- 1Department of Thoracic Surgery, Shanghai Chest Hospital, Shanghai Jiao Tong University, Shanghai, China
- 2Department of Thoracic Surgery, Shanghai Pulmonary Hospital, Tongji University School of Medicine, Shanghai, China
- 3Department of Cardiology, Shanghai Chest Hospital, Shanghai Jiao Tong University, Shanghai, China
- 4Department of Colorectal and Anal Surgery, Xinhua Hospital, Shanghai Jiao Tong University School of Medicine, Shanghai, China
- 5Shanghai Colorectal Cancer Research Center, Shanghai, China
- 6Department of Nuclear Medicine, Ren Ji Hospital, Shanghai Jiao Tong University School of Medicine, Shanghai, China
- 7Bio-Med Big Data Center, CAS Key Laboratory of Computational Biology, CAS-MPG Partner Institute for Computational Biology, Shanghai Institute of Nutrition and Health, Chinese Academy of Sciences, Shanghai, China
Acute and chronic inflammation often leads to fibrosis, which is also the common and final pathological outcome of chronic inflammatory diseases. To explore the common genes and pathogenic pathways among different fibrotic diseases, we collected all the reported genes of the eight fibrotic diseases: eye fibrosis, heart fibrosis, hepatic fibrosis, intestinal fibrosis, lung fibrosis, pancreas fibrosis, renal fibrosis, and skin fibrosis. We calculated the Kyoto Encyclopedia of Genes and Genomes (KEGG) and Gene Ontology (GO) enrichment scores of all fibrotic disease genes. Each gene was encoded using KEGG and GO enrichment scores, which reflected how much a gene can affect this function. For each fibrotic disease, by comparing the KEGG and GO enrichment scores between reported disease genes and other genes using the Monte Carlo feature selection (MCFS) method, the key KEGG and GO features were identified. We compared the gene overlaps among eight fibrotic diseases and connective tissue growth factor (CTGF) was finally identified as the common key molecule. The key KEGG and GO features of the eight fibrotic diseases were all screened by MCFS method. Moreover, we interestingly found overlaps of pathways between renal fibrosis and skin fibrosis, such as GO:1901890-positive regulation of cell junction assembly, as well as common regulatory genes, such as CTGF, which is the key molecule regulating fibrogenesis. We hope to offer a new insight into the cellular and molecular mechanisms underlying fibrosis and therefore help leading to the development of new drugs, which specifically delay or even improve the symptoms of fibrosis.
Introduction
Acute and chronic inflammation often leads to fibrosis, which is also the common and final pathological outcome of chronic inflammatory diseases (Rockey et al., 2015). Fibrosis is defined as overaccumulation of fibrous connective tissue in and around the tissues with inflammation or damage, triggering irreversible scar formation. The clinical manifestations are renal disease, idiopathic pulmonary fibrosis (IPF), heart failure, end-stage liver diseases, and so on (Bataller and Brenner, 2005). Besides, fibrosis can also be observed in many chronic autoimmune diseases, such as rheumatoid arthritis, scleroderma, myelofibrosis, and Crohn disease. But the common characteristics of these fibrosis diseases were still unknown.
Fibrosis can affect chronic graft rejection, tumor invasion and metastasis, and the pathogenesis of many progressive myopathies. With regard to chronic graft rejection, fibrosis is one of the most common symptoms in chronic graft rejection. For example, liver transplantation in children has a 20-year survival of more than 80% at present, but the long-term results of these grafts still remain uncertain. Biopsies after liver transplantation show idiopathic post-transplant hepatitis and graft fibrosis occur even in children with good graft function (Kelly et al., 2016). As for tumor invasion and metastasis, carcinoma-associated fibroblasts are able to enhance tumor cells migration and invasion via activating the process of specific pathways. For example, as lung cancer maintains the leading cause of cancer-related deaths, IPF has been demonstrated that it increases the risk of lung cancer development by 7–20%, and there are multiple common molecular processes that associated IPF with lung cancer, such as epithelial–mesenchymal transition (EMT), endoplasmic reticulum stress, and abnormal expression of growth factors (Gu et al., 2018, 2020a,b; Ballester et al., 2019; Jiao and Yang, 2020). In the tissue of myopathies, there is prominent endomysial fibrosis, but little or no inflammation.
The fact that fibrotic changes are commonly observed in different diseases of diverse organ systems suggests common pathogenic pathways (Rockey et al., 2015). The wound healing in the fibrotic tissue is regulated by complex processes within different cells, and therefore some specific molecular pathways are activated. For example, in IPF, the fibrosis starts from the lung periphery to the lung center, finally causing respiratory failure. The underlying mechanisms of IPF were proven that elevated mechanical tension activates a transforming growth factor β (TGF-β) signaling loop in alveolar stem cells (AT2).
In this study, we proposed a new computational method incorporating feature engineering and feature selection algorithms to explore the common controlling genes and corresponding pathways among eight different organs’ fibrosis. The key genes and pathways were revealed, and the cross-talks between diseases were investigated. These results were helpful for understanding the molecular mechanisms of fibrosis diseases and finding new therapeutic indications of existing drugs, i.e., drug repositioning.
Materials and Methods
The Reported Genes of the Eight Fibrotic Diseases
All the genes of the related eight fibrotic diseases (eye fibrosis, heart fibrosis, hepatic fibrosis, intestinal fibrosis, lung fibrosis, pancreas fibrosis, renal fibrosis, and skin fibrosis) extracted from published researches are listed in Supplementary Table 1. In Supplementary Table 1, “1” refers to the genes associated with the specific fibrotic diseases, whereas “0” means the genes have no relationship with the specific fibrotic diseases. We compared the reported genes of the eight fibrotic diseases using R package SuperExactTest,1 which has the function of identification of sets of objects with shared features, which is a common operation in all disciplines. Analysis of intersections among multiple sets is fundamental for in-depth understanding of their complex relationships. This package implements a theoretical framework for efficient computation of statistical distributions of multiset intersections based on combinatorial theory and provides multiple scalable techniques for visualizing the intersection statistics (Wang et al., 2015). There were 954 genes that were associated with at least one of the eight fibrotic diseases. In each fibrotic disease, the numbers of reported genes are listed in Table 1.
Encoding the Fibrotic Disease Genes With KEGG and GO Features
We calculated the KEGG (Kyoto Encyclopedia of Genes and Genomes) and GO (Gene Ontology) enrichment scores of all fibrotic disease genes. For each specific fibrotic disease, the reported genes of this disease were considered as positive samples, and the other genes were considered as negative samples. The KEGG and GO enrichment scores (Shi et al., 2018; Gu et al., 2020c) were used as features to encode genes and characterize their functions.
The KEGG and GO enrichment scores were the functional profiles of a gene. To be more specific, we enriched the neighbors of genes in STRING network (version 11.02) (Szklarczyk et al., 2018) on to KEGG pathway and GO terms. Given a gene g, let S(g) be a gene set consisting of genes that have functional associations with gene g in STRING network (Szklarczyk et al., 2018). Given a gene g and a GO termGOj, the GO enrichment score was defined as the −log10 of the hypergeometric test P-value (Chen et al., 2016) of the gene set S(g) and the GO term GOj, which can be computed as follows:
where N was the total number of human genes in STRING database, M and n were the number of genes annotated to GOj and the number of genes in S(g), respectively, and m was the number of genes in S(g) that were annotated to GOj.
Similarly, the KEGG enrichment scores can be calculated by replacing the GO terms with KEGG pathways. The higher enrichment score meant this gene can affect this biological function. In total, there were 22,130 features (324 KEGG enrichment scores and 21,806 GO enrichment scores). The GO (2019-Apr24) annotations were downloaded from ftp://ftp.geneontology.org/, and the KEGG (Release 91.0) annotations were extracted from https://www.kegg.jp/ using R/Bioconductor package KEGGREST3 on July 1, 2019.
Identifying the Key KEGG and GO Features for Each Fibrotic Disease
The Monte Carlo feature selection (MCFS) method (Draminski et al., 2008) was applied to rank all the KEGG and GO features based on their importance in classification. It has been widely used and showed great power in identify robust key features for complex biological problems (Pan et al., 2018, 2020; Chen et al., 2020; Li et al., 2020a; Ren et al., 2020). As a supervised feature selection method, the MCFS method was based on tree classifiers. It constructed a series of tree classifiers on a series of subsets randomly selected from the whole dataset. By considering how much a feature contributed in these tree classifiers, the importance of this feature was calculated. By comparing with its importance calculated on permuted datasets, its significance can be calculated. As it ensembled a series of trees, the results were robust and trustworthy (Pan et al., 2019a,b,c, 2020; Li et al., 2020b).
For each fibrotic disease, the KEGG and GO enrichment features of the positive samples (the reported genes of this disease) and the negative samples (the other genes) were compared, and the relative importance (RI) of each feature was evaluated using MCFS algorithm. The significant KEGG and GO features were selected and analyzed. Software dmlab downloaded from http://www.ipipan.eu/staff/m.draminski/mcfs.html was used to apply the MCFS algorithm, and the default parameters were used.
Results
The Overlapped Genes of the Eight Fibrotic Diseases
We compared the reported genes of the eight fibrotic diseases using R package SuperExactTest (see text footnote 1) (Wang et al., 2015). The results are shown in Supplementary Table 2. In Supplementary Table 2, degree 1 represents the original gene lists of the eight fibrotic diseases, degree 2 means the gene overlaps between any two groups, and degree 3 shows the gene overlaps among any three groups. By that analogy, degree 8 means the gene overlaps among all the eight groups. The data visualization is illustrated in Figure 1. The numbers of overlapped genes are listed over the histogram, and the darkness of the color represents how significant the overlap was. The connective tissue growth factor (CTGF) was finally identified as the common key molecule in the process of fibrosis.
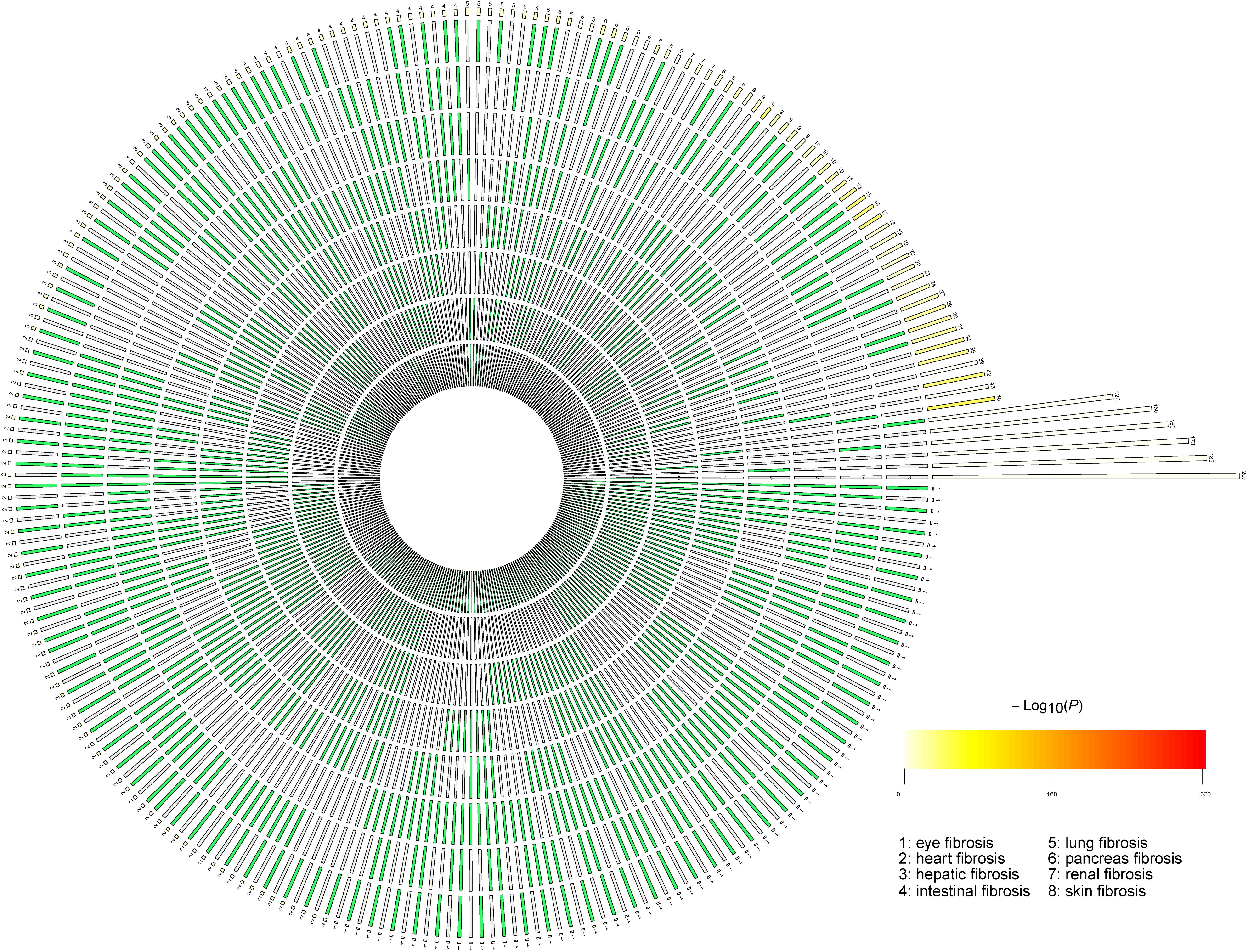
Figure 1. The number of overlapped genes among the eight fibrotic diseases. A circular plot illustrating all possible intersections and the corresponding statistics. The eight circles from inside to outside represent the eight fibrotic diseases (1, eye fibrosis; 2, heart fibrosis; 3, hepatic fibrosis; 4, intestinal fibrosis; 5, lung fibrosis; 6, pancreas fibrosis; 7, renal fibrosis; and 8, skin fibrosis), respectively. The height of the bars in the outer layer is proportional to the intersection sizes, as indicated by the numbers on the top of the bars. The color intensity of the bars represents the P-value significance of the intersections.
The Key KEGG and GO Features of the Eight Fibrotic Diseases
The key KEGG and GO features of the eight fibrotic diseases were screened by MCFS method. As shown in Supplementary Table 3, it means that if a gene could influence a specific function, it may cause a certain fibrotic disease.
As for eye fibrosis, the top three GO terms are GO:0033693 neurofilament bundle assembly, GO:1904530 negative regulation of actin filament binding, and GO:0031113 regulation of microtubule polymerization, respectively. GO:0033693 is associated with neurofilament bundle assembly, which means the assembly of neurofilaments into bundles, in which the filaments are longitudinally oriented, with numerous cross-bridges between them. GO:1904530 is related to negative regulation of actin filament binding, which means reducing physiological activities of actin filament binding. GO:0031113 is connected with the normal physiological activities of microtubule polymerization. Corneal fibrosis is the major type of eye fibrosis. Vimentin, a major structural type III intermediate filament, is a required component of keratocyte activation and differentiation corneal fibrosis, which often accelerates the process of fibrosis (Das et al., 2014).
As for heart fibrosis, the top three GO terms are GO:0032971 regulation of muscle filament sliding, GO:0070296 sarcoplasmic reticulum calcium ion transport, and GO:1990584 troponin complex, respectively. GO:0032971 is in connection with the process that regulates the frequency, rate, or extent of muscle filament sliding. GO:0070296 determines the movement of calcium ions, and GO:1990584 is associated with the cardiac troponin complex and influences muscle contraction. Therefore, muscle filament sliding and calcium ions have been proven to play important roles in the process of hypertrophic cardiomyopathy and heart fibrosis (Huang et al., 2014).
As for hepatic fibrosis, the top three GO terms are GO:0047747 cholate-CoA ligase activity, GO:0008508 bile acid:sodium symporter activity, and GO:0051264 mono-olein transacylation activity, respectively. GO:0047747 affects the activity of cholate-CoA ligase, which catalyzes some reactions in liver. GO:0008508 is related with bile acid and sodium ion transport. GO:0051264 is connected with mono-olein metabolism. Serum bile acids and total cholesterol (TC) are closely related to liver cirrhosis; the potential diagnostic value of total bile acid-to-cholesterol ratio (TBA/TC) for liver fibrosis has been proven (Yan et al., 2020).
As for intestinal fibrosis, the top three GO terms are GO:0032500 muramyl dipeptide binding, GO:0032498 detection of muramyl dipeptide, and GO:0045076 regulation of interleukin 2 (IL-2) biosynthetic process, respectively. GO:0032500 is related with muramyl dipeptide binding, whereas GO:0032498 is associated with detection of muramyl dipeptide. GO:0045076 regulates the process of IL-2 in fibrosis, which has also been proven in patients with cirrhosis and ascitic fluid (Juanola et al., 2016).
As for lung fibrosis, the top three GO terms are GO:0070950 regulation of neutrophil mediated killing of bacterium, GO:0070951 regulation of neutrophil mediated killing of Gram-negative bacterium, and GO:0004957 prostaglandin E receptor activity, respectively. GO:0070950 is related with regulation of neutrophil mediated killing of bacterium. GO:0070951 participates in regulation of neutrophil-mediated killing of Gram-negative bacterium. GO:0004957 means fibrogenesis via prostaglandin E receptor activity. It has been reported that neutrophil-mediated Gram-negative bacterial killing was connected with the cystic fibrosis (CF) lung (Vega-Carrascal et al., 2014).
As for pancreas fibrosis, the top three GO terms are GO:2000878-positive regulation of oligopeptide transport, GO:2000880-positive regulation of dipeptide transport, and GO:2001150-positive regulation of dipeptide transmembrane transport, respectively. All of the three are related to peptide transport. GO:2000878 is associated with positive regulation of oligopeptide transport, whereas GO:2000880 with positive regulation of dipeptide transport. GO:2001150 is related to positive regulation of dipeptide transmembrane transport. CF in the pancreas is characterized by an abnormality in cAMP-regulated chloride transport, which supports the findings of the predicted GO terms (Marino et al., 1991).
As for renal fibrosis, the top three GO terms are GO:0072015 glomerular visceral epithelial cell development, GO:0036057 slit diaphragm, and GO:0005362 low-affinity glucose:sodium symporter activity, respectively. GO:0072015 affects glomerular visceral epithelial cell development and therefore influences its formation to the mature structure. GO:0036057 associated a specialized cell–cell junction, which affects glomerular filtration. GO:0005362 is related to the transfer function of a solute. Renal fibrosis is often caused by renal glomerular sclerosis and interstitial fibrosis. Therefore, glomerular visceral epithelial cell development and formation, glomerular filtration, and transfer function act as the internal causes of renal fibrosis (Qi et al., 2020).
As for skin fibrosis, the top three GO terms are GO:0005600 collagen type XIII trimer, GO:0030936 transmembrane collagen trimer, and GO:0030316 osteoclast differentiation, respectively. GO:0005600 plays a role by collagen type XIII trimer, whereas GO:0030936 via transmembrane collagen trimer. Collagen trimer contributes to derangements in extracellular matrix (ECM) remodeling and leads to fibrosis (Madahar et al., 2018).
The Cross-Talks Between Different Fibrotic Diseases
From the key KEGG and GO features of all the eight fibrotic diseases, we interestingly found overlaps of pathways within some specific fibrotic diseases. For example, renal fibrosis and skin fibrosis jointly influence GO:1901890-positive regulation of cell junction assembly. Some researchers have demonstrated that in renal fibrosis, MG132 successfully sustained cytoskeletal assembly and tight junction, preventing EMT process via RhoA-dependent TGF-β1 pathway, whereas in systemic sclerosis, endothelial junction–associated protein plays vital importance to the pathogenicity (Kanno et al., 2017).
To explore the cross-talk between renal fibrosis and skin fibrosis, we mapped the genes of renal fibrosis, the genes of skin fibrosis, and the genes of GO:1901890-positive regulation of cell junction assembly, which was the common GO feature between renal fibrosis and skin fibrosis, onto STRING network (Figure 2). In Figure 2, genes in red refer to the overlaps between renal fibrosis and skin fibrosis, whereas the specific genes in renal fibrosis, skin fibrosis, and GO:1901890 are shown in light yellow, light blue, and pink circles, respectively. As illustrated in Figure 2, the overlapped genes between renal fibrosis and skin fibrosis included CCL2, SIRT1, KLF5, PPARG, AKT1, SHH, NOTCH, SMAD7, TGFB1, CTNNB1, MMP2, CTGF, FN1, ITGB1, PLAUR, MMP14, NOX4, and COL1A1.
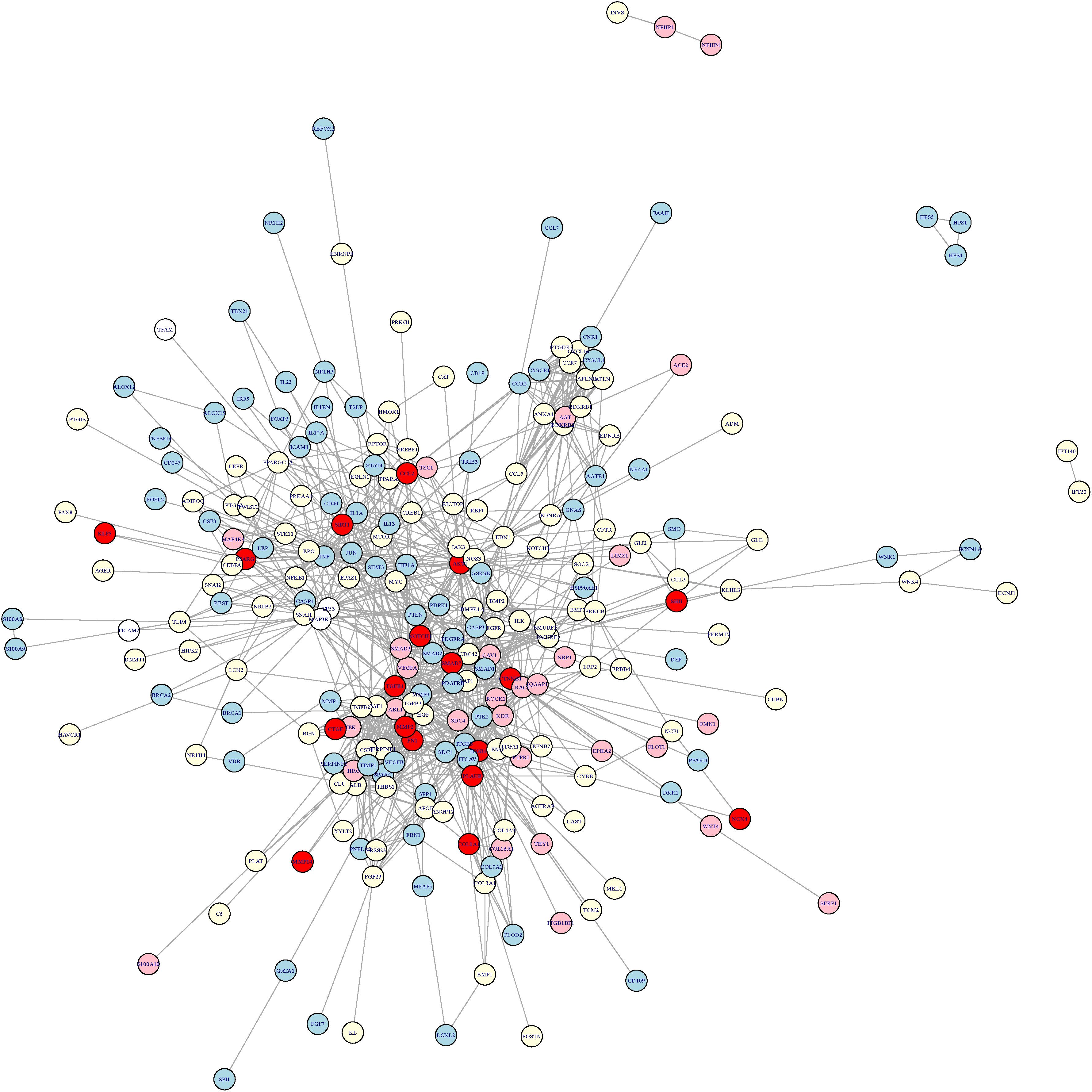
Figure 2. The cross-talk network between renal fibrosis and skin fibrosis. The genes in red refer to the overlaps between renal fibrosis and skin fibrosis, whereas the specific genes in renal fibrosis, skin fibrosis, and GO:1901890 are shown in light yellow, light blue, and pink circles, respectively. The overlapped genes between renal fibrosis and skin fibrosis included CCL2, SIRT1, KLF5, PPARG, AKT1, SHH, NOTCH, SMAD7, TGFB1, CTNNB1, MMP2, CTGF, FN1, ITGB1, PLAUR, MMP14, NOX4, and COL1A1.
Discussion
Fibrosis is a pathological characteristic of most chronic inflammatory diseases, and many deep learning methods have been developed to study human diseases (Wynn and Ramalingam, 2012; Chen Q. et al., 2019; Cheng and Ghany, 2020; Feng et al., 2020; Lan et al., 2020; Zhao et al., 2020). In recent years, fibrosis is recognized as a main reason of the occurrence of adverse events in many chronic inflammatory diseases. However, the underlying mechanisms in different organs are various and the generality among diverse fibrotic diseases still need to be uncovered. In this study, we applied a new computational method incorporating several machine learning algorithms to explore the common controlling genes and their corresponding pathways among eight different organs’ fibrosis.
Common Genes
In our study, CTGF was identified as the common regulatory gene in the eight kinds of fibrotic diseases by MCFS method. It has been around 30 years since the discovery of CTGF from human umbilical vein endothelial cells. In previous researches, CTGF plays an important role in diverse diseases, including cancers, neurodegenerative diseases, systemic sclerosis, kidney diseases, pancreatic diseases, and so on, which means CTGF expresses generally. Mao et al. (2019) demonstrated that megakaryocytic leukemia 1 (MKL1) mediates TGF-β–induced CTGF transcription to promote renal fibrosis. CTGF knockdown dampened TGF-β–induced profibrogenic response in renal tubular epithelial cells. In cardiac fibrosis, Tan et al. (2019) developed an the lamin gene (LMNA) dilated cardiomyopathy (DCM) mouse model and found silencing of cardiac LMNA-induced DCM with associated cardiac fibrosis and inflammation and further uncovered that Yy1 suppresses DCM and cardiac fibrosis through regulation of bmp7 and CTGF. Besides, another study also proved that in patients with rheumatic heart disease, high CTGF expression was related to enlarged left atrial diameter, atrial fibrosis, and atrial anatomical remodeling (Chen J.Q. et al., 2019). In lung fibrosis, disintegrin and metalloproteinase 17, and CTGF were found to play critical roles in fibrotic procedures and contribute to lung fibrosis (Chen et al., 2018).
With regard to the gene overlaps of pathways within some specific fibrotic diseases, we have identified some common pathways and genes within renal fibrosis and skin fibrosis. For example, in chronic renal allograft injury resulting in progressive interstitial fibrosis, early urinary CCL2 is an independent predictor for the subsequent development of interstitial fibrosis and tubular atrophy at 24 months (Ho et al., 2010). Similarly, in systemic sclerosis (skin fibrosis), the levels of circulating CCL2, CCL3, and CCL5 chemokines were significantly higher in patients with systemic sclerosis than in controls.
Common Pathways
Fibrosis and resultant organ failure result in approximately one-third of deaths worldwide (Zeisberg and Kalluri, 2013). Now that fibrosis is common and has harmful effects in almost all organs, it is a potential therapeutic target. As for predicted pathways, we have demonstrated some new pathways associated with the specific fibrotic diseases. In intestinal fibrosis, the GO term, GO:0045076, regulates the process of IL-2 in fibrosis. In patients with cirrhosis and ascitic fluid, Juanola et al. (2016) identified how the role of regulatory T cells played for compensating the inflammatory environment in cirrhosis when norfloxacin was applied, and they found norfloxacin immunomodulatory effect on IL-2 and interferon γ reduction. In lung fibrosis, GO:0070951 participates in regulation of neutrophil-mediated killing of Gram-negative bacterium. It has been reported that neutrophil-mediated Gram-negative bacterial killing was connected with the CF lung. The underlying mechanism was that galectin-9 (Gal-9) signaling through the T-cell Ig and mucin domain-containing molecule (TIM) and neutrophil TIM-3/Gal-9 signaling is perturbed in the CF airways due to proteolytic degradation of the receptor (Vega-Carrascal et al., 2014). GO:0004957 means fibrogenesis via prostaglandin E receptor activity. As Sieber et al. (2018) demonstrated, pathological features of pulmonary fibrosis include accumulation of myofibroblasts and increased ECM deposition in lung tissue; they developed a new assay with therapeutic potential in pulmonary fibrosis that acts via EP2 and EP4 receptors. In heart and renal fibrosis, angiotensin-converting enzyme inhibitors and angiotensin-receptor blockers that ameliorate cardiac and renal damage and fibrosis through many pathways such as TGF-β and SMAD pathways (Lambers Heerspink et al., 2013). In liver fibrosis, as hepatocytes process the ability of regeneration, intervention is needed for patients with hepatic fibrosis. For example, colchicine has been proven to prevent hepatic fibrosis via suppressing collagen secretion (Rockey, 2013). As the common pathways and genes were identified by our new computational method, old drugs for a specific fibrosis may be effective for another organ fibrosis.
Conclusion
In conclusion, we identified that CTGF is acted as the key molecule regulating the processes of fibrogenesis and some common pathways within different fibrotic diseases via a new computational method. We hope to offer a new insight into the cellular and molecular mechanisms underlying fibrosis and therefore help lead to the development of new drugs that specifically delay or even improve the symptoms of fibrosis.
Data Availability Statement
The original contributions presented in the study are included in the article/Supplementary Material, further inquiries can be directed to the corresponding author/s.
Author Contributions
TH, XP, and YC: conception and design and administrative support. CG, XS, and XD: collection and assembly of the data and data analysis and interpretation. All authors wrote the manuscript and approved the submitted version.
Funding
This work was supported by Strategic Priority Research Program of Chinese Academy of Sciences (XDB38050200), National Key R&D Program of China (2017YFC1201200), the National Natural Science Foundation of China (81900280 and 31701151), Shanghai Sailing Program (19YF1431600), National Key R&D Program of China (2018YFC0910403), Shanghai Municipal Science and Technology Major Project (2017SHZDZX01), and The Youth Innovation Promotion Association of Chinese Academy of Sciences (CAS) (2016245).
Conflict of Interest
The authors declare that the research was conducted in the absence of any commercial or financial relationships that could be construed as a potential conflict of interest.
Supplementary Material
The Supplementary Material for this article can be found online at: https://www.frontiersin.org/articles/10.3389/fgene.2020.627396/full#supplementary-material
Supplementary Table 1 | The reported genes of the eight fibrotic diseases.
Supplementary Table 2 | The overlapped genes of the eight fibrotic diseases.
Supplementary Table 3 | The key KEGG and GO features of the eight fibrotic diseases.
Footnotes
- ^ https://CRAN.R-project.org/package=SuperExactTest
- ^ https://string-db.org/
- ^ https://bioconductor.org/packages/KEGGREST/
References
Ballester, B., Milara, J., and Cortijo, J. (2019). Idiopathic pulmonary fibrosis and lung cancer: mechanisms and molecular targets. Int. J. Mol. Sci. 20:593. doi: 10.3390/ijms20030593
Bataller, R., and Brenner, D. A. (2005). Liver fibrosis. J. Clin. Invest. 115, 209–218. doi: 10.1172/JCI24282
Chen, H. Y., Lin, C. H., and Chen, B. C. (2018). ADAM17/EGFR-dependent ERK activation mediates thrombin-induced CTGF expression in human lung fibroblasts. Exp. Cell Res. 370, 39–45. doi: 10.1016/j.yexcr.2018.06.008
Chen, J. Q., Guo, Y. S., Chen, Q., Cheng, X. L., Xiang, G. J., Chen, M. Y., et al. (2019). TGFbeta1 and HGF regulate CTGF expression in human atrial fibroblasts and are involved in atrial remodelling in patients with rheumatic heart disease. J. Cell Mol. Med. 23, 3032–3039. doi: 10.1111/jcmm.14165
Chen, Q., Lai, D., Lan, W., Wu, X., Chen, B., Chen, Y. P., et al. (2019). ILDMSF: inferring associations between long non-coding RNA and disease based on multi-similarity fusion. IEEE/ACM Trans. Comput. Biol. Bioinform. doi: 10.1109/TCBB.2019.2936476 Online ahead of print.
Chen, L., Pan, X., Guo, W., Gan, Z., Zhang, Y.-H., Niu, Z., et al. (2020). Investigating the gene expression profiles of cells in seven embryonic stages with machine learning algorithms. Genomics 112, 2524–2534. doi: 10.1016/j.ygeno.2020.02.004
Chen, L., Zhang, Y. H., Zheng, M., Huang, T., and Cai, Y. D. (2016). Identification of compound-protein interactions through the analysis of gene ontology. KEGG enrichment for proteins and molecular fragments of compounds. Mol. Genet. Genom. 291, 2065–2079. doi: 10.1007/s00438-016-1240-x
Cheng, X., and Ghany, M. G. (2020). Hepatitis C virus—from Discovery to the Nobel Prize. Cambridge, MA: Cell Press.
Das, S. K., Gupta, I., Cho, Y. K., Zhang, X., Uehara, H., Muddana, S. K., et al. (2014). Vimentin knockdown decreases corneal opacity. Invest. Ophthalmol. Vis. Sci. 55, 4030–4040. doi: 10.1167/iovs.13-13494
Draminski, M., Rada-Iglesias, A., Enroth, S., Wadelius, C., Koronacki, J., and Komorowski, J. (2008). Monte Carlo feature selection for supervised classification. Bioinformatics 24, 110–117. doi: 10.1093/bioinformatics/btm486
Feng, M., Pan, Y., Kong, R., and Shu, S. (2020). Therapy of primary liver cancer. Innovation 1:100032. doi: 10.1016/j.xinn.2020.100032
Gu, C., Huang, Z., Chen, X., Liu, C., Rocco, G., Zhao, S., et al. (2020a). TEAD4 promotes tumor development in patients with lung adenocarcinoma via ERK signaling pathway. Biochim Biophys. Acta Mol. Basis Dis. 1866:165921. doi: 10.1016/j.bbadis.2020.165921
Gu, C., Shi, X., Dai, C., Shen, F., Rocco, G., Chen, J., et al. (2020b). RNA m6A modification in cancers: molecular mechanisms and potential clinical applications. Innovation. 1:100066. doi: 10.1016/j.xinn.2020.100066
Gu, C., Shi, X., Huang, Z., Chen, J., Yang, J., Shi, J., et al. (2020c). A comprehensive study of construction and analysis of competitive endogenous RNA networks in lung adenocarcinoma. Biochim Biophys. Acta Proteins Proteom. 1868:140444. doi: 10.1016/j.bbapap.2020.140444
Gu, C., Pan, X., Chen, Y., Yang, J., Zhao, H., and Shi, J. (2018). Short-term and mid-term survival in bronchial sleeve resection by robotic system versus thoracotomy for centrally located lung cancer. Eur. J. Cardiothorac. Surg. 53, 648–655. doi: 10.1093/ejcts/ezx355
Ho, J., Rush, D. N., Gibson, I. W., Karpinski, M., Storsley, L., Bestland, J., et al. (2010). Early urinary CCL2 is associated with the later development of interstitial fibrosis and tubular atrophy in renal allografts. Transplantation 90, 394–400. doi: 10.1097/TP.0b013e3181e6424d
Huang, W., Liang, J., Kazmierczak, K., Muthu, P., Duggal, D., Farman, G. P., et al. (2014). Hypertrophic cardiomyopathy associated Lys104Glu mutation in the myosin regulatory light chain causes diastolic disturbance in mice. J. Mol. Cell Cardiol. 74, 318–329. doi: 10.1016/j.yjmcc.2014.06.011
Jiao, D., and Yang, S. (2020). Overcoming resistance to drugs targeting KRASG12C mutation. Innovation 1:100035. doi: 10.1016/j.xinn.2020.100035
Juanola, O., Gomez-Hurtado, I., Zapater, P., Moratalla, A., Caparros, E., Pinero, P., et al. (2016). Selective intestinal decontamination with norfloxacin enhances a regulatory T cell-mediated inflammatory control mechanism in cirrhosis. Liver Int. 36, 1811–1820. doi: 10.1111/liv.13172
Kanno, Y., Shu, E., Kanoh, H., Matsuda, A., and Seishima, M. (2017). alpha2AP regulates vascular alteration by inhibiting VEGF signaling in systemic sclerosis: the roles of alpha2AP in vascular dysfunction in systemic sclerosis. Arthritis Res. Ther. 19:22. doi: 10.1186/s13075-017-1227-y
Kelly, D., Verkade, H. J., Rajanayagam, J., McKiernan, P., Mazariegos, G., and Hubscher, S. (2016). Late graft hepatitis and fibrosis in pediatric liver allograft recipients: current concepts and future developments. Liver Transpl. 22, 1593–1602. doi: 10.1002/lt.24616
Lambers Heerspink, H. J., de Borst, M. H., Bakker, S. J., and Navis, G. J. (2013). Improving the efficacy of RAAS blockade in patients with chronic kidney disease. Nat. Rev. Nephrol. 9, 112–121. doi: 10.1038/nrneph.2012.281
Lan, W., Lai, D., Chen, Q., Wu, X., Chen, B., Liu, J., et al. (2020). LDICDL: LncRNA-disease association identification based on collaborative deep learning. IEEE/ACM Trans. Comput. Biol. Bioinform. doi: 10.1109/TCBB.2020.3034910 Online ahead of print.
Li, J., Lu, L., Zhang, Y.-H., Xu, Y., Liu, M., Feng, K., et al. (2020a). Identification of leukemia stem cell expression signatures through monte carlo feature selection strategy and support vector machine. Cancer Gene Therapy 27, 56–69. doi: 10.1038/s41417-019-0105-y
Li, J., Xu, Q., Wu, M., Huang, T., and Wang, Y. (2020b). Pan-Cancer classification based on self-normalizing neural networks and feature selection. Front. Bioeng. Biotechnol. 8:766. doi: 10.3389/fbioe.2020.00766
Madahar, P., Duprez, D. A., Podolanczuk, A. J., Bernstein, E. J., Kawut, S. M., Raghu, G., et al. (2018). Collagen biomarkers and subclinical interstitial lung disease: the multi-ethnic study of atherosclerosis. Respir. Med. 140, 108–114. doi: 10.1016/j.rmed.2018.06.001
Mao, L., Liu, L., Zhang, T., Wu, X., Zhang, T., and Xu, Y. (2019). MKL1 mediates TGF-beta-induced CTGF transcription to promote renal fibrosis. J. Cell Physiol. 235, 4790–4803. doi: 10.1002/jcp.29356
Marino, C. R., Matovcik, L. M., Gorelick, F. S., and Cohn, J. A. (1991). Localization of the cystic fibrosis transmembrane conductance regulator in pancreas. J. Clin. Invest. 88, 712–716. doi: 10.1172/JCI115358
Pan, X., Chen, L., Feng, K. Y., Hu, X. H., Zhang, Y. H., Kong, X. Y., et al. (2019a). Analysis of expression pattern of snoRNAs in different cancer types with machine learning algorithms. Int. J. Mol. Sci. 20:2185. doi: 10.3390/ijms20092185
Pan, X., Hu, X., Zhang, Y.-H., Chen, L., Zhu, L., Wan, S., et al. (2019b). Identification of the copy number variant biomarkers for breast cancer subtypes. Mol. Genet. Genom. 294, 95–110. doi: 10.1007/s00438-018-1488-1484
Pan, X., Zeng, T., Yuan, F., Zhang, Y. H., Chen, L., Zhu, L., et al. (2019c). Screening of methylation signature and gene functions associated with the subtypes of isocitrate dehydrogenase-mutation gliomas. Front. Bioeng. Biotechnol. 7:339. doi: 10.3389/fbioe.2019.00339
Pan, X., Hu, X., Zhang, Y. H., Feng, K., Wang, S. P., Chen, L., et al. (2018). Identifying patients with atrioventricular septal defect in down syndrome populations by using self-normalizing neural networks and feature selection. Genes (Basel) 9:208. doi: 10.3390/genes9040208
Pan, X., Zeng, T., Zhang, Y. H., Chen, L., Feng, K., Huang, T., et al. (2020). Investigation and prediction of human interactome based on quantitative features. Front. Bioeng. Biotechnol. 8:730. doi: 10.3389/fbioe.2020.00730
Qi, S. S., Zheng, H. X., Jiang, H., Yuan, L. P., and Dong, L. C. (2020). Protective effects of chromium picolinate against diabetic-induced renal dysfunction and renal fibrosis in streptozotocin-induced diabetic rats. Biomolecules 10:398. doi: 10.3390/biom10030398
Ren, X., Wang, S., and Huang, T. (2020). Decipher the connections between proteins and phenotypes. Biochim Biophys. Acta Proteins Proteom 1868:140503. doi: 10.1016/j.bbapap.2020.140503
Rockey, D. C. (2013). Translating an understanding of the pathogenesis of hepatic fibrosis to novel therapies. Clin. Gastroenterol. Hepatol. 11, 224–31.e1-5. doi: 10.1016/j.cgh.2013.01.005
Rockey, D. C., Bell, P. D., and Hill, J. A. (2015). Fibrosis–A common pathway to organ injury and failure. N. Engl. J. Med. 373:96. doi: 10.1056/NEJMc1504848
Shi, X., Huang, T., Wang, J., Liang, Y., Gu, C., Xu, Y., et al. (2018). Next-generation sequencing identifies novel genes with rare variants in total anomalous pulmonary venous connection. EBioMedicine 38, 217–227. doi: 10.1016/j.ebiom.2018.11.008
Sieber, P., Schafer, A., Lieberherr, R., Le Goff, F., Stritt, M., Welford, R. W. D., et al. (2018). Novel high-throughput myofibroblast assays identify agonists with therapeutic potential in pulmonary fibrosis that act via EP2 and EP4 receptors. PLoS One 13:e0207872. doi: 10.1371/journal.pone.0207872
Szklarczyk, D., Gable, A. L., Lyon, D., Junge, A., Wyder, S., Huerta-Cepas, J., et al. (2018). STRING v11: protein-protein association networks with increased coverage, supporting functional discovery in genome-wide experimental datasets. Nucleic Acids Res. 47, D607–D613. doi: 10.1093/nar/gky1131
Tan, C. Y., Wong, J. X., Chan, P. S., Tan, H., Liao, D., Chen, W., et al. (2019). Yin Yang 1 suppresses dilated cardiomyopathy and cardiac fibrosis through regulation of Bmp7 and Ctgf. Circ. Res. 125, 834–846. doi: 10.1161/CIRCRESAHA.119.314794
Vega-Carrascal, I., Bergin, D. A., McElvaney, O. J., McCarthy, C., Banville, N., Pohl, K., et al. (2014). Galectin-9 signaling through TIM-3 is involved in neutrophil-mediated Gram-negative bacterial killing: an effect abrogated within the cystic fibrosis lung. J. Immunol. 192, 2418–2431. doi: 10.4049/jimmunol.1300711
Wang, M., Zhao, Y., and Zhang, B. (2015). Efficient test and visualization of multi-set intersections. Sci. Rep. 5:16923. doi: 10.1038/srep16923
Wynn, T. A., and Ramalingam, T. R. (2012). Mechanisms of fibrosis: therapeutic translation for fibrotic disease. Nat. Med. 18, 1028–1040. doi: 10.1038/nm.2807
Yan, L. T., Wang, L. L., Yao, J., Yang, Y. T., Mao, X. R., Yue, W., et al. (2020). Total bile acid-to-cholesterol ratio as a novel noninvasive marker for significant liver fibrosis and cirrhosis in patients with non-cholestatic chronic hepatitis B virus infection. Medicine (Baltimore) 99:e19248. doi: 10.1097/MD.0000000000019248
Zeisberg, M., and Kalluri, R. (2013). Cellular mechanisms of tissue fibrosis. 1. common and organ-specific mechanisms associated with tissue fibrosis. Am. J. Physiol. Cell Physiol. 304, C216–C225. doi: 10.1152/ajpcell.00328.2012
Keywords: fibrotic diseases, genes, pathways, Monte Carlo feature selection, CTGF
Citation: Gu C, Shi X, Dang X, Chen J, Chen C, Chen Y, Pan X and Huang T (2021) Identification of Common Genes and Pathways in Eight Fibrosis Diseases. Front. Genet. 11:627396. doi: 10.3389/fgene.2020.627396
Received: 09 November 2020; Accepted: 15 December 2020;
Published: 15 January 2021.
Edited by:
Chen Qingfeng, Guangxi University, ChinaReviewed by:
Xuechao Wan, Northwestern University, PhilippinesYungang Sun, Nanjing Chest Hospital, China
Copyright © 2021 Gu, Shi, Dang, Chen, Chen, Chen, Pan and Huang. This is an open-access article distributed under the terms of the Creative Commons Attribution License (CC BY). The use, distribution or reproduction in other forums is permitted, provided the original author(s) and the copyright owner(s) are credited and that the original publication in this journal is cited, in accordance with accepted academic practice. No use, distribution or reproduction is permitted which does not comply with these terms.
*Correspondence: Tao Huang, tohuangtao@126.com; Xufeng Pan, panxfshch@163.com; Yumei Chen, chym0215@hotmail.com
†These authors have contributed equally to this work