Abstract
Sea Surface Temperature (SST) plays an important role in marine ecology. SST prediction raises considerable attention in ocean-related fields. Recently, deep learning models are widely used in SST prediction, but it is not easy to obtain optimal prediction results using historical observation data directly. More than temporal information, SST data also contains other features, such as trend, periodicity and disturbance. In this paper, we proposed a Multi-Channel LSTM (MC-LSTM) model to improve SST prediction. Firstly, a wavelet transform is used to decompose time-sequence data into multiple sequences representing trend, period and disturbance respectively. Secondly, we use multiple LSTM channels to train these data in parallel, and then obtain the combined prediction results. MC-LSTM can predict Sea surface temperature only by historical SST data, without the help of other spatial and climatic information, so it is easy to get the data. Compared with the direct use of LSTM for prediction, MC-LSTM can improve the prediction accuracy by 26%.
Export citation and abstract BibTeX RIS
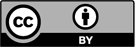
Content from this work may be used under the terms of the Creative Commons Attribution 3.0 licence. Any further distribution of this work must maintain attribution to the author(s) and the title of the work, journal citation and DOI.