Abstract
We estimate the costs of climate change to US agriculture, and associated potential benefits of abating greenhouse gas emissions. Five major crops' yield responses to climatic variation are modeled empirically, and the results combined with climate projections for a no-policy, high-warming future, as well as moderate and stringent mitigation scenarios. Unabated warming reduces yields of wheat and soybeans by 2050, and cotton by 2100, but moderate warming increases yields of all crops except wheat. Yield changes are monetized using the results of economic simulations within an integrated climate-economy modeling framework. Uncontrolled warming's economic effects on major crops are slightly positive—annual benefits <$4 B. These are amplified by emission reductions, but subject to diminishing returns—by 2100 reaching $17 B under moderate mitigation, but only $7 B with stringent mitigation. Costs and benefits are sensitive to irreducible uncertainty about the fertilization effects of elevated atmospheric carbon dioxide, without which unabated warming incurs net costs of up to $18 B, generating benefits to moderate (stringent) mitigation as large as $26 B ($20 B).
Export citation and abstract BibTeX RIS
1. Introduction
What are the costs and benefits to US agriculture of mitigating greenhouse gas (GHG) emissions? Agriculture has significant climate change exposure, but despite being a sector that has long been studied (e.g., Mendelsohn et al 1994), projections of future impacts on crops, and the associated costs of damage, remain too uncertain to provide a definitive answer. The issue is highlighted by disagreements over the responses of US agricultural yields and profits inferred from historical observations, and their implications for the sign and magnitude of future climate impacts.
The empirical climate economics literature provides ample evidence that yields of major US crops are adversely affected by exposure to cumulative growing season degree day extremes (Schlenker et al 2006, Fisher et al 2012) and temperatures above a 86 °F (30 °C) threshold (Schlenker and Roberts 2009, Ortiz-Bobea 2013). But the economic consequences are contested. The robustness of accumulated heat's adverse effects on farm profits (Schlenker et al 2006, Fisher et al 2012) has been questioned in light of the potentially confounding influence of spatially and temporally varying non-climatic factors (Deschênes and Greenstone 2007, 2012). When combined with earth system model (ESM) simulations of future climate, the latter responses suggest that climatic changes experienced by 2100 would have only small impacts on today's agricultural system (annual losses of US (2002)-$4 B to -$16 B).
Additional uncertainty abounds in the future trajectory of production, and meteorological exposure, of US agriculture—even for a given warming scenario. Despite improved understanding of climate change feedbacks on land use (Hurtt et al 2011), the future geographic distribution and output expansion of US field crops remain indeterminate. With fixed cropping patterns and warming trajectories, assessment using meteorological exposures from an ensemble of ESMs can increase the range of projected impacts and the magnitude of 'worst-case' losses (Deschênes and Greenstone 2012, and especially Burke et al 2015). Addressing the latter uncertainty, the Inter-Sectoral Impact Model Intercomparison Project (ISI-MIP) uses global gridded crop models (GGCMs) forced by ESM ensemble projections to quantify the range of crop shocks to crop yields (Rosenzweig et al 2014), which are in turn employed as input forcings to integrated assessment models (IAMs) that simulate concomitant crop production, price and economic welfare impacts (Nelson et al 2014).
The dollar value of damages depends critically on the uncertain state of the economy in the future decades when climate change affects crop yields. However, the ISI-MIP impact modeling protocol's baseline socio-economic, technological and GHG mitigation trajectories are not synchronized with the assumptions used by the IAMs that simulate the representative concentration pathway scenarios forcing ESM projections. The key omission is the relative price effects of the GHG mitigation measures that are necessary to realize low-radiative forcing futures. Cost-benefit analysis requires a modeling framework that can simulate the economic effects of mitigation, the climatic consequences of the resulting emissions, the concomitant biophysical impacts and their effects on the perturbed economy. A further limitation is that the resulting economic impacts understate the potential benefits of GHG mitigation by including adaptation that arises out of IAMs' price-driven substitution responses—among the inputs to crop production and the outputs of agricultural sectors, and between other goods and agriculture, and domestic and imported varieties of each commodity (Nelson et al 2014)—whose cost-reducing effects are difficult to monetize5 .
This paper draws upon and extends aforementioned approaches to estimate the costs of climate change to US agriculture, and the potential benefits of GHG mitigation, in a manner that is both economically and climatically consistent, and exclusive of adaptation. We first econometrically model the long-run yield response of five major crops (corn, soybeans, wheat, cotton, sorghum) to climatic variation, using data on weather, output and harvested area for ∼3000 counties in the coterminous US over the period 1948–2010. We then combine the resulting yield responses with ESM simulations of climate change scenarios prepared for the US Environmental Protection Agency's climate change impacts and risk analysis (CIRA) project (Waldhoff et al 2014) to estimate yield changes under a no-policy high-warming future as well as two lower-warming emission mitigation scenarios. Finally, we use the resulting yield shocks in conjunction with the output of the computable general equilibrium (CGE) economic model that generated the CIRA emission scenarios (MIT-EPPA—Paltsev et al 2013) to calculate aggregate economic costs in terms of future revenue changes in each scenario. Cost differences between the no-policy and mitigation scenarios indicate the benefits of reducing GHG emissions.
We find that unmitigated climate change has substantial adverse effects on yields of soybeans and wheat by mid-century, and cotton by century's end, but compensating beneficial impacts on corn and sorghum yields. Climatic shocks exhibit substantial geographic variation, with yield increases (reductions) in regions that currently have cooler (warmer) average climates, and, over time, increasingly severe impacts at lower latitudes. If climatic changes projected by 2100 under the reference warming scenario were to occur today, annual major crop revenues would be largely unaffected. But once the agriculture sector's projected future expansion is taken into account, the upshot is an annual net benefit of US (2010) $3 B by 2050, which falls to $1.3 B by 2100. But forgoing less vigorous climate change is nonetheless costly. Both mitigation policy scenarios have net beneficial effects, up to $1.2 B by 2050 and $2 B by 2100 if climate change were to impact today's agricultural system, and $3.3 B by 2050 and almost $17 B by 2100 with the price and output level that are projected in the future. However, these results are sensitive to the specification of the carbon dioxide (CO2) fertilization effect (CFE), whose influence on yields is thought to be positive but subject to considerable uncertainty. Omitting the CFE flips the sign of our impact estimates, giving rise to net agriculture sector costs as high as $18 B, with attendant amplification of mitigation benefits.
The rest of the paper is organized as follows. Section 2 summarizes our methodology for empirically modeling climate-yield relationships and coupling these with ESM simulations. Section 3 presents the resulting yield responses to climate change, changes in crop output at the county and regional levels, and monetized damages. In section 4 we offer a summary of our findings and discussion of their caveats.
2. Methods
2.1. Empirical analysis: using historical observations to infer climate impact on yields
Following the recent climate-economics literature (Schlenker and Roberts 2006, 2009, Deschênes and Greenstone 2007, 2012, Lobell and Burke 2010, Ortiz-Bobea 2013, Burke and Emerick 2015) we quantify the potentially nonlinear influence of climate on yields using semi-parametric cross section-time series regressions. Previous studies exploit the historical co-variation between yields and weather shocks to infer the effects of future climate. Motivated by Burke and Emerick's (2015) finding that long-run adaptations are limited in their ability to alleviate the short-run impacts of extreme heat, we extend their approach using a dynamic modeling framework that statistically distinguishes between the effects of short-run (weather) and long-run (climate) shocks. Since farmers' planting, management and harvesting decisions are based on land quality and expectations of weather, yields and meteorological variables share a long-run equilibrium relationship. In any given year, weather shocks cause yields to diverge from their expected long-run values, prompting farmers to revise their long-run expectations, and make management decisions that can have persistent effects6 .
To statistically identify the former equilibrium and latter disequilibrium responses we employ an error-correction model (ECM)7
. Our data are an unbalanced panel of counties over
years, recording yields,
(calculated as the ratio of production,
to harvested area,
as well as three-hourly observations of growing season temperature, precipitation and soil moisture, indexed by
, respectively. Interannual variation in log annual yield (
is modeled as a function of a vector of county-specific effects (
which capture the influence of unobserved time-invariant local characteristics such as topography and soils), a vector of climatic covariates
within each annual growing season, the cumulative exposure over
crop growth stages to
temperature intervals,
precipitation intervals,
and
soil moisture intervals,
and a vector of time-varying county-level statistical controls
.
Our model, which is derived and explained in the supplementary information (SI), is written:
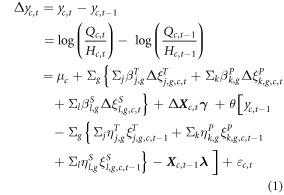
and is estimated via ordinary least squares for five crops (corn, soybeans, wheat, cotton, sorghum), indexed by Interannual difference terms (prefixed by
model the yield impacts of transitory disequilibrium shocks, the expression in square braces captures the long-run equilibrium relationship between yields and the covariates, and
is a random disturbance term. Parameters to be estimated are the disequilibrium (weather) impacts (βv), equilibrium (climate) impacts (ηv), short- and long-run effects of non-climatic variables (γ and λ), and the error-correction parameter (
measuring producers' speed of adjustment to the long-run equilibrium. The parameters (ηv) are vectors of semi-elasticities indicating the percentage by which yields shift relative to their conditional mean levels in response to additional time spent in a given interval. The vectors' elements—the individual coefficient estimates—each capture the distinct marginal effect of exposure within the corresponding interval (e.g., the average impact of an additional hour to 70–80 °F versus 80–90 °F temperatures). Collectively, the elements of
flexibly capture
overall long-run effect as a piecewise linear spline. The shape of the resulting function is identified from the covariation between observed yields and meteorology within each interval, as well as the distribution of observations across intervals over the historical period of the sample. With regard to temperature, the advantage of this approach is that it more precisely resolves the yield impacts of extreme heat relative to the standard degree-day specification (see Schlenker and Roberts 2009). Our dataset is described in the SI.
Omitted from equation (1) is the CFE. Rising CO2 concentrations are a time-varying shock that simultaneously affects yields in all counties. However, there is near-perfect collinearity between the CFE and long-run impacts of other strongly trending, spatially homogeneous, beneficial influences such as total factor productivity improvements or technological progress. Data constraints preclude quantification of the latter with accuracy sufficient to construct credible statistical controls8 . Given the potential for the long-run coefficient on CO2 concentrations to erroneously capture these confounding secular effects, we eschew empirical estimation of the CFE and instead incorporate its effect on our yield projections using relationships based on the literature.
2.2. Projecting yield impacts of future climate change
Climate change impacts are quantified by combining the fitted values of the equilibrium meteorological parameters ( with meteorological exposures derived from ESM simulations of different warming scenarios. We spatially aggregate simulated 3-hourly fields of temperature, precipitation and soil moisture to the county level (
and
and bin the results into the
and
intervals (respectively) over crop growth stages in current and future growing seasons to generate exact analogs of the regression covariates,
9
. Yields under irrigated and rainfed management regimes (indexed by
exhibit different responses to precipitation and moisture as well as elevated CO2. Accordingly, we model them separately (see SI), specifying rainfed impacts as a function of temperature, precipitation, soil moisture and ambient CO2 concentrations
and irrigated impacts as a functions of temperature and CO2:
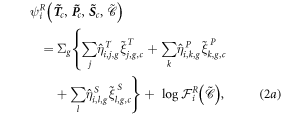
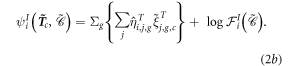
Here, is a concave function that captures the differential benefits of CO2 fertilization under different moisture stress conditions, based on Hatfield et al (2011) and McGrath and Lobell (2013). Our calibration of the CFE index is documented in the SI.
The terms indicate the partial effects of climate on the logarithm of irrigated and rainfed yields. Our normalized decadal index of climate impact is the yield ratio:
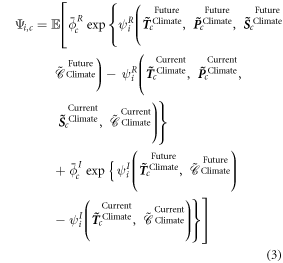
in which is the expectation operator and
denotes the average shares of irrigated and rainfed cultivation from the MIRCA dataset (Portmann et al 2010), which we treat as remaining fixed into the future. (This assumption is discussed in § 3.2.)
is interpretable as the climatically-attributable fractional change in a county's average yield relative to its own conditional mean10
. Accordingly, holding the current geographic distribution of harvested area constant as well, the change in production of each crop is simply the quantity
.
Our simulated meteorological fields and ambient CO2 concentrations are taken from the CIRA project (Waldhoff et al 2014), a 15-member ensemble of simulations using the MIT Integrated Global System Model-Community Atmosphere Model (IGSM-CAM) modeling framework (Monier et al 2013)11 . CIRA is underlain by three consistent socioeconomic and emissions scenarios: a reference scenario with unconstrained emissions and two climate stabilization scenarios that impose uniform global taxes on GHGs to limit total radiative forcing to 4.5 W m−2 and 3.7 W m−2 by century's end. Reductions in climate damages to agriculture in moving from the reference to the policy scenarios are interpretable as the benefits of GHG mitigation, and the associated differences in US agriculture sector output and relative prices are crucial to our cost estimates (Paltsev et al 2013). For each emission scenario, IGSM-CAM was run with different values of climate sensitivity and aerosol forcing, and different representations of natural variability, resulting in a 60-member ensemble (Monier et al 2015). We focus on simulations with a climate sensitivity of 3 °C, with each scenario run as a 5-member initial condition ensemble in an attempt to span the potential range of natural variability. Spatially disaggregating these projections to the county scale and using equations (2) and (3) enables us to calculate yield impacts at the middle and the end of the century (2036–2065 and 2086–2115) for each combination of scenario and ensemble member. We analyze 30-year time periods over 5 ensemble members with different representations of natural variability, resulting in a total of 150 years defining changes from the present day to the middle and end of the century, in order to obtain robust estimates of climate impacts on yield where the anthropogenic signal is extracted from the noise associated with natural variability.
3. Results
3.1. Yield responses to climate change
Our long-run estimates are for the most part broadly consistent with current agronomic understanding of weather effects on field crop yields. Space constraints preclude detailed description of these results for all five crops. We highlight our findings for corn and consign the remaining results to the SI. Figure 1 shows corn's meteorological yield response functions (panel A) and the changes in exposure to weather conditions experienced by an average county in our three scenarios circa 2050 and 2100 (panels B and C). Yields decline precipitously with extreme temperature (Schlenker and Roberts 2009, Burke and Emerick 2015), but stratification of our responses by growth phase highlights the large impact in the first half of the growing season (Ortiz-Bobea 2013)—each additional 3 h period below 15 °C increases yields by as much as 0.005% relative to their conditional mean, but similar exposure above 40 °C triggers a reduction of more than 0.01%. Over the second half of the growing season, temperatures cause slight yield declines below the latter threshold but a marked increase above (as much as 0.01% per 3 h)12 . A key point of divergence with prior results is our finding that yields increase strongly and approximately linearly with precipitation, with trace amounts associated with slight declines but 3 h extreme exposures (>15 mm) increasing yields by up to 0.01% (0.015%) in the early (late) sub-periods. The instantaneous soil moisture response exhibits a generalized inverse U shape with an apex at the conditional mean exposure, a very slight negative influence over most of its range and sharply negative impact at the upper extreme (>35 kg m−2), with reduction of up to 0.004% (0.008%) in the early (late) sub-periods. Other crops' responses share many of these characteristics (figures S3–S6)13 .
Figure 1. Corn empirical yield response functions (A) and the change in the distributions of average county temperature, precipitation and soil moisture exposure circa year 2050 (B) and 2100 (C), over growing season sub-periods. Gaps in splines correspond to omitted modal intervals. Histograms show the differences in the distributions of exposure between the no-policy reference scenario and the current climate, and between the 4.5 W m−2 and 3.7 W m−2 GHG mitigation scenarios and the reference case. The vertical axes of the differenced exposure distributions have non-linear scales to better illustrate the shifts in meteorology due to climate change.
Download figure:
Standard image High-resolution imageIn panels B and C, movements in the average distribution of exposure due to unmitigated climate change shifts the relative weights on different spline segments. The larger the increases in exposure within intervals associated with negative semi-elasticities (the pink bars), the greater the downward pressure on yield. Throughout the growing season temperature increases shift exposure out of low-temperature intervals into high-temperature intervals, negatively affecting yields, particularly in early stages of crop growth. Climate change is also associated with increases in both rainfall and soil moisture that shift exposure from low to high ranges of these variables, which is generally beneficial. But where the latter intervals are associated with negative marginal effects, the sign of the yield impacts is negative as well.
GHG mitigation's broad influence is to partially reverse these shifts in probability mass (the green and blue bars). Across meteorological variables, the most common pattern is for the bars indicating the policy scenarios to be of smaller magnitude but mostly opposite sign to those corresponding to the reference case. Crucially, such reversals are not always beneficial. In the reference scenario, exposure to low precipitation declines in both halves of the growing season circa 2100, and, relative to this outcome, GHG emission mitigation increases the average frequency of such dry episodes, with adverse late-season yield impacts. Similarly, increases in large precipitation events under the reference scenario improve yields, but mitigation reduces these increases, curtailing this particular benefit from unmitigated climate change. Finally, as indicated in the SI (figure S2), a pervasive consequence of mitigation is the reduction in the CFE and its attendant yield benefits.
3.2. Projected changes in crop yields and production
Conditions within individual counties can diverge markedly from the aforementioned average changes in exposure. Maps of projected yield changes in figure 2 indicate the spatial patterns of the threat to the five major crops posed by unmitigated climate change, as well as the substantial threat reduction due to moderate mitigation. Panel A shows the counties where crop production is concentrated, while panels B and C illustrate the percentage changes in yields calculated using equation (3). All crops experience both beneficial and adverse effects, depending on the region. In the reference scenario, wheat yields increase in the northwest and decline in the south central and southwest regions circa 2050, a pattern that intensifies markedly toward century's end. For the remaining crops, the patterns of impact tend to follow the north-south temperature gradient. Soybean and corn yields suffer pronounced negative impacts in the South and the Mississippi River valley that first lessen before turning positive with proximity to Canada. For cotton, the largest adverse effects are dispersed across the crescent of southernmost counties, while for sorghum negative impacts are concentrated in the southwest. The reductions in changes in climatic variables as a consequence of mitigation policies attenuate the amplitude of beneficial as well as adverse yield shocks. Even a 4.5 W m−2 GHG stabilization policy limits impacts to ±10% from baseline levels in the majority of cultivated counties. Results for the stringent 3.7 W m−2 scenario (not shown) are similar but further accentuated.
Figure 2. Geographic distributions of 1980–2010 crop production (A), and % change in yields of five major US crops relative to current climate circa years 2050 and 2100, under a no-policy reference scenario (B) and a moderate GHG mitigation scenario (C).
Download figure:
Standard image High-resolution imageHowever, the risk to agricultural supply arises out of the spatial intersection of yield shocks and patterns of crop production in future decades when climatic changes occur. Although the latter will likely differ from today, given the challenges that attend prediction of agriculture's future geographic distribution (see, e.g., Ortiz-Bobea and Just 2012, Iizumi and Ramankutty 2015), we follow the empirical literature in assuming that irrigated and rainfed crop cultivation will continue to follow the current geographic pattern in panel A. We quantify the implications at the scale of US climate regions in table 1.
Table 1. Changes in crop production (%) relative to current climate in the no-policy reference and GHG mitigation scenarios, circa years 2050 and 2100: by US climate regions.
![]() |
Note: Square braces: changes in output from the reference scenario; shaded cells: losses relative to the current period (in cells with square braces, relative to reference scenario); bold: major producing regions.
Under reference warming, circa 2050, there are increases in yields of corn and sorghum, declines in wheat and soybeans, and mixed impacts on cotton in the regions where production of these crops is concentrated. Climate impacts that manifest in one or two regions at mid-century often spread geographically by 2100, with production in regions that suffer early adverse impacts (e.g., the southeast, and, to a lesser extent, south central regions) often experiencing further declines. Mitigation often only softens the blow in regions with the largest percentage losses, and, paradoxically, changes in weather patterns associated with stringent emission reductions may have smaller ameliorative impacts (see south, southeast and central sorghum and corn), likely due to the interplay between the impact of changes in meteorological variables and the CFE. Conversely, climate change improves yields in the cooler northeast, northwest, and, less reliably, north central areas around mid-century, with declines in the pool of regions experiencing beneficial weather as warming proceeds. Mitigation offsets output declines from regions experiencing losses at the cost of curtailing gains to those benefiting from climate change. However, rarely does mitigation transform gains under the reference into outright losses: more commonly regions that gain experience smaller benefits.
Our projected percentage changes in aggregate yield understate those of prior studies, although a clean comparison is elusive because of differences in the scenarios of future warming and their meteorological consequences as elaborated by ESMs (see table S3). Our inclusion of the CFE accounts for some of this divergence. Re-running our projections without the CFE14 results in yield losses that are 20% larger for wheat and between 2 and 4 times as large for soybeans and cotton, and yield gains for corn and sorghum that are 10%–15% smaller (table S2). More consequential are our findings of countervailing effects of extreme heat on corn yields (adverse early, beneficial late), and the importance of precipitation generally. The latter is particularly important given that our estimates assume no water stress, and therefore no impact of projected changes in precipitation or soil moisture, on the irrigated fraction of the crop in each county. Relative to the customary method of applying a single fitted yield response function everywhere, our approach reduces yield losses (gains) in regions experiencing precipitation and soil moisture declines (increases).
3.3. Economic costs of agricultural impacts and benefits of GHG mitigation
The implications for aggregate climate damage costs and GHG mitigation benefits are summarized in table 2. Costs (negative entries)and benefits (positive entries) are assessed by establishing two baselines from which to compute the absolute changes in output that correspond to US-wide percentage changes. Panel A, which collapses table 1, demonstrates that, under reference warming, adverse national average yield impacts are dominated by wheat, soybeans and, toward century's end, cotton. Conversely, corn and sorghum experience large increases in national average yield. Panel B shows the result of a comparative static calculation of the associated costs and benefits of climate change if crop production and prices remain at today's levels. Output losses (i) follow the reference pattern in Panel A, but negative impacts on wheat are lessened and on soybeans are reversed by mitigation's reduction of warming to beneficial levels. The corresponding economic impacts (ii) are expressed as changes in revenue, calculated by multiplying the quantity shocks by each crop's 1981–2010 average real farmgate price. Broadly echoing Deschênes and Greenstone's (2007) findings, climate change has a net beneficial impact which is modest at mid-century ($1 B) but becomes negligibly small by 2100. Relative to the reference, moderate mitigation generates additional annual benefits of $1 B ($2 B) circa 2050 (2100), while stringent mitigation's benefits are smaller: $0.6 B ($0.8 B) per year by 2050 (2100). Panel B's estimates incorporate future increases in production, and associated price changes, as simulated by MIT-EPPA's CIRA runs. Impacts are identical in sign, but expansions in crop output and revenue increase the magnitude of costs and benefits15 . Net annual benefits under reference warming are still small ($3 B circa 2050, $1B by 2100), while moderate (stringent) mitigation gives rise to modest additional annual benefits of $3 B ($1 B) by 2050 and $17 B ($7 B) by 2100.
Table 2. Aggregate annual changes in crop yields, production and associated gross costs and benefits relative to current climate in the no-policy reference scenario, and aggregate avoided changes in crop yields and associated costs and benefits under GHG mitigation scenarios, circa years 2050 and 2100. (A) aggregate yield changes; (B) prices and quantities in current agricultural system; (C) prices and quantities scaled according to future growth simulated by the MIT-EPPA model's CIRA simulations.
2036–2055 | 2086–2115 | |||||
---|---|---|---|---|---|---|
Ref | 4.5 W m−2 | 3.7 W m−2 | Ref | 4.5 W m−2 | 3.7 W m−2 | |
(A) Average change in yield relative to current climate (%) | ||||||
Wheat | −3.5 | −0.3 | −0.8 | −7.9 | −1.3 | −1.9 |
Soybeans | −1.6 | 3.5 | 1.8 | −7.8 | 1.4 | 0.3 |
Sorghum | 6.7 | 3.0 | 2.6 | 18.1 | 5.3 | 1.7 |
Cotton | 2.2 | 3.6 | 2.6 | −3.5 | 3.2 | 1.5 |
Corn | 6.4 | 5.3 | 4.8 | 10.4 | 6.6 | 3.8 |
(B) Current agricultural system | ||||||
(i) Average change in production relative to current climate (106 tons) | ||||||
Wheat | −2.1 | −0.2 | −0.5 | −4.9 | −0.8 | −1.2 |
Soybeans | −1.1 | 2.3 | 1.2 | −5.2 | 1.0 | 0.2 |
Sorghum | 1.1 | 0.5 | 0.4 | 2.9 | 0.9 | 0.3 |
Cotton | 0.1 | 0.1 | 0.1 | −0.1 | 0.1 | 0.1 |
Corn | 14.9 | 12.3 | 11.0 | 24.1 | 15.2 | 8.7 |
(ii) Impact gross cost (negative) or benefit (positive) in reference scenario; mitigation net benefit (positive) or cost (negative) in policy scenarios (2010$ M) | ||||||
Wheat | −388 | 360 | 297 | −887 | 741 | 673 |
Soybeans | −336 | 1050 | 698 | −1608 | 1904 | 1664 |
Sorghum | 106 | −58 | −65 | 288 | −204 | −261 |
Cotton | 138 | 85 | 24 | −222 | 424 | 313 |
Corn | 1503 | −258 | −390 | 2434 | −895 | −1553 |
Total | 1024 | 1179 | 563 | 5 | 1971 | 836 |
(C) Projected future agricultural system | ||||||
(i) Average change in production (106 tons) | ||||||
Wheat | −5.5 | −0.4 | −1.1 | −32.4 | −4.6 | −5.8 |
Soybeans | −2.8 | 5.5 | 2.6 | −34.3 | 6.3 | 1.2 |
Sorghum | 2.8 | 1.2 | 0.9 | 19.5 | 5.7 | 1.8 |
Cotton | 0.2 | 0.3 | 0.2 | −0.3 | 0.3 | 0.1 |
Corn | 38.0 | 29.4 | 24.7 | 159.1 | 100.6 | 57.6 |
(ii) Impact gross cost (negative) or benefit (positive) in reference scenario; mitigation net benefit (positive) or cost (negative) in policy scenarios (2010$ M) | ||||||
Wheat | −1232 | 1170 | 1011 | −8395 | 7368 | 7475 |
Soybeans | −1065 | 3251 | 2143 | −15 230 | 18 442 | 17 125 |
Sorghum | 336 | −199 | −231 | 2726 | −1973 | −2682 |
Cotton | 439 | 229 | 12 | −811 | 1539 | 1199 |
Corn | 4769 | −1093 | −1730 | 23 048 | −8666 | −15 985 |
Total | 3247 | 3358 | 1205 | 1338 | 16710 | 7132 |
4. Discussion and conclusions
By combining empirical analysis with integrated economic and climate projections, we demonstrate that climate change effects on US crop yields are likely to be slight around mid-century but substantially costly near century's end, with regions where climates are already warm suffering losses but cooler regions enjoying gains, and declines in production concentrated in soybeans, cotton and wheat that are partially offset by increases in output of corn and sorghum. Reductions in radiative forcing from GHG mitigation generally offset output declines from regions and crops that experience losses, but at the cost of curtailing gains to those that benefit from climate change.
As summarized in figure 3, our results suggest that the overall effect of mitigation policies on agricultural revenues will be positive, but the magnitude is sensitive to the beneficial impacts of CO2 fertilization. Without the CFE, the impact of unmitigated climate change flips sign, incurring annual net costs of $3 B ($18 B) circa 2050 (2100). This amplifies the positive effect of emission reductions, increasing the benefits of moderate mitigation by $1.4 B ($10 B) circa 2050 (2100), and of stringent mitigation by $1.1 B ($13 B) circa 2050 (2100). These estimates, which should be considered upper bounds on the costs of climate change impact and corresponding emission reduction benefits, highlight the critical importance of assumptions regarding the CFE. They are also somewhat larger than, but in the same general range as, climate change damages generated by prior studies (see table S2), though simple comparisons of total dollar values are not appropriate given the use of different impact endpoints (land values, agricultural profits, non-monetized yields, or affected crops) and climate change projections, as well as the lack of accounting for the CFE. As a case in point, the study most closely related to ours—Beach et al (2015)—employs a crop model forced by the CIRA IGSM-CAM simulations to construct gross-of-CFE changes in corn, soybean and wheat yields and which are then applied as exogenous shocks in a partial equilibrium simulation of the US agriculture and forestry sector. Yield impacts are mostly positive in the reference scenario and become more beneficial with stringent mitigation, over 2015–2100 increasing cumulative agricultural surplus by (2005) $45 B—or an average annual mitigation benefit of $0.5 B.
Figure 3. Annual costs (negative entries) and benefits (positive entries) of climate change impacts and mitigation on US agriculture with and without CO2 fertilization, circa 2050 and 2100. (A) Impacts by crop (total shown at bottom). (B) Costs and benefits of 4.5 W m−2 and 3.7 W m−2 stabilization policies by crop (total shown at bottom).
Download figure:
Standard image High-resolution imageOur analysis represents an advance over current approaches to quantifying the costs and benefits of climate change. We use projections of the future state of the agricultural economy using CGE model results whose simulated growth rates of agricultural output and prices are consistent with the economic expansion, general equilibrium price and quantity effects of mitigation policies, and concomitant GHG emissions, radiative forcing and meteorological changes that determine the shocks to crop yields in the decades in which these impacts occur. By contrast, empirical studies' comparative statics valuation of impacts as changes in agricultural revenues or profits under current production and prices can understate costs (see table 2). Modeling studies that simply impose GGCM-simulated yield changes onto economic models risk being inconsistent with the future economic conditions, GHG emissions and the climatic forcing of yield shocks that we argue is essential to consistent estimation of costs and benefits. But the critical feature of such model-based economic consequence analyses is the additional uncertainty they introduce by simulating the moderating effects of adaptation via market-mediated price and quantity adjustments (see Beach et al 2015). Although adaptation will almost surely occur, its associated indirect economic costs and benefits are poorly characterized and difficult to estimate, yet require accurate quantification to avoid potential double-counting when estimating the net benefit of mitigation. Our deliberately conservative approach is therefore to exclude the effects of future adaptation from our cost-benefit calculations. Instead we value the impact of climate change under the economic conditions likely to prevail at the instant such a shock occurs, before producers and consumers have an opportunity to react (Fisher-Vanden et al 2013).
Nevertheless, several caveats to our analysis remain. Our narrow focus on well-documented impact pathways omits myriad indirect climate-related changes in crops' growing environment (e.g., ozone concentrations, diseases, pathogens and weeds) on which the literature provides less guidance regarding yield responses. Space constraints preclude a full uncertainty analysis of the underlying economic assumptions in the MIT EPPA model and the climate system response, and particularly the CFE's yield benefits, which were difficult to bound (see SI). The dependence of yield changes on the assumption of perfect water application in currently irrigated areas highlights the sensitivity of our cost-benefit projections to the availability of water resources sufficient for irrigation as crop production expands out to century's end. The latter, while driven by shifting precipitation patterns, requires hydrological analysis (e.g., future water infrastructure and efficiency assumptions, changes in runoff and discharge, competition with growing municipal and industrial demands, groundwater resource development and depletion) to determine how, and where, it might influence our results. Finally, the CGE model that we use resolves future changes in aggregate agricultural activity, not individual crops. Research is ongoing to address these issues.
More broadly, our results illustrate the potential of reduced-form empirical analysis as an alternative to GGCMs in evaluating climate change impacts on agriculture (Nelson et al 2014, Rosenzweig et al 2014). Although crop models incorporate both detailed process-based understanding of crop physiology and the ameliorating effects of a plethora of management options, concerns regarding their accuracy in capturing crop yields' responses to meteorological change (Hertel and Lobell 2014) have been slow to prompt extensive testing, especially at the geographic scales examined here16 . Our methodology can usefully be applied to model the relationships between GGCM simulated yields and their climatic drivers, and thereby facilitate head-to-head comparisons that can lead to more robust estimates of impact response, future yield shocks, and associated economic costs and benefits.
Acknowledgments
ISW, AS and AM gratefully acknowledge support from NSF (grant nos. EAR-1038907 and GEO-1240507), and US Department of Energy Office of Science (BER) (grant no. DE-SC005171). EM gratefully acknowledges support from the US Environmental Protection Agency's Climate Change Division, under Cooperative Agreement #XA-83600001 and from the US Department of Energy, Office of Biological and Environmental Research, under grant DEFG02-94ER61937.
Footnotes
- 5
These are passive adaptations mediated by relative price changes (see Sue Wing and Fisher-Vanden 2013).
- 6
A key example is soil amendments. With agricultural profits, analogous decisions involve inventory adjustments (Deschênes and Greenstone 2012).
- 7
See Nickell (1985). Prior research employing ECMs to understand climate change impacts on agriculture (e.g., Blanc 2012) has not to our knowledge sought to explicitly partition yield variance into the effects of weather versus climate. For a general application of ECMs to agricultural supply response, see Hallam and Zanoli (1993).
- 8
Absent specific indicators of technological advance (e.g. patent stocks), productivity improvements are customarily modeled using a time trend.
- 9
Comparing the future climate simulated by an ESM against current climate simulated by the same model (as opposed to observations) is a way of minimizing the impact of potential bias in ESM projections.
- 10
If
the shift in the mean climatic exposure reduces crop productivity, and increases it otherwise.
- 11
- 12
The positive late response to high temperature is not the result of outlying observations. Historically, corn has regularly been exposed to this kind of heat, albeit in tiny amounts. In 23% of our 136 000 county × year observations, corn was exposure to one or more 3 h periods with
in the second half of the growing season, covering 2307 out of 2842 counties and all the years of our sample. Using simpler empirical models, Blanc and Sultan (2015: figures C1–C4) uncover similar beneficial yield responses to late extreme heat in the results of ISI-MIP GGCMs. Work remains to be done to understand the mechanisms responsible for this phenomenon, both in GGCMs and the field.
- 13
We find negative and strongly nonlinear temperature sensitivity of sorghum, soybeans, and, to a lesser extent wheat, especially in the first half of the growing season (see Tack et al 2015). For the most part, precipitation's long-run effect is positive or statistically insignificant over most of its range (especially in the second half of the growing period). Exceptions are the negative and significant impacts of early extreme precipitation on soybeans and wheat. Long-run soil moisture responses peak apex at the modal exposure, suggesting detrimental yield impacts of soil waterlogging as well as drying, with the exception of cotton early in the growing season. These results are generally in line with recent empirical findings. A shortcoming of our model is its omission of freezing temperatures and the associated negative response of wheat yields, whose amelioration in a warming climate provides an offsetting beneficial effect (Tack et al 2015).
- 14
This is achieved simply by setting
in equation (S9).
- 15
Relative to today, composite agricultural output is projected to increase by a factor of 2.5 by 2050 and 6.5 by 2100, with real composite agricultural prices increasing by 25% and 44%, respectively.
- 16