Abstract
Higher education institutions aim to forecast student success which is an important research subject. Forecasting student success can enable teachers to prevent students from dropping out before final examinations, identify those who need additional help and boost institution ranking and prestige. Machine learning techniques in educational data mining aim to develop a model for discovering meaningful hidden patterns and exploring useful information from educational settings. The key traditional characteristics of students (demographic, academic background and behavioural features) are the main essential factors that can represent the training dataset for supervised machine learning algorithms. In this study, we compared the performances of several supervised machine learning algorithms, such as Decision Tree, Naïve Bayes, Logistic Regression, Support Vector Machine, K-Nearest Neighbour, Sequential Minimal Optimisation and Neural Network. We trained a model by using datasets provided by courses in the bachelor study programmes of the College of Computer Science and Information Technology, University of Basra, for academic years 2017–2018 and 2018–2019 to predict student performance on final examinations. Results indicated that logistic regression classifier is the most accurate in predicting the exact final grades of students (68.7% for passed and 88.8% for failed).
Export citation and abstract BibTeX RIS
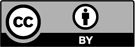
Content from this work may be used under the terms of the Creative Commons Attribution 3.0 licence. Any further distribution of this work must maintain attribution to the author(s) and the title of the work, journal citation and DOI.