and monitoring owing to its relative ease of implementation, four-dimensional (4D) imaging ability, larger volumes of interrogation of bulk electrical resistivity/conductivity than other measurement or sampling methods, automated data acquisition, and the availability of robust, open-source software for forward and inverse modeling (Binley 2015; Johnson 2014; Johnson et al. 2010, 2017; Kuras et al. 2016; Park et al. 2016; Robinson et al. 2019).
Although ERT has been used successfully in many applications, effective imaging of subsurface electrical resistivity at complex industrial waste sites has been hampered by the presence of metallic infrastructure such as buried tanks, pipes, and wells. Rucker and Fink (2007) used ERT with surface electrode arrays to delineate contaminant plumes in the subsurface underlying a waste disposal facility at the Hanford Site in the US. The use of surface electrodes, presence of near-surface metallic infrastructure, relatively deep target region (~40–70 m below ground surface), and choice of inversion method resulted in smoothed images with limited depth resolution. Rucker et al. (2010) subsequently evaluated the use of surface electrode arrays versus vertically oriented steel wells as long electrodes for characterizing a contaminant plume in the subsurface of another waste disposal site at Hanford. The (60–80 m) long electrodes (wells) were explicitly accounted for in resistivity modeling by assigning low resistivity values to model grid blocks representing the well locations. Modeling results indicated that when no other near-surface metallic infrastructure (e.g. tanks, pipes) was present, a surface electrode array was better able to identify the location of a low-resistivity target (e.g. contaminant plume) in the subsurface than the long-electrode array, which lacked depth resolution. However, when a thin, conductive layer was placed above the target and just below the ground surface to mimic metallic infrastructure such as a piping network, the surface electrode array was unable to resolve the target. In this case the long-electrode array was reported to find the location of the target (in the x–y plane) with reasonable accuracy.
Limited success in using ERT to image the subsurface at sites containing significant metallic infrastructure motivated the development of improved inversion algorithms. Johnson et al. (2012) developed methods for incorporating constraints into an ERT inversion algorithm, in the form of discontinuous boundaries, known values, and independently determined spatial covariance information. Johnson and Wellman (2013) reinverted the resistivity data collected by Rucker and Fink (2007) using an algorithm that explicitly accounts for metallic infrastructure, including wells, pipes, and storage tanks, and reported significantly improved imaging results using the new inversion methods. Marinenko et al. (2019) reported on numerical experiments to evaluate the potential effects of metal pipes located above and below the ground surface on the ability of ERT to identify low-resistance zones of permafrost thawing around gas production wells. The presence of metallic objects led to significant anomalies in apparent resistivities. Their inversion algorithms were reported to only partially suppress the anomalies, but their analyses allowed them to determine points that could be excluded from the model to reduce the influence of highly conductive objects. In general, advancements in inversion methods have resulted in significantly improved imaging results from ERT.
Additional improvements can potentially be achieved using other strategies such as placement of electrodes closer to target regions, and by combining ERT with other technologies. For example, Slater et al. (2010) demonstrated the combined use of ERT and fiber-optic distributed temperature sensing for characterizing areas of enhanced groundwater-surface water exchange in a section of the Columbia River adjacent to former uranium disposal ponds and trenches at the Hanford Site in the US, while Busato et al. (2014) and Cultrera et al. (2018) demonstrated the combined use of ERT and fiber-optic distributed temperature sensing at field sites in Italy. In the study by Cultrera et al. (2018), ERT and fiber-optic distributed temperature sensing was used for site characterization and to help inform a numerical flow and transport model of the field site. The study by Busato et al. (2014) used surface and subsurface electrodes to monitor along an 80-m-long transect across an alpine creek in northern Italy. The subsurface electrodes were located at a depth of up to 5 m below the ground surface and under the creek. Although the subsurface ERT electrodes were relatively shallow, this study illustrates the feasibility and utility of using horizontal directional drilling technology for emplacement of electrodes for ERT.
Using ERT with electrodes emplaced by directional drilling offers the potential for increasing the resolution of imaging around deep subsurface features of interest relative to using surface electrode arrays (Binley and Kemna 2005). Danielsen and Dahlin (2010) used numerical modeling to evaluate the resolution and sensitivity of ERT using horizontal boreholes for mining applications. They found that surveys containing cross-hole dipole-dipole and multiple gradient electrode configurations gave the best results. Power et al. (2015) evaluated the use of electrodes placed along horizontal boreholes for imaging dense, nonaqueous phase liquid (DNAPL) experiments in the laboratory and in field-scale DNAPL remediation scenarios. Combined application of surface and subsurface horizontal electrode arrays was found to provide significantly improved imaging results relative to using only surface electrodes.
The objective of the current study is to examine the feasibility of applying ERT for (1) characterization of the spatial extent of a PA underlying a thick vadose zone beneath a large industrial waste disposal complex at the Hanford Site in southeastern Washington State, and (2) monitoring potential remediation efforts associated with subsurface contamination in the deep, variably saturated sediments. The effectiveness of surface electrode arrays versus a combination of surface and horizontal subsurface electrode arrays is evaluated. Realistic field site conditions are simulated using a high-resolution subsurface flow and transport model developed from site characterization data, which is used as a physical basis for ERT simulations. ERT numerical simulations are used to determine the likelihood of success prior to any actual field deployment.
Materials and methods
Site description
The B-Complex, located within the 200 East Area of the Central Plateau on the Hanford Site in south-central Washington State, USA (Fig. 1), was selected as a test site. The site contains three tank farms. The B- and BX-tank farms each contain 12 single-shell, 530,000 gal (2,003,400 L), carbon steel waste-storage tanks, whereas the BY-tank farm contains 12 single-shell, 758,000 gal (2,865,240 L), carbon steel waste-storage tanks. All of the tanks contain mixtures of radioactive and chemical wastes produced during past nuclear weapons production activities. Unplanned releases of contaminants occurred by overfill events and leaks during and after the filling of some of these tanks. In addition, the site contains several major crib and trench disposal sites, tile fields, and French drains, where liquid wastes were intentionally disposed to the subsurface. The B-Complex, which received wastes from 1946–1974, is responsible for releasing nearly 346 million L of waste liquids containing nearly 14,000 kg of cyanide, 29,000 kg of chromium, 12,000 kg of uranium, and 145 Ci of technetium-99 into the groundwater (Serne et al. 2010). High concentrations of uranium, Tc-99, and nitrate have been found in groundwater extracted from the PA and underlying unconfined aquifer (Serne et al. 2010). The primary source of uranium contamination in the PA was an unplanned release from waste storage tank, BX-102 (Serne et al. 2010; see Fig. 1).
Aerial photograph of the B-Complex showing locations of waste storage tanks, groundwater monitoring wells, various waste disposal cribs and trenches, and surface electrode locations used in ERT modeling. Contamination of the perched water zone is attributed to an unplanned release from Tank BX-102 (shaded red)
Figure 2 shows a cutaway view (Fig. 2a) and east–west cross section (Fig. 2b; northing coordinate = 137,382 m) through the B-Complex showing the hydrostratigraphic units (HSUs), based on a geologic framework model (GFM) for the site (Springer 2018; Serne et al. 2010). From top to bottom, the HSUs include a thick sequence of highly permeable unconsolidated sands and gravels of the Pleistocene-age Hanford formation (units H1, H2, H3), deposited during a series of mega-floods. The H2 unit has been subdivided into coarse (C) and lower-permeability fine- (F) grained subunits (Serne et al. 2010). The Hanford formation is underlain by fluvial and lacustrine deposits of the Pliocene-age Cold Creek unit (CCU), which contains silt- (CCUz), sand- (CCUz_sand), and gravel-dominated (CCUg) subunits (Springer 2018; Oostrom et al. 2013). The feature of primary interest is a PA that is located within the CCU. The lowermost subunit of the PA is labeled “Perchsilt” in Fig. 2. The CCU is underlain by various subunits of fluvial and lacustrine deposits of the semi-consolidated Pliocene-age Ringold Formation, including the Taylor Flat member, and the unit E, Lower Mud unit, and unit A. Although present over a large area of the Hanford Site, the Taylor Flat member, unit E, and Lower Mud unit are absent in the immediate vicinity of the domain investigated for this study, so these units are not shown in Fig. 2. The Ringold Formation is underlain by basalt of the Columbia River basalt group.
Hydrostratigraphic units underlying the B-Complex used in flow and transport modeling. a The top of the perching silt unit is shown, and b the vertical extent of the PA is shown. The cross section shown (b) is for the northing coordinate 137,382 m, which passes through the deepest part of the PA. Vertical exaggeration = 5×
Physical and hydraulic properties that were assigned to these units for flow and transport modeling are listed in Table 1. These parameters were based on prior modeling efforts and site characterization data (Oostrom et al. 2013, 2017; Rockhold et al. 2018a, b). The parameters Ksxx, Ksyy, and Kszz are the saturated hydraulic conductivities in the x-, y-, and z-directions, respectively. The parameters θs, Sr, α, and n are the saturated water content, residual saturation, and two water retention parameters for the van Genuchten (1980) model, respectively. The α parameter is the inverse of the air-entry pressure, and the n parameter affects the slope of the water retention function for the porous media. The parameter ρs is the grain density.
Pump-and-treat operations at the field site are ongoing, currently using three extraction wells screened within the PA. The permeability of the PA is relatively low, however, so extraction rates are limited (Oostrom et al. 2013; Rockhold et al. 2018b). Reasonable borehole coverage exists at the site (Fig. 1) but borehole samples are insufficient to delineate the entire spatial extent of the PA or to allow for optimizing the placement of pump-and treat extraction or monitoring wells. Supplemental characterization and monitoring approaches are therefore needed.
Subsurface flow and transport modeling
A conceptual-numerical flow and transport model of the B-Complex was developed based on an existing GFM (Springer 2018) for the site (Fig. 2), and additional characterization data from Serne et al. (2010). Simulations of water flow and solute transport in the variably saturated porous media were performed using eSTOMP (Fang et al. 2015; White and Oostrom 2000, 2006). The numerical model domain spans 800 m in both the easting and northing directions, and 101 m in the vertical direction (elevation range: 110–211 m). The model was discretized using variable grid spacing with 144 × 144 × 184 grid blocks in the x-y-z directions, respectively, for a total of 3,815,424 grid blocks. Spatial discretization ranges from 0.1 to 2 m in the vertical direction and 4–10 m in the horizontal direction, with finer discretization used in the vicinity of the tanks, the PA, and the fine-grained H2 units. This discretization is finer than what has been used in earlier three-dimensional (3D) models of the site (Rockhold et al. 2018a). Neumann-type flux boundaries are applied to the upper surface for the flow equation to represent natural groundwater recharge rates (Fayer and Keller 2007; Last et al. 2006). For the B-Complex area, Fayer and Keller (2007) estimated long-term average recharge rates ranging from 92 mm/year for the gravel-covered surface of the tank farm with no vegetation, to 2.8 mm/year for undisturbed areas with sandy loam soil and shrub vegetation. A combination of Dirichlet-type hydraulic head and Neumann-type seepage face boundary conditions is applied to the lateral sides of the model domain for the flow equation, and pressures are determined using measured water levels from a well monitoring network (refer to Fig. 1). Outflow conditions were assumed for solutes at all lateral boundaries. No-flow conditions were assumed for both water and solutes at the bottom boundary of the domain, which is in basalt. Source terms representing historical releases of liquid waste from tanks, cribs, and trenches were incorporated in the model from published records (Serne et al. 2010; Rockhold et al. 2018a).
Flow and transport modeling were performed for the time period from year 0 to 2045, with years 0 to 1945 representing a period used to establish a steady-state flow field for a pre-Hanford operational setting. Simulation of liquid waste and disposal of contaminants of concern (e.g., nitrate, Tc-99, uranium) from 1946 to 1972 was based on historical records (Serne et al. 2010; Rockhold et al. 2018a). Extraction of contaminated groundwater from the PA at the site began in 2011. Removal of contaminated water from a single hypothetical well screened within the deepest part of the PA was simulated for the time period from 2011 to 2045 to evaluate the feasibility of using horizontal subsurface ERT electrodes to image changes in the PA attributable to pump-and-treat operations. A single hypothetical well was simulated for clarity of presentation.
Electrical resistivity tomography
Electrical resistivity is a measure of how strongly a material resists (inversely conducts) the flow of an electric current. ERT constructs an image of the electrical resistivity (or conductivity) distribution in the subsurface based on the inversion of data determined by inducing a known current (I) between pairs of current electrodes, and measuring the voltage drop (ΔV) across pairs of potential electrodes (Loke and Barker 1996; Friedel 2003; Binley 2015) to produce measurements of electrical resistance (\( R=\frac{\Delta V}{I} \), in ohms). The measurement resolution is determined by the spacing between the transmitting and receiving electrodes, which can be placed in surface (Cassiani et al. 2006) and/or borehole configurations (Binley et al. 2002; Daily and Ramirez, 2000; Daily et al. 2004) that can be optimized to image targeted regions of interest. Subsurface horizontal emplacement of electrodes is also potentially possible using directional drilling methods but has not yet been tested at the Hanford Site.
Buried metallic infrastructure such as boreholes, underground piping, and tanks redistribute subsurface current flow during ERT measurements and can have a significant impact on ERT images, thereby reducing the utility of ERT. Johnson et al. (2012) and Johnson and Wellman (2013) demonstrated a method of removing the effects of buried infrastructure by explicitly modeling the infrastructure in the ERT imaging algorithm in the E4D computational mesh (Johnson 2014). This method was used by explicitly incorporating borehole, underground piping, and underground tanks in the ERT modeling (Fig. 3).
Electrode and metallic infrastructure included in the ERT modeling. ERT scenarios consisted of surface electrodes (200 total) and surface plus below-surface electrodes (400 total). Dry wells are shown in addition to groundwater monitoring wells shown in Fig. 1
An unstructured tetrahedral mesh was used with 1,679,184 elements, which includes a more finely discretized region between the elevations of 140–121 m to provide better delineation of the spatiotemporal variability in bulk electrical conductivity within the PA. A water-table boundary was included at elevation 121 m. Surface topography was incorporated at 20-m resolution and from this information nearest neighbor elevations were used at electrode locations.
Transformation of flow and transport parameters to bulk electrical conductivity
Porosities, simulated water saturations, and nitrate concentrations output from eSTOMP were used to estimate bulk electrical conductivities using Archie’s Law (Archie 1942). Archie’s Law (Archie 1942) relates the bulk electrical conductivity to the primary factors that influence it (e.g., porosity, water saturation, ionic strength, or concentration of pore water):
where σw is the fluid conductivity, ϕint is the interconnected porosity, and S is the aqueous saturation. The cementation exponent m is dependent on the rate of change in pore complexity with porosity (Yue 2019), and on particle shape and orientation (Niu and Zhang 2018), and typically varies between 1.2 and 4.4 (Lesmes and Friedman 2005). A value of 1.8, which has been previously applied to represent Hanford sediments (Johnson and Wellman 2013), was used. The saturation exponent n is associated with the additional tortuosity due to the replacement of pore fluid with air (an insulator). A typical value of n = 2 was used in this study (e.g., Brunet et al. 2010; Day-Lewis et al. 2005). Fluid conductivity σw is the summation of the background groundwater conductivity and the contribution from nitrate, if any exists at a given location. To convert nitrate to fluid specific conductance, 34 unique well locations were identified in this area and a total of 667 total records were retrieved over the date range 12/12/1983–11/2/2018. A linear regression of nitrate (kg/L) versus σw (S/m) produced a linear coefficient equal to 0.15 (kg/L/S/m) with a coefficient of determination (R2) equal to 0.61 (Fig. 4). The linear coefficient value of 0.15 was used to convert nitrate concentrations (if any) to fluid specific conductance. A median value equal to 0.05 S/m (average = 0.06 S/m) was used as the background conductivity, based on an expanded data records review across the Hanford Site.
Experimental design (eSTOMP scenarios and ERT design)
Flow and transport model simulation results representing two snapshots—a pre-extraction condition in 2010 and a post-extraction condition in 2020—were used for ERT simulations. Bulk electrical conductivities were translated to the E4D computational mesh using tri-linear interpolation (Johnson et al. 2017).
Electrical resistance data for the years 2010 and 2020 were generated using two different electrode layouts: two surface electrode lines (Figs. 1 and 3) consisting of 100 electrodes spaced 5 m apart (200 total electrodes); and the same two surface electrode lines with two identical subsurface electrode lines directly beneath the surface lines at the 130 m elevation within the PA (400 total electrodes), emplaced through directional drilling. The surface electrode measurements (200 total electrodes) consisted of 11,400 cross-well, dipole-dipole, and Wenner-Schlumberger measurements designed to capture shallow and deep features with both large and small dipole offsets. The surface and subsurface electrode measurements (400 total electrodes) consisted of 38,445 1D (dipole, Wenner-Schlumberger), two-dimensional (2D) cross-well (current and potential electrodes in cross borehole configurations), and 3D cross-well (current and potential electrodes across three- or four-electrode lines) measurements. The large and small dipole offsets in the four-line measurement sequence were designed to capture features both near and far from the electrode locations and randomly distributed noise (2% normally) was added to the synthetic data to represent field conditions.
Time-lapse inverse ERT modeling was performed using the noisy synthetic ERT data sets for the two- and four-electrode line scenarios from 2010 and 2020. This was achieved by first inverting a baseline model from the 2010 synthetic data (i.e., one baseline model for the two surface lines and another baseline model for the four-electrode line scenario). Then changes from the 2010 baseline model were inverted using the 2020 noisy synthetic data set. Major changes affecting bulk electrical conductivity (i.e., saturation and nitrate concentration) occurred below an elevation of 140 m (more information in section ‘Conclusions’). This information was used in the time-lapse ERT inversion to only invert for changes within the PA zone defined in the E4D mesh (between elevations 140 and 121 m) to determine the PA spatial extent and evaluate the region influenced by pump-and-treat operations.
Results
Flow and transport
Output flow and transport variables of saturation (S) from eSTOMP and groundwater fluid conductivity (σw) change over time from 2010 to 2020 and therefore affect the transformation to bulk electrical conductivity (EC) used in the ERT simulations. Simulated saturation distributions for east-west cross sections through the model domain in 2010 and 2020 are shown in Fig. 5. The figure shows elevated saturations in the fine-grained H2 subunits, and water-saturated conditions within the PA and below the regional unconfined aquifer in 2010. In 2020, the simulation results show partial desaturation of the PA in response to the hypothetical extraction well. The simulated elevation of the regional water table is also shown to drop slightly between years 2010 and 2020, based on boundary conditions that were determined from observed water levels in monitoring wells.
Cross sections of simulated aqueous saturations through the B-Complex in a 2010 and b 2020. The hypothetical extraction well, screened within the PA, is shown as the vertical white line. The northing coordinate of the cross section is 137,382 m, which passes through the deepest part of the PA. Vertical exaggeration = 5×
Simulated nitrate concentrations are shown in east-west cross sections through the model domain in 2010 and 2020 (Fig. 6). While there have been no recent releases of nitrate, slow downward migration of nitrate occurs as a result of simulated natural groundwater recharge, as well as water extraction in the PA. Generally, the largest changes in nitrate concentrations occur below an elevation of 140 m, which was presumed to be the maximum elevation of the PA in the ERT simulations. These nitrate concentrations, together with the simulated saturations and porosities, were used to calculate bulk electrical conductivities using Archie’s Law (refer to Eq. 1).
Cross sections of simulated nitrate concentration through the B-Complex in a 2010 and b 2020 showing the effects of water extraction from the PA. The plumes on the left sides of the figure are from the BX trenches, shown in Fig. 1. The northing coordinate of the cross section is 137,382 m, which passes through the deepest part of the PA. Vertical exaggeration = 5×
Figure 7 shows simulated lowering of the water level in the hypothetical extraction well and associated water removal rates. Lowering of the water level was modeled using a combination of specified aqueous pressure (Dirichlet) and seepage face boundary conditions applied to an internal region of the model domain representing the extraction well. Water removal rates were then calculated by summing the water fluxes passing into the well region over time. Simulated extraction rates are shown to drop dramatically around year 2014 due to dewatering of the region around the extraction well. The permeability of the sediments in the PA is relatively low, such that small extraction rates are required to maintain water in the well. This suggests that the efficiency of remediation efforts for the PA involving pump-and-treat operations may be low.
Figure 8 represents states of flow and transport parameters transformed to bulk EC using Archie’s Law from 2010 (Fig. 8a) and 2020 (Fig. 8b). These figures represent the “true” bulk EC from which the feasibility of using ERT to image changes in the PA can be determined. The plan view images (top) are sliced at elevation 130 m, which is within the PA. Note the reduction in bulk EC from 2010 to 2020 as the water extraction reduces the volume within the PA.
Vadose zone conceptual site model of bulk electrical conductivity [S/m] for a 2010, plan view (top) sliced at elevation 130 m and elevation view (bottom) sliced at N 137395 m; b 2020, plan view (top) and elevation view (bottom). The change in the images from 2010 to 2020 demonstrates a decrease in conductivity within the perched water aquifer as a result of pumping activities. The piping, tanks, and wells incorporated as metallic infrastructure are shown. The vertical exaggeration in the bottom images is 2:1
Electrical resistivity tomography
Electrical resistivity tomography is not typically used for imaging complex industrial waste sites where significant metallic infrastructure exists (e.g., underground piping, tanks, and wells), because this infrastructure has a significant impact on current flow during resistivity measurements. Consequently, the presence of such metallic infrastructure has major implications for modeling subsurface current flow near the location of injection electrodes. It has been shown that improved ERT imaging results can be obtained if metallic infrastructure, and other known electrical conductivity constraints, are explicitly accounted for in ERT model inversions (Johnson et al. 2012; Johnson and Wellman 2013). These capabilities are highlighted here because their use may be critical to the success of ERT for imaging and monitoring changes occurring in the deep subsurface at complex industrial waste sites where remediation activities are planned or taking place.
Figure 9 demonstrates the theoretical potential field generated from the injection of current in two electrodes adjacent to an underground tank and piping for the study site. Figure 9a is the potential field without including these features, and Fig. 9b results from explicitly including this metallic infrastructure in the simulations. Visually, the extent of the potential field is reduced when metallic infrastructure is included in the modeling; therefore, the resistance value should decrease for measurements using these current electrodes (shown graphically in Fig. 10). Resistance values are shown for measurements using the current injection electrodes in Fig. 9 and potential electrodes at an increasing distance from the current electrodes. Using the negative current injection electrode as a spatial reference, the x-axis labels in Fig. 9 is the midpoint distance between current and potential dipoles. In all cases, modeling metallic infrastructure results in a decrease of resistance. Inaccurate potential fields can result in artificially high electrical conductivity zones in the vicinity of the metallic infrastructure.
Equipotential distributions for a current injection surrounded by metallic infrastructure where metallic boundaries are a not incorporated and b incorporated into the ERT modeling. Dry wells in addition to groundwater monitoring wells (refer to Fig. 1) are included
Figure 11a represents the baseline model for the surface electrode scenario. Where only surface electrodes are used for imaging, limited structure is resolved in both the plan view sliced at 130 m (top) and elevation view (bottom). In the time-lapse ERT inversion, there is a reduction in conductivity near the extraction well, but the changes are subtle. These results indicate that the use of surface electrodes alone has limited utility for this application.
Vadose zone inversion results from surface ERT for a 2010 showing a plan view (top) within the perched water aquifer sliced at elevation 130 m and an elevation view (bottom) sliced at N137400 m and b 2020 plan view (top) and elevation view (bottom) images. The vertical exaggeration in the bottom image is 2:1. The white-dashed line denotes the maximum assumed top elevation of the perched water aquifer in the ERT modeling
Figure 12 represents the scenario with two surface and two subsurface electrode lines (400 electrodes total) emplaced through directional drilling. Figure 12a is for year 2010 and represents the baseline model. Figure 12b represents year 2020. The white-dashed line at elevation 140 m represents the top boundary of the finely discretized region assumed to be the boundary in the ERT modeling of the PA. There is a visible reduction in bulk EC in both the plan (top) and elevation (bottom) views within the PA from 2010 (Fig. 11a) to 2020 (Fig. 11b).
Vadose zone inversion results from surface and below-surface ERT for a 2010 showing a plan view (top) within the perched water aquifer sliced at elevation 130 m and an elevation view (bottom) sliced at N137400 m; b 2020 plan view (top) and elevation view (bottom) images. The vertical exaggeration in the bottom images is 2:1. The white-dashed line denotes the maximum assumed top elevation of the perched water aquifer in the ERT modeling
To demonstrate and compare the changes occurring in the “true” model to the ERT model, the change in logarithmic bulk EC from 2010 to 2020 was calculated. Figure 13a is the “true” change in bulk EC from 2010 to 2020 (i.e., subtracting Fig. 8a from b), and Fig. 13b is the change detected in the ERT model (i.e., subtracting Fig. 12a from b). The highest sensitivity of the ERT imaging is in between the electrode strings and this region is well-delineated as being the region with the greatest change.
Discussion
Standard characterization and monitoring methods typically rely on data obtained from sparse, vertically oriented wells. Technologies such as ERT can be used to augment these well-based methods. Although ERT is well established, to date most field applications of ERT for characterization and monitoring of hazardous waste sites have relied on the use of surface electrodes, owing to their relative ease of deployment. It is well known, however, that the imaging resolution obtained from ERT using surface electrodes decreases with depth, which is an especially important consideration for some arid waste sites that have a very thick vadose zone.
A comparison of Figs. 11 and 12 clearly shows that the ERT results obtained using only surface electrodes provide very limited information for this complex waste site and do not reveal information about the key subsurface feature of interest, the PA. The results also show that having electrodes in the vicinity of the PA yields significantly improved imaging results for this region of interest. With the combined surface and subsurface electrode configuration, the dewatering of the PA during simulated pump-and-treat operations was clearly visible, and changes in bulk EC resulting from the simulated water extraction approximate the extent of the PA for the modeled scenario. The improved imaging results obtained in this study using horizontal subsurface electrodes are consistent with results obtained by Danielsen and Dahlin (2010) for mining applications, and by Power et al. (2015) for DNAPL remediation scenarios.
Use of subsurface electrode arrays offers the potential for significantly improving the ability to characterize the deep subsurface, including features such as PAs, and to better monitor remediation progress. Directional drilling is a mature technology that is being used in subsurface remediation applications (Kaback et al. 1992; Parmentier and Klemovich 1996). However, its potential use for emplacement of subsurface ERT electrodes to supplement site characterization and monitoring for waste management applications has not been previously demonstrated, and the logistics of mounting electrodes on the exterior of a conduit emplaced in the deep subsurface beneath an industrial waste site by directional drilling need to be explored.
Conclusions
This paper presents an application of ERT using both surface and horizontal subsurface electrodes for imaging a contaminated, deep, PA system, and changes within this system resulting from pump-and-treat operations. Known electrical conductivity constraints associated with the water table, and the effects of metallic infrastructure (waste tanks, well casing, including horizontal wells and pipes) on current flow, are accounted for in ERT model inversions. Results show that using surface electrodes for ERT imaging was insufficient to resolve simulated changes occurring within the PA system. With the surface electrode only configuration, the 2010 baseline image (Fig. 11) also showed limited subsurface structure. However, results show that using horizontal subsurface electrodes, which can be emplaced by horizontal drilling, can significantly improve the utility of ERT for imaging the PA and changes associated with remediation activities. These results suggest that further investigation into the use of directional drilling for emplacement of horizontal electrode arrays for ERT is warranted. Combining these technologies offers the potential for significantly improving the ability to characterize the subsurface and monitor remediation progress at complex waste sites.
References
Archie GE (1942) The electrical resistivity log as an aid in determining some reservoir characteristics. Trans AIME 146(01):54–62
Binley A (2015) Tools and techniques: electrical methods. Treatise Geophys 233–259. https://doi.org/10.1016/B978-0-444-53802-4.00192-5
Binley A, Kemna A (2005) DC resistivity and induced polarization methods. In: Hydrogeophysics. Springer, Dordrecht, The Netherlands, pp 129–156
Binley A, Cassiani G, Middleton R, Winship P (2002) Vadose zone flow model parameterisation using cross-borehole radar and resistivity imaging. J Hydrol 267(3–4):147–159
Binley A, Hubbard SS, Huisman JA, Revil A, Robinson DA, Singha K, Slater LD (2015) The emergence of hydrogeophysics for improved understanding of subsurface processes over multiple scales. Water Resour Res 51(6):3837–3866
Brunet P, Clément R, Bouvier C (2010) Monitoring soil water content and deficit using electrical resistivity tomography (ERT): a case study in the Cevennes area, France. J Hydrol 380(1–2):146–153
Busato L, J Boaga, MT Perri, G Cassiani (2014) Time-lapse monitoring of the hyporheic zone of an alpine river using non-invasive methodologies. In: Proceedings of the 33rd Gruppo Nazionale di Geofisica della Terra Solida (GNGTS), Bologna, Italy, 25–27 November 2014, pp 129–135. http://www3.ogs.trieste.it/gngts/index.php/2014-xxxiii-bo;. Accessed 9 Jan 2020
Carroll KC, Taylor R, Gray E, Brusseau ML (2009) The impact of composition on the physical properties and evaporative mass transfer of a PCE-diesel immiscible liquid. J Hazard Mater 164(2–3):1074–1081
Cassiani G, Binley A (2005) Modeling unsaturated flow in a layered formation under quasi-steady state conditions using geophysical data constraints. Adv Water Resour 28(5):467–477
Cassiani G, Bruno V, Villa A, Fusi N, Binley A (2006) A saline trace test monitored via time-lapse surface electrical resistivity tomography. J Appl Geophys 59(3):244–259
Chambers JE, Kuras O, Meldrum PI, Ogilvy RD, Hollands J (2006) Electrical resistivity tomography applied to geologic, hydrogeologic, and engineering investigations at a former waste-disposal site. Geophysics 71(6):B231–B239
Chambers JE, Wilkinson PB, Wardrop D, Hameed A, Hill I, Jeffrey C, Loke MH, Meldrum PI, Kuras O, Cave M, Gunn DA (2012) Bedrock detection beneath river terrace deposits using three-dimensional electrical resistivity tomography. Geomorphology 177–178:17–25
Cultrera M, Boaga J, Di Sipio E, Dalla Santa G, De Seta M, Galgaro A (2018) Modeling an induced thermal plume with data from electrical resistivity tomography and distributed temperature sensing: a case study in Northeast Italy. Hydrogeol J 26:837–851
Dahlin T (2001) The development of DC resistivity imaging techniques. Comput Geosci 27:1019–1029
Daily W, Ramirez A (2000) Electrical imaging of engineered hydraulic barriers. Geophysics 65(1):83–94
Daily W, Ramirez A, LaBrecque D, Nitao J (1992) Electrical resistivity tomography of vadose water movement. Water Resour Res 28(5):1429–1442
Daily W, Ramirez A, Binley A, LeBrecque D (2004) Electrical resistance tomography. Lead Edge 23(5):438–442
Danielsen BE, Dahlin T (2010) Numerical modelling of resolution and sensitivity of ERT in horizontal boreholes. J Appl Geophys 70(3):245–254
Day-Lewis FD, Singha K, Binley A (2005) Applying petrophysical models to radar travel time and electrical resistivity tomograms: resolution-dependent limitations. J Geophys Res 110(B8)
Fang Y, Appriou D, Bacon DH et al (2015) eSTOMP online user guide. http://stomp.pnnl.gov/estomp_guide/eSTOMP_guide.stm. Accessed April 2020
Fayer MJ, Keller JM (2007) Recharge data package for Hanford Single-Shell Tank Waste Management Areas (PNNL-16688). Pacific Northwest National Lab, Richland, WA
Freeze RA, Cherry JA (1979) Groundwater. Prentice-Hall, Englewood Cliffs, NJ
Friedel S (2003) Resolution, stability and efficiency of resistivity tomography estimated from a generalized inverse approach. Geophys J Int 153(2):305–316
Johnson TC (2014) E4D: a distributed memory parallel electrical geophysical modeling and inversion code user guide, version 1.0. PNNL-SA-23783, Pacific Northwest National Lab, Richland, WA
Johnson TC, Wellman DM (2013) Re-inversion of surface electrical resistivity tomography data from the Hanford Site B-Complex. PNNL-22520; RPT-DVZ-AFRI-014, Pacific Northwest National Lab, Richland, WA
Johnson TC, Versteeg RJ, Ward A, Day-Lewis FD, Revil A (2010) Improved hydrogeophysical characterization and monitoring through parallel modeling and inversion of time-domain resistivity and induced-polarization data. Geophysics 75(4):1JA–Z98
Johnson TC, Versteeg RJ, Rockhold ML, Slater LD, Ntarlagiannis D, Greenwood WJ, Zachara J (2012) Characterization of a contaminated wellfield using 3D electrical resistivity tomography implemented with geostatistical, discontinuous boundary, and known conductivity constraints. Geophysics 77(6):EN85–EN96
Johnson TC, Hammond GE, Chen X (2017) PFLOTRAN-E4D: a parallel open source PFLOTRAN module for simulating time-lapse electrical resistivity data. Comput Geosci 99:72–80
Kaback DS, Looney BB, Eddy CA, Hazen TC (1992) Innovative ground water and soil remediation: in situ air stripping using horizontal wells. In: Proceeding of the Fifth National Outdoor Action Conference on Aquifer Restoration, Ground Water Monitoring, and Geophysical Methods, Las Vegas, NV, 1991
Kuras O, Wilkinson PB, Meldrum PI, Oxby LS, Uhlemann S, Chambers JE, Atherton N (2016) Geoelectrical monitoring of simulated subsurface leakage to support high-hazard nuclear decommissioning at the Sellafield site, UK. Sci Total Environ 566:350–359
Last GV, Freeman EJ, Cantrell KJ, Fayer MJ, Gee GW, Nichols WE, Horton DG (2006) Vadose zone hydrogeology data package for Hanford assessments. PNNL-14702 rev. 1, Pacific Northwest National Lab, Richland, WA
Lesmes DP, Friedman SP (2005) Relationships between the electrical and hydrogeological properties of rocks and soils. In: Hydrogeophysics. Springer, Dordrecht, The Netherlands, pp 87–128
Loke MH, Barker RD (1996) Rapid least-squares inversion of apparent resistivity pseudosections by quasi-Newton method. Geophys Prospecting 44(1):131–152
Marinenko AV, Epov MI, Olenchenko VV (2019) Solving direct problems of electrical resistivity tomography for media with high-conductivity irregular-shaped heterogeneities by an example of a multiple well platform. J Appl Indust Math 13(1):93–102
Nimmo JR, Rousseau JP, Perkins KS, Stollenwerk KG, Glynn PD, Bartholomay RC, Knobel LL (2004) Hydraulic and geochemical framework of the Idaho National Engineering and Environmental Laboratory vadose zone. Vadose Zone J 3(1):6–34
Niu Q, Zhang C (2018) Physical explanation of Archie’s porosity exponent in granular materials: a process-based, pore-scale numerical study. Geophys Res Lett 45(4):1870–1877
Oostrom M, Truex MJ, Carroll KC, Chronister GB (2013) Perched-water analysis related to deep vadose zone contaminant transport and impact to groundwater. J Hydrol 505:228–239
Oostrom M, Truex MJ, Rockhold ML, Johnson TC (2017) Deep vadose zone contaminant flux evaluation at the Hanford BY-cribs site using forward and imposed concentration modeling approaches. Environ Proc 4(4):771–797
Park S, Yi MJ, Kim JH, Shin SW (2016) Electrical resistivity imaging (ERI) monitoring for groundwater contamination in an uncontrolled landfill, South Korea. J Appl Geophys 135:1–7
Parmentier PP, Klemovich RM (1996) A new direction in remediation. Civ Eng 66(4):55
Power C, Gerhard JI, Tsourlos P, Soupios P, Simyrdanis K, Karaoulis M (2015) Improved time-lapse electrical resistivity tomography monitoring of dense non-aqueous phase liquids with surface-to-horizontal borehole arrays. J Appl Geophys 112:1–13
Robinson BA, Houseworth JE, Chu S (2012) Radionuclide transport in the unsaturated zone at Yucca Mountain, Nevada. Vadose Zone J 11(4)
Robinson J, Johnson T, Rockhold M (2019) Feasibility assessment of long-term electrical resistivity monitoring of a nitrate plume. Groundwater. https://doi.org/10.1111/gwat.12899
Rockhold ML, Song X, Tagestad JD, Thorne PD, Tartakovsky GD, Chen X (2018a) Sensitivity analysis of contaminant transport from vadose zone sources to groundwater. PNNL-28065, Pacific Northwest National Lab, Richland, WA
Rockhold ML, Spane FA, Wietsma TW, Newcomer DR, Clayton RE, Demirkanli I, Saunders DL, Truex MJ, Valenta-Snyder MM, Thompson CJ (2018b) Physical and hydraulic properties of sediments from the 200-DV-1 operable unit. PNNL-27846, RPT-DVZ-CHPRC 0005, rev 0, Pacific Northwest National Lab, Richland, WA
Rucker DF, Fink JB (2007) Inorganic plume delineation using surface high-resolution electrical resistivity at the BC cribs and trenches site. Vadose Zone J 6:946–958
Rucker DF, Loke MH, Levitt MT, Noonan GE (2010) Electrical-resistivity characterization of an industrial site using long electrodes. Geophysics 75(4):WA95–WA104
Serne RJ, Bjornstad BN, Keller JM, Thorne PD, Lanigan DC, Christensen JN, Thomas GS (2010) Conceptual models for migration of key groundwater contaminants through the vadose zone and into the upper unconfined aquifer below the B-complex. PNNL-19277, Pacific Northwest National Lab, Richland, WA
Slater LD, Ntarlagiannis D, Day-Lewis FD, Mwakanyamale K, Versteeg RJ, Ward A, Strickland C, Johnson CD, Lane JW Jr (2010) Use of electrical imaging and distributed temperature sensing to characterize surface water-groundwater exchange regulating uranium transport at the Hanford 300 area, Washington. Water Resour Res 46:W10533. https://doi.org/10.1029/2010WR009110
Springer SD (2018) Model Package report: Central Plateau Vadose Zone Geoframework version 1.0. CP-60925, Rev. 0, Hanford Site (HNF), Richland, WA
van Genuchten MT (1980) A closed-form equation for predicting the hydraulic conductivity of unsaturated soils. Soil Sci Soc Am J 44(5):892–898
Wellman DM, Truex MJ, Johnson TC, Bunn AL, Golovich EC (2013) Deep vadose zone–applied field research initiative fiscal year 2012 annual report. PNNL-22322, Pacific Northwest National Lab, Richland, WA
White MD, Oostrom M (2000) STOMP subsurface transport over multiple phases theory guide, version 2. PNNL-12030, Richland, WA. https://stomp.pnl.gov. Accessed 4 May 2020
White MD, Oostrom M (2006) STOMP subsurface transport over multiple phases, version 4: user’s guide. PNNL-15782 (UC-2010), Richland, WA. https://stomp.pnl.gov. Accessed 13 April 2020
Wu YS, Ritcey AC, Bodvarsson GS (1999) A modeling study of perched water phenomena in the unsaturated zone at Yucca Mountain. J Contam Hydrol 38(1–3):157–184
Yue W (2019) Pore-scale explanation of the Archie’s cementation exponent: microstructure, electrical anisotropy, and numerical experiments. Geophys Res Lett. https://doi.org/10.1029/2019GL082585
Funding
This document was prepared under the Deep Vadose Zone – Applied Field Research Initiative at Pacific Northwest National Laboratory. The Pacific Northwest National Laboratory is operated by Battelle Memorial Institute for the US Department of Energy under Contract DE-AC05-76RL01830.
Author information
Authors and Affiliations
Corresponding author
Rights and permissions
Open Access This article is licensed under a Creative Commons Attribution 4.0 International License, which permits use, sharing, adaptation, distribution and reproduction in any medium or format, as long as you give appropriate credit to the original author(s) and the source, provide a link to the Creative Commons licence, and indicate if changes were made. The images or other third party material in this article are included in the article's Creative Commons licence, unless indicated otherwise in a credit line to the material. If material is not included in the article's Creative Commons licence and your intended use is not permitted by statutory regulation or exceeds the permitted use, you will need to obtain permission directly from the copyright holder. To view a copy of this licence, visit http://creativecommons.org/licenses/by/4.0/.
About this article
Cite this article
Rockhold, M.L., Robinson, J.L., Parajuli, K. et al. Groundwater characterization and monitoring at a complex industrial waste site using electrical resistivity imaging. Hydrogeol J 28, 2115–2127 (2020). https://doi.org/10.1007/s10040-020-02167-1
Received:
Accepted:
Published:
Issue Date:
DOI: https://doi.org/10.1007/s10040-020-02167-1