Abstract
Skin diseases are a major and worrying problem in societies due to their physical and psychological effects on patients. Detecting skin diseases at an early stage has an important role in treatment. The process of diagnosing and treating skin injury is related to the skill and experience of the specialist doctor. The diagnostic process must be accurate and timely. Recently, artificial intelligence science has been used in the field of diagnosing skin diseases through the use of machine learning algorithms and the exploitation of the vast amount of data available in health centers and hospitals. In this paper, quite many previous studies related to methods of classification of skin diseases based on the principle of machine learning were collected. In a group of previous studies, the researchers used some systems, mechanisms, and algorithms. Several systems have been successful in classifying skin diseases and achieving varying diagnostic accuracy. Various systems have relied on methods of image processing and feature extraction that help predict and detect disease type. There are other systems designed to identify specific types of skin disease through clinical features and features obtained from tissue analyzes after a skin biopsy of the affected area. This survey shows that the diagnostic accuracy in image processing methods was relatively uneven, ranged between (50% to 100%). As for the methods of treating tissue features, the accuracy was of an excellent level of 94% or more. The results provide an overview of the actual relevant studies found in the literature and highlight most of which research gaps have emerged.
Export citation and abstract BibTeX RIS
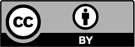
Content from this work may be used under the terms of the Creative Commons Attribution 3.0 licence. Any further distribution of this work must maintain attribution to the author(s) and the title of the work, journal citation and DOI.