- 1State Key Laboratory of Animal Nutrition, Beijing Engineering Technology Research Center of Raw Milk Quality and Safety Control, College of Animal Science and Technology, China Agricultural University, Beijing, China
- 2Department of Animal and Poultry Science, University of Saskatchewan, Saskatoon, SK, Canada
Limit-feeding of a high concentrate diet has been proposed as an effective method for improving feed efficiency and reducing total manure output of dairy heifers; meanwhile the effects of this method on hindgut microbiota are still unclear. This study investigated the effects of a wide range of dietary forage:concentrate ratios (F:C) on the fecal composition of bacteria and archaea in heifers using next-generation sequencing. Four diets with different F:C (80:20, 60:40, 40:60, and 20:80) were limit-fed to 24 Holstein heifers, and the fecal fermentation parameters and bacterial and archaeal communities were investigated. With increasing dietary concentrate levels, the fecal dry matter output, neutral detergent fiber (NDF) content, and proportion of acetate decreased linearly (P < 0.01), while the fecal starch content and proportions of propionate, butyrate, and total branched-chain volatile fatty acids (TBCVFAs) were increased (P ≤ 0.05). An increased concentrate level linearly increased (P = 0.02) the relative abundance of Proteobacteria, and linearly decreased (P = 0.02) the relative abundance of Bacteroidetes in feces. At the genus level, the relative abundance of unclassified Ruminococcaceae and Paludibacter which may have the potential to degrade forage decreased linearly (q ≤ 0.02) with increasing dietary concentrate levels, while the relative abundance of Roseburia and Succinivibrio which may be non-fibrous carbohydrate degrading bacteria increased linearly (q ≤ 0.05). Some core microbiota operational taxonomic units (OTUs) also showed significant association with fecal VFAs, NDF, and/or acid detergent fiber (ADF) content. Meanwhile, the relative abundance of most detected taxa in archaea were similar across different F:C, and only Methanosphaera showed a linear decrease (P = 0.01) in high concentrate diets. Our study provides a better understanding of fecal fermentation parameters and microbiota under a wide range of dietary F:C. These findings support the potential for microbial manipulation by diet, which could enhance feed digestibility and relieve environmental problems associated with heifer rearing.
Introduction
Ruminants are uniquely able to convert large quantities of plant fiber into high quality products, such as meat and milk, for human consumption. With the current rapidly exploding human population, there is a great need to increase ruminant productivity and feed conversion efficiency (Thornton, 2010). Recently, the limit-feeding of high concentrate diets has been proposed as an effective method for improving feed efficiency in heifers, and has been explored by many researchers (Suarez-Mena et al., 2015; Lascano et al., 2016; Zanton and Heinrichs, 2016). Furthermore, when heifers are raised under a limit-fed diet of enhanced energy density, intakes of feed and forage are reduced, as is fecal or total manure output (Moody et al., 2007; Zanton and Heinrichs, 2009); this is an environmentally friendly dairy farming strategy. Previous studies report that wet fecal output for high concentrate fed heifers represents 64–66% of the amounts excreted from low concentrate fed heifers (Lascano et al., 2016; Zanton and Heinrichs, 2016). However, the effects of limit-feeding different forage to concentrate ratios (F:C) on hindgut microbiota communities are still unclear.
Microbiota of the gastrointestinal tract (GIT) have an important role in animal health, welfare, and productivity, and also in food safety, pathogen shedding, and the detection of fecal microbial contamination in water or lands by microbial source tracking (Lee et al., 2011; Shanks et al., 2011; Rice et al., 2012). Previous studies report that diets and/or feeding strategy mainly determine cattle fecal bacterial community structure (Callaway et al., 2010; Shanks et al., 2011; Kim et al., 2014). However, the GIT microbiome is quite complicated, and other microbes like archaea, which are solely responsible for methane production, also live in the ruminant gut (Kumar et al., 2015; Zhang et al., 2017). Methane (CH4) emitted from ruminants accounts for 4–12% of gross energy loss, and also causes environmental problems due to its remarkable global warming potential (Johnson and Johnson, 1995; Kumar et al., 2015). Manure CH4 from ruminant livestock may contribute up to 2% of global CH4 emissions; moreover, manure CH4 emissions are a larger proportion of total farm CH4 emissions in intensively managed dairy operations with manure storage systems (Knapp et al., 2014). Therefore, a better understanding of fecal bacterial and archaeal communities under a wide range of F:C diets could be important in improving feed utilization, manipulating digestion, further increasing livestock growth performance, and even reducing related environmental problems.
Nowadays, high-through sequencing techniques have been successfully used to reveal changes and function of the microbial community of the GIT (Mao et al., 2015; Ye et al., 2016; Zhang et al., 2017). Therefore, the primary objective of this study was to evaluate the effects of a wide range of dietary F:C (four levels) on the fecal composition of bacteria and archaea in heifers using next-generation sequencing.
Materials and Methods
Animals and Diets
Twenty-four half-sib Holstein heifers (8–10 months old and 263 ± 30 kg body weight) with similar initial body condition (2.73 ± 0.03 using a five-point system body condition score) were assigned into one of four F:C treatments: 80:20, 60:40, 40:60, and 20:80, on a dry matter (DM) basis; the groups were named C20, C40, C60, and C80, respectively (Table S1). Corn silage was used as the sole forage resource, as reported in our previous study (Zhang et al., 2018). Before transfer to treatment diets, all heifers were fed an adaptation diet containing 50% forage and 50% concentrate (DM basis; Table S1) for 4 weeks. Diets were designed according to the nutrient requirements for dairy heifers (NRC, 2001). Diet quantity provided to the high concentrate groups was restricted to give a similar intake of metabolizable energy (ME) to the low concentrate groups. Additionally, diets were formulated to maintain the same crude protein (CP):ME ratios to minimize potential effects due to differences in CP intake across diets. Heifers were individually fed a total mixed ration (TMR) twice daily at 12 h intervals (07:00 and 19:00 h) and weighed weekly 2 h prior to the morning feeding (0500 h) for the adjustment of feed offered. Water was available ad libitum.
This study was carried out in Sanyuan Dairy Group, Beijing, China, and in accordance with the recommendations of Instructive Notions with Respect to Caring for Experimental Animals, Ministry of Science and Technology of China. The protocol was approved by the Ethical Committee of the College of Animal Science and Technology (Project number 31402099) of China Agricultural University.
Sampling and Measurements
To obtain representative samples, fecal samples (about 200 g/sampling) were collected from the rectum of heifers during days 20–23 of the experimental period (except for one sick heifer from the C60 group) using sterile gloves. On days 20 and 22, collection was at 08:00, 14:00, and 20:00 h, while on days 21 and 23, collection was at 02:00, 05:00 h, 11:00, 17:00, and 23:00 h to achieve a combined 2-day sample taken at 3 h intervals. After each sampling time point, half the sample was immediately stored in liquid nitrogen and the remainder was stored at −20°C. At the end of sampling, equal amounts of the liquid nitrogen samples from each time point and from the same heifer were mixed to homogeneity using a Slapping Sterile Homogenizer (Shanghai Hannuo Ltd., Shanghai China), and subsamples of ~1 g were frozen at −80°C in the laboratory for further genomic DNA extraction. In addition, equal amounts of the −20°C samples from each time point and from the same heifer were mixed to homogeneity, and subsamples of ~2 g were dissolved with 2 mL of water for the detection of volatile fatty acids (VFAs) (Mao et al., 2012). The remaining fecal samples were analyzed for DM (AOAC, 2000; method 930.15), organic matter (OM) (Van Soest et al., 1991), CP (AOAC, 2000; method 976.05), neutral detergent fiber (NDF; with a heat stable amylase and expressed inclusive of residual ash), and acid detergent fiber (ADF) (Van Soest et al., 1991). Fecal starch content was analyzed using a total starch assay kit (Megazyme, Bray, Ireland) based on AOAC method 996.11 (AOAC, 2000).
Microbial DNA Extraction, PCR Amplification, and Sequencing
Genomic DNA was extracted from the fecal samples using a FastDNA SPIN Kit (MP Biomedicals, Solon, USA) following the manufacturer's protocol. The extracted DNA was subjected to PCR amplification in triplicate using a KAPA HiFi Hotstart ReadyMix PCR kit (Life Technology, Carlsbad, USA). For bacteria, the V3–V4 region of the bacteria 16S rRNA gene was amplified using primers F341 (5′- ACTCCTACGGGRSGCAGCAG−3′) and R806 (5′- GGACTACVVGGGTATCTAATC−3′) (Sun et al., 2017). For archaea, the V3–V4 region of the archaea 16S rRNA gene was amplified using primers F341 (5′- CTACGGGGYGCASCAG′) (Wei et al., 2013) and R806 (5′- GGACTACVVGGGTATCTAATC−3′). Barcodes, an eight-base sequence unique to each sample, were added to each primer for sample identification. Amplification was performed as follows: initial denaturing step of 95°C for 3 min, followed by 25 cycles of 95°C for 30 s, annealing (60°C for bacteria/55°C for archaea, 20 s), elongation (72°C, 40 s), and a final extension step of 72°C for 10 min. Amplicons were extracted from 2% agarose gels and purified using an AxyPrep DNA Gel Extraction Kit (Axygen Bioscience, Union City, CA, USA) following the manufacturer's instructions and quantified using a NanoDrop 2000 (Thermo, Mass, USA). Illumina TruSeq PE Cluster and SBS kits (Illumina, San Diego, USA) were used to perform cluster generation, template hybridization, isothermal amplification, linearization, blocking, denaturization, and hybridization of the sequencing primers according to the manufacturer's instruction. Paired-end sequencing (2 × 250 bp) was performed to sequence all libraries on an Illumina HiSeq2500 PE250 platform (Illumina, USA) according to standard protocols (Caporaso et al., 2012).
Sequence Data Processing
The sequences obtained from the HiSeq platform were processed through an open-source software pipeline, Quantitative Insights into Microbial Ecology (QIIME) version 1.8.0-dev (Caporaso et al., 2010b), with criteria similar to a previous report (Zhang et al., 2017). Briefly, (1) the reads with an average quality score of no less than 25 and with a length of 220–250 nt were retained; (2) reads that had more than three N bases, two nucleotide mismatches in primer matching, and ambiguous characters were discarded; (3) reads that could not be assembled were discarded. Sequences were binned into operational taxonomic units (OTUs) based on 97% identity using USEARCH after removing the singletons, and chimeric sequences were identified and removed by UCHIM (Edgar, 2013). For bacterial sequencing analysis, three samples with quite low numbers of OTUs were excluded. Thus, 20 fecal samples were finally used to assess the bacterial community, with five samples from each group. The most abundant sequence within each OTU from specific libraries (libraries constructed for bacteria and archaea) was designated as the “representative sequence” and then aligned against the SILVA bacterial database (SILVA version 123) (Pruesse et al., 2007) and SILVA archaea database (SILVA version 123) using PYNAST (Caporaso et al., 2010a), respectively, with default parameters set by QIIME. Community richness and diversity, such as Good's coverages, Observed species, PD whole tree, Chao1, Shannon, and Simpson indices, which are used to illustrate significant differences among samples, were assessed by the program MOTHUR v.1.35.0 (Schloss et al., 2009). Beta diversity was measured according to unweighted UniFrac distances which were calculated by QIIME, and displayed using Principal Coordinate Analysis (PCoA). The significance of grouping in the PCoA plot was tested by analysis of similarity (ANOSIM) in QIIME with 999 permutations (R Core Team, 2014).
After assembling and filtering, all the raw sequences (bacteria and archaea) were submitted to the NCBI Sequence Read Archive (SRA; http://www.ncbi.nlm.nih.gov/Traces/sra/), under accession number SRP117671.
Quantitative Real-Time PCR Analysis
Marker loci gene copies for total bacteria, total Methanogens, the genus Prevotella, Fibrobacter succinogenes, Ruminococcus albus, and Ruminococcus flavefaciens were enumerated by quantitative PCR on a QuantStudio 6 Flex Real-Time PCR System (Thermo Scientific, Rockford, IL, USA) using SYBR Green as the fluorescent dye. The primers and reaction conditions were selected on the basis of a careful review of published literature (Table S2). The standard curves were generated from diluting plasmid DNA (10-fold serial dilution) that contained the cloned marker loci. Each reaction contained 5 μL of 2 × SYBR Premix Ex Taq II (Tli RNaseH Plus) (TaKaRa Bio Inc., Shiga, Japan), 1 μL of each primer (10 μM), 0.2 μL of Rox (Takara Bio), 80 ng of microbial DNA, and 2.3 μL of deionized water. Thermal cycling was performed at 95°C for 30 s, followed by 40 cycles of 95°C for 15 s, annealing for 30–60 s (annealing temperatures and times are shown in Table S2), and collection of fluorescent signals. All samples were prepared from the heifers and each sample was assayed in triplicate. All obtained standard curves met the required standards of efficiency (R2 > 0.99, 110% > E > 90%). Results were expressed as the log10 transfer of numbers of marker loci gene copies per gram of feces (wet weight).
Correlations Between the Fecal Microbiota OTUs and Nutrient Content and VFAs Concentrations
OTUs that appeared in all the samples with an abundance > 0.001 were considered as core OTUs (Table S3). Fecal fermentation parameters, nutrient contents, and core microbiota OTUs which can be identified at family and/or genus levels were used for interactive analysis in R (R Core Team, 2014). Spearman's rank correlations and q-values were calculated using the Psych packages [http://cran.r-project.org/web/packages/psych; author, W. Revelle; published date, 2016; version, 1.6.9; FDR correct (q-value) was embedded in the package] and visualized using the corrplot package (https://cran.r-project.org/web/packages/corrplot/; author, Taiyun Wei; published date, 2017; version, 0.84) in R. Correlations had an absolute Spearman's correlation > 0.50 with a q-value under 0.05.
Statistical Analysis
Statistical analyses were performed using R software packages. The dietary effects were tested using an estimability package (https://cran.r-project.org/package=estimability; author: R. V. Lenth; published date: 2015; version: 1.1-1) in R. The lm function of estimability package in R was used to evaluate the linear, quadratic, and cubic effects of the dietary concentrate levels on the variables. Standard errors of the mean were reported. Significance was declared at P ≤ 0.05, and trend was reported at 0.05 < P < 0.10. Furthermore, all P-values from the multiple comparison analyses of the bacterial community (genus level) were adjusted by the FDR using the BonEV package (https://cran.r-project.org/package=BonEV; author, D. Li; published date, 2016; version, 1.0) with p.adjust in R. FDR-corrected P-values ≤ 0.05 (q ≤ 0.05) were considered a significant difference.
Results
Fecal Nutrient and VFAs Content
Fecal DM output and OM, NDF, and ADF content decreased linearly (P < 0.01) with increasing amounts of concentrate in the diets, while CP and starch content increased linearly (P ≤ 0.05; Table 1). The proportion of acetate and acetate:propionate ratios in feces decreased linearly (P ≤ 0.01) with increasing dietary concentrate levels, while the proportion of butyrate was increased linearly (P < 0.01). The proportion of propionate increased quadratically (P = 0.03) with increasing dietary concentrate levels with the C60 group having the highest value. The proportions of isobutyrate, isovalerate, and total branched-chain volatile fatty acids (TBCVFA) increased quadratically (P = 0.01) with the C40 group having the lowest values.
Sequence and General Bacterial Community Composition
A total of 687,332 quality-checked sequences were obtained from all the 20 samples, and 30,518–38,910 sequences were returned for each sample. After OTU picking and chimera checking, a total of 1,091 OTUs were calculated for all the samples at 3% dissimilarity. The number of OTUs for the C20, C40, C60, and C80 groups were 752, 720, 710, and 715, respectively; furthermore, 801 OTUs were found in all four treatments (Figure S1A). Good's coverage for each sample was more than 0.99.
A total of 14 phyla were detected via taxonomic analyses. The five most abundant phyla were Firmicutes, Bacteroidetes, Verrucomicrobia, Spirochaetes, and Proteobacteria, representing 45.34, 41.67, 4.02, 3.22, and 1.66% of total sequences, respectively (Figure 1A). At the genus level, a total of 92 bacterial genera were detected, and 33 genera were common in all samples; these were identified as the core bacterial microbiome (Figure S2A). The seven most abundant genera were unclassified Ruminococcaceae, Alistipes, Bacteroides, Paraprevotella, unclassified Porphyromonadaceae, unclassified Lachnospiraceae, and Akkermansia, representing 22.53, 6.54, 6.00, 4.63, 4.55, 3.74, and 3.61% of total sequences, respectively (Figure 1B).
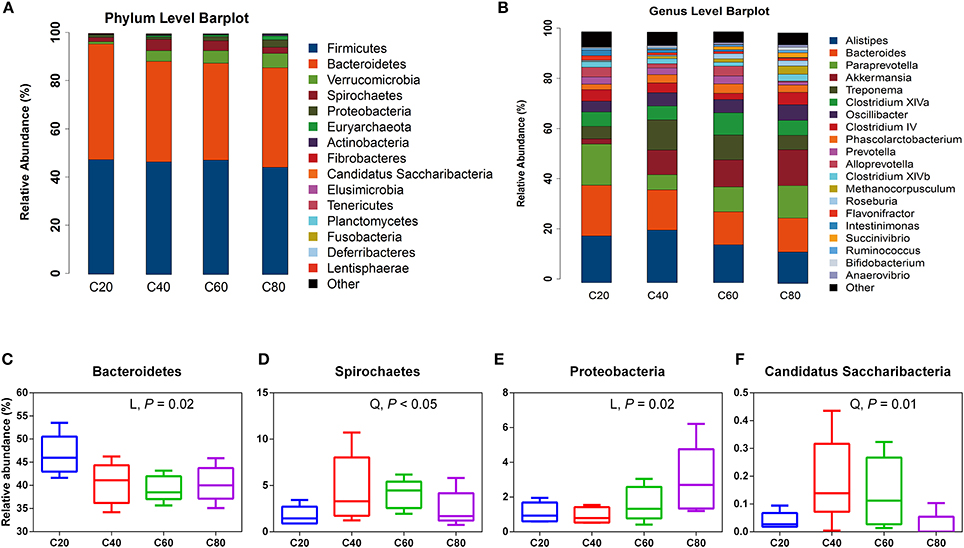
Figure 1. Fecal bacterial phyla and genera in four treatments. (A) The bacterial taxonomic composition of fecal samples from the four treatments at the phylum level. (B) The bacterial taxonomic composition of fecal samples from the four treatments at the genus level (top 20, according to relative abundance). The significantly changed bacterial phyla Bacteroidetes (C), Spirochaetes (D), Proteobacteria (E), and Candidatus Saccharibacteria (F) among treatments. The small box plots show the 25, 50, and 75th percentiles, with whiskers showing the extremes of the data. L, changed linearly; Q, changed quadratically. C20, diet containing 20% of concentrate; C40, diet containing 40% of concentrate; C60, diet containing 60% of concentrate; C80, diet containing 80% of concentrate.
Effects of Increasing Dietary Concentrate Levels on Fecal Bacterial Communities
After rarefaction, 22,000 sequences per sample were used for diversity analysis. Alpha bacterial diversity is presented in Table 2. Similar values for Chao1, Observed species, and PD whole tree indices were observed among the four groups (P > 0.05). The diversity indices (Shannon and Simpson indices) linearly decreased (P < 0.01) with increasing concentrate levels in diets.
PCoA analysis of overall diversity based on unweighted UniFrac metrics was performed to compare the four treatments (Figure 2A). ANOSIM showed significant differences in bacterial community composition between treatments C20 and C60 (R = 0.476, P < 0.01), between treatments C20 and C80 (R = 0.836, P = 0.01), and between treatments C40 and C80 (R = 0.572, P = 0.02). A tendency of difference was found between treatments C20 and C40 (R = 0.172, P = 0.08) and between treatments C60 and C80 (R = 0.268, P = 0.08). No significant difference was found between treatments C40 and C60 (R = 0.140, P = 0.16).
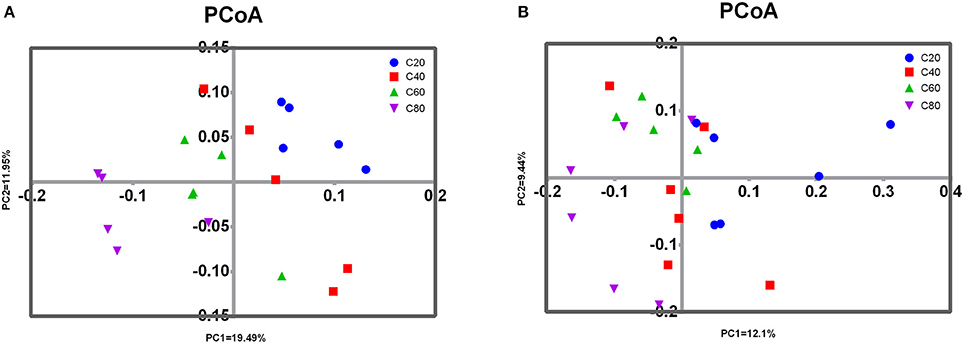
Figure 2. Principal coordinated analysis (PCoA) of fecal microbial communities. Unweighted PCoA by fecal bacteria (A) and archaea (B) microbiota. C20, diet containing 20% of concentrate; C40, diet containing 40% of concentrate; C60, diet containing 60% of concentrate; C80, diet containing 80% of concentrate.
At the phylum level, the relative abundance of Proteobacteria increased linearly (P = 0.02) with increasing dietary concentrate levels, while the relative abundance of Bacteroidetes decreased linearly (P = 0.02; Table 3, Figures 1C,E). The relative abundances of Candidatus Saccharibacteria and Spirochaetes quadratically decreased (P < 0.05; Figures 1D,F) with increasing dietary concentrate levels, with the C40 group having the highest values. At the genus level, the relative abundances of Roseburia, Succinivibrio, and Clostridium sensu strict increased linearly (q ≤ 0.05) with increasing dietary concentrate levels, while the relative abundances of unclassified Ruminococcaceae, Intestinimonas, and Paludibacter decreased linearly (q ≤ 0.02; Table 4).
Sequence and General Archaeal Community Compositions
Following denoising and chimera checking, a total of 829,456 clean sequence reads were generated from 23 fecal samples. The sequence reads, which had an average length of 388 bp among the samples, were assigned to 1,178 OTUs. We obtained an average of 36,063 sequences per animal across the fecal samples, and each sample had an average of 399 OTUs. After normalization to 30,000 sequences, individual sample OTUs ranged from 286 to 523, and 521 OTUs were presented in all the samples (Figure S1B).
Two distinct phyla were were identified in all the fecal samples. The most abundant phylum was Euryarchaeota, which comprised 87.63% of the sequence reads, with only several sequences belonging to Thaumarchaeota as the remaining phylum. Unclassified sequences comprised 12.37% of the total reads. At the genus level, we detected six distinct genera among the treatments, and three genera were common in all samples; these were identified as the core microbiome (Figure S2B). The most abundant known genera were Methanocorpusculum (48.17%) and Methanobrevibacter (37.67%; Table 5). Other minor genera such as Methanosphaera, Methanimicrococcus, Methanosarcina, and Nitrososphaera accounted for 0.20, 0.01, < 0.01, and < 0.01%, respectively. The remainder of the sequences (12.37%) were unclassified at the genus level.
Effects of Increasing Dietary Concentrate Levels on Fecal Archaeal Communities
Analyses of archaeal 16S rRNA gene fragments revealed no significant differences in richness (Chao1 and PD whole tree) and diversity (Shannon and Simpson indices) with increasing dietary concentrate levels (Table 2). PCoA analysis of overall diversity based on unweighted UniFrac metrics was performed to compare the four treatments (Figure 2B). ANOSIM showed significant difference in archaeal community composition between treatments C80 and C20 (R = 0.572, P = 0.02). However, there were no significant differences between other treatment comparisons (P > 0.10). At the genus level, only the relative abundance of Methanosphaera decreased linearly (P = 0.01) with increasing dietary concentrate levels (Table 5).
Changes in the Density of Fecal Microbiota
Quantitative PCR data revealed the relationships between the different levels of dietary concentrate and the populations of microbial taxa (Table 6). However, no significant differences were found in the density of detected fecal microbiome taxa.
Correlations Between Fecal Core OTUs and Main Nutrient and VFAs Content
Only one core OTU derived from Oscillibacter was positively associated (r = 0.76, q = 0.01) with total VFA (TVFA) content (Figure 3). Seven core OTUs derived from Alistipes, Bacteroides, Intestinimonas, and unclassified Ruminococcaceae were positively (r > 0.67, q < 0.05) and three core OTUs derived from Clostridium XlVa, Succinivibrio, and unclassified Ruminococcaceae were negatively (r < −0.68, q < 0.04) associated with acetate content. Four core OTUs derived from Clostridium XlVa, unclassified Lachnospiraceae, Roseburia, and unclassified Ruminococcaceae were positively (r > 0.69, q < 0.04) and two core OTUs derived from Bacteroides and unclassified Ruminococcaceae were negatively (r < −0.74, q < 0.02) associated with butyrate content. Two core OTUs derived from Clostridium XlVb and unclassified Ruminococcaceae were positively (r > 0.66, q < 0.04) and two core OTUs derived from unclassified Ruminococcaceae were negatively (r < −0.70, q < 0.03) associated with TBCVFA content. Two core OTUs derived from unclassified Lachnospiraceae and Oscillibacter were positively (r > 0.66, q < 0.05) and two core OTUs derived from Bacteroides and unclassified Ruminococcaceae were negatively (r < −0.67, q < 0.04) associated with CP content. One core OTU derived from unclassified Ruminococcaceae was positively (r = 0.70, q = 0.03) and one core OTU derived from Succinivibrio was negatively (r = −0.71, q = 0.02) associated with NDF content. Nine core OTUs derived from Bacteroides, Intestinimonas, Paludibacter, and unclassified Ruminococcaceae were positively (r > 0.67, q < 0.05) and three core OTUs derived from Oscillibacter, unclassified Ruminococcaceae, and Succinivibrio were negatively associated (r < −0.74, q < 0.02) with ADF content. One core OTU derived from Alistipes was positively (r = 0.68, q = 0.01) and one core OTU derived from unclassified Lachnospiraceae was negatively (r = −0.71, q = 0.02) associated with starch content.
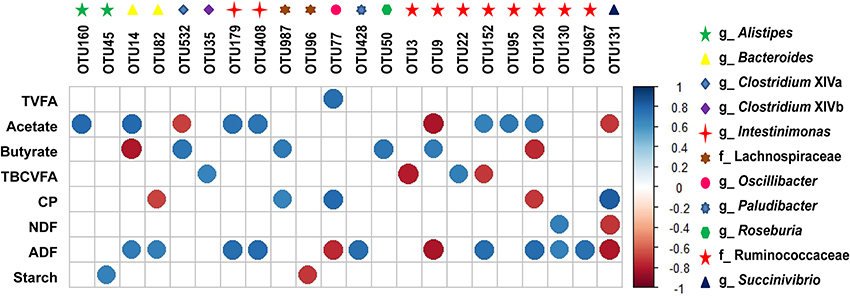
Figure 3. Correlations between fecal core microbiota OTUs and nutrient and VFA content. Spearman non-parametric rank correlation matrix of the core microbiota OTUs and nutrient contents and VFA proportions (molar%). Only significant correlations were shown (r > 0.50 or r < −0.50 and q-value < 0.05). The scale colors denote whether the correlation is positive (closer to 1, dark blue) or negative (closer to −1, dark red) between the OTUs and nutrient and VFA contents. CP, crude protein; NDF, neutral detergent fiber; ADF, acid detergent fiber; TVFA, total volatile fatty acid; TBCVFA, total branch-chain volatile fatty acids; OTUs, operational taxonomic units.
Discussion
Fecal Nutrient and VFAs Content
As described in our previous study, the intakes of DM, OM, NDF, and ADF decreased linearly with increasing diet concentrate levels, while intakes of starch increased linearly (Zhang et al., 2018). In addition, feed efficiency (average daily gain/dry matter intake) was linearly increased (P < 0.01) with increasing dietary concentrate levels (Zhang et al., 2018). These findings might partly explain the changed nutrient content in the feces of the heifers in this study. Because a limit-feeding strategy was used in our study, the TVFA concentrations were a little lower than that in previous reports (Li et al., 2012; Mao et al., 2012, 2015). Similar to the ruminal TVFA concentrations (Zhang et al., 2017), the proportion of fecal acetate decreased in high concentrate diets, while the proportions of fecal propionate and butyrate increased. The butyrate concentration in our study was lower than 8 mM, which is considered as a threshold for inducing severe intestinal epithelial cell apoptosis and disruption of the intestinal barrier (Peng et al., 2007). Moreover, the multiple sampling time points used in our study might have reduced sampling bias as the total feces output (5.33–11.01 kg/d, wet weight) was much greater than the amount of each sample (Mao et al., 2012; Suarez-Mena et al., 2015; Lascano et al., 2016).
Fecal Bacterial Community Composition
With increasing dietary concentrate levels, fecal bacterial diversity linearly decreased, which agreed with other reports where high proportions of cereals were fed to cattle (Plaizier et al., 2016, 2017). This finding might be due to the lower amounts of available nutrients in the hindgut as concentrates are preferentially fermented in the foregut (Shanks et al., 2011; Li et al., 2012). Our findings showed that Firmicutes and Bacteroidetes were the most predominant bacteria in feces, which agreed with previous studies (Rice et al., 2012; Li et al., 2017; Plaizier et al., 2017). In addition, the relative abundance of Firmicutes and Bacteroidetes was comparable to that of the rumen, cecum, and feces in similar studies, where members of the families Clostridiaceae, Lachnospiraceae, Ruminococcaceae, and Prevotellaceae dominated (de Oliveira et al., 2013; Hua et al., 2017; Plaizier et al., 2017). Previous studies report that the ratios of Firmicutes to Bacteroidetes are related to energy harvesting and body fat in humans and mice (Ley et al., 2006; Turnbaugh et al., 2006). Meanwhile, in ruminants, fecal Firmicutes to Bacteroidetes ratios are hardly affected by diets as most of the energy is produced in the foregut rather than the hindgut (Plaizier et al., 2016; Li et al., 2017). Compared with Firmicutes, Bacteroidetes has more glycoside hydrolases (GHs) and polysaccharide lyases (PLs) genes per genome and acts as one of the main degraders of many complex polysaccharides in plant cell walls (Meale et al., 2016). In heifers fed high concentrate diets, the competitiveness of Bacteroidetes declines allowing other phyla such as Candidatus Saccharibacteria and Proteobacteria to proliferate faster, resulting in an increase of these two phyla, which may be a reflection of the lower amounts of available fibrous material in the hindgut.
Similarly, unclassified Ruminococcaceae and Lachnospiraceae, Bacteroides, Alistipes, Akkermansia, Clostridium, Ruminococcus, and Prevotella have been found to predominate in the feces (Callaway et al., 2010; Deng et al., 2017; Li et al., 2017). Unclassified Ruminococcaceae and Lachnospiraceae, Prevotella, and Ruminococcus have also been detected in 742 rumen samples of 32 types of ruminants from all over the world and might be considered as the “core bacterial microbiome” at the genus level (Henderson et al., 2015). These results indicate that the rumen and feces share a “core bacterial microbiome” at family and/or genus levels and that these bacteria play an important role in the GIT ecosystem (Callaway et al., 2010). However, because the type and amounts of nutrients available to the microbes in the lower tract differ substantially from those in the rumen, it is likely that there are substantial differences between the rumen and the lower GIT with respect to the identity and abundance of individual species within each of these core families and genera (Mao et al., 2015; Popova et al., 2017; Ji et al., 2018). Unclassified Ruminococcaceae and Ruminococcus, in the same bacterial family of Ruminococcaceae, both might have important roles in fiber digestion (Russell and Rychlik, 2001; Liu et al., 2016; Shen et al., 2017). Considering the roles of unclassified Ruminococcaceae on fiber digestion (Dowd et al., 2008; Shen et al., 2017), their reduced population in the feces in our study might be due to the reduced fiber content in the hindgut of heifers fed increasing amounts of concentrates. Previous studies also show that the abundance of Paludibacter is positively related with dietary forage levels, indicating that they might have the ability to degrade fiber (Nathani et al., 2015; Plaizier et al., 2016). Similarly, our study found a decreased abundance of Paludibacter in heifers fed high concentrate diets. These results can be further confirmed by the correlations between most core OTUs derived from unclassified Ruminococcaceae and Paludibacter and the fecal NDF and/or ADF contents (Figure 3).
Bacteroides are well-known intestinal bacteria with the ability to utilize polysaccharides; they occupy about 5.07–13.7% of cattle feces (Dowd et al., 2008; Callaway et al., 2010; Rice et al., 2012). Previous reports state that Bacteroides populations decrease in the feces of cattle fed forage enriched diets (Kim et al., 2014; Nathani et al., 2015; Meale et al., 2016). However, no significant differences were found in the abundance of Bacteroides and some other fiber utilizers among the treatments in our study; this might be due to insufficient fiber intake in the high dietary forage groups due to the use of the limit-feeding strategy. Alistipes is another common GIT bacteria that was previously classified as a member of the genus Bacteroides (Dowd et al., 2008; Kim et al., 2014; Klein-Jöbstl et al., 2014). Therefore, it was reasonable to infer that Alistipes might have a similar function to Bacteroides in GIT.
Akkermansia is reported as one of the important bacteria in the lower intestinal tract with a variety of functions related to glucose homeostasis, mucosa health, lipid and energy metabolism, and inflammation markers; it can also use intestinal mucins to produce acetate and propionate for host intestine epithelial cells (Shin et al., 2014; Schneeberger et al., 2015; Guo et al., 2017). In accordance with other studies, Akkermansia predominated in the heifers' feces (Dowd et al., 2008; Li et al., 2017), which might be beneficial to their GIT health, although further investigation is needed.
As a ubiquitous genus in the gastrointestinal tract, Clostridium spp. can have both positive and negative health effects on host animals, with the specific effects being related to the individual Clostridium species involved (Kopecný et al., 1996; Ozutsumi et al., 2005; Dowd et al., 2008). Even though some members of Clostridium sensu stricto are generally perceived as pathogenic, the density of some clostridia can be found up to 107-1011 cells/g of intestinal content of healthy individuals (Rajilić-Stojanović and de Vos, 2014). The reasons for the increased abundance of Clostridium sensu stricto in the high concentrate diet heifers in our study needs further investigation, and the specific species also requires identification. Prevotella produces a variety of extracellular degradative enzymes and is believed to be a starch degrader (Danielsson et al., 2017). In previous studies, cattle fecal Prevotella is commonly found associated with dietary, age, and/or seasonal differences (Durso et al., 2012; Kim et al., 2014; Meale et al., 2016). While in this study, the relative abundance of Prevotella was only slightly decreased in the feces of the C80 group compared with other groups, which might be due to the higher levels of starch degradation in the foregut.
Intestinimonas is a newly described bacterial genus with representative strains present in the GIT of humans, mice, and other animals; they have the ability to convert lysine and sugar into butyrate and acetate (Bui et al., 2016; Durand et al., 2017). In vitro tests note that some strains of Intestinimonas, when isolated from murine and human intestines, grow poorly in hexose sugars; however, growth is much improved by the addition of acetate (Bui et al., 2016). These findings were confirmed by our study which showed that Intestinimonas was significantly positively associated with fecal acetate content (Figure 3). Nevertheless, the relative abundance of Intestinimonas decreased linearly with increasing amounts of dietary concentrates, even though high concentrate fed heifers had higher fecal protein and starch contents (Table 1). There might be two reasons for these findings. Firstly, the CP and starch reaching the hindgut might be less available as most of it is insoluble or indigestible (Lukas et al., 2005; Wang et al., 2009). Secondly, the species of Intestinimonas in the GIT of ruminants might be different from that in the GIT of humans and mice. Hence, further studies are needed to examine the species level of gut microbiota. To our knowledge, we suggest that this is one of the first studies to report Intestinimonas in ruminant GIT.
Succinivibrio and Roseburia seem to be increased by diets rich in grain, and might contribute to the fermentation of a variety of nonstructural carbohydrates (Kim et al., 2014; Henderson et al., 2015; Plaizier et al., 2017). In accordance, our study found that the abundance of Succinivibrio and Roseburia increased linearly in the feces of heifers fed increasing levels of dietary concentrates. Roseburia was also considered as a butyrate producer (Pryde et al., 2002). These results might partly explain the positive association between Roseburia and fecal butyrate content, and the negative association between Succinivibrio and fecal NDF and ADF contents (Figure 3). Meanwhile, caution is suggested, because the correlation analyses in this study were based on combined calculations; some non-significant correlations do not indicate there is no real interaction between microbial taxa and nutrient and VFA content, and significant correlations should be confirmed by further experimentation (Berry and Widder, 2014; Patra and Yu, 2015; Shen et al., 2017).
Fecal Archaeal Community Composition
Euryarchaeota is recognized as the most abundant archaeal phylum in ruminants (Kumar et al., 2015; Danielsson et al., 2017; Zhou et al., 2017). Methanobrevibacter is reported to be the most abundant archaeal genus in the rumen as well as in most samples from dairy feces (Kumar et al., 2015; Holman et al., 2016). However, our study found that both Methanobrevibacter and Methanocorpusculum were predominant in the feces of Holstein dairy heifers. Our results agree with those of Daquiado et al. (2014), who report that Methanocorpusculum populations can be found at up to 54.6% in the rectal dung of Hanwoo (Bos taurus coreanae). In addition, Jin et al. (2017) found fecal Methanocorpusculum could be increased up to 39.4% when active dried yeast is added into diets. These inconsistencies might be due to different animal species, sampling methods, and/or primers used by different authors (Jin et al., 2017). Methanosphaera is reported to uniquely generate CH4 by reducing methanol with H2, and is present at lower levels in the rumen of efficient cattle (Zhou et al., 2009; Jin et al., 2017). Similarly, in our study, Methanosphaera abundance decreased linearly in the feces of heifers fed a high concentrate ration and which had been identified as efficient heifers in our previous study (Zhang et al., 2018). Furthermore, the decreased abundance of Methanosphaera in feces might be helpful in reducing CH4 emission in ruminants (Knapp et al., 2014). Even though the total level of methanogens was similar among treatments, total CH4 emission could decrease with the linear decrease in fecal output found with increasing levels of dietary concentrates (Table 1). In a sense, limit-feeding of high concentrate diets could be an efficient and environmentally friendly strategy for heifer rearing (Zanton and Heinrichs, 2009; Zhang et al., 2018).
In summary, this study determined the fecal fermentation parameters, bacterial, and archaeal compositions of heifers limit-fed a wide range of F:C diets based on 16S rRNA gene sequencing. This study demonstrated that different F:C diets had significant effects on the fecal bacterial communities, while they had only small effects on the fecal archaeal communities. The most affected bacterial phyla were Bacteroidetes and Proteobacteria. The change in core fecal microbiota taxa, such as unclassified Ruminococcaceae, Intestinimonas, Succinivibrio, and Roseburia, had a close relation with fecal VFAs, NDF, and/or ADF content. These conclusions provide a framework for understanding the microbiota present in the GIT of dairy heifers fed a wide range of dietary F:C ratio, which could be helpful in providing integrative information on their potential for manipulation and relieve some environment problems. Meanwhile, the most beneficial way to implement a limit-feeding strategy with the aim of changing GIT microbiota and subsequently improving feed digestion and/or conversion efficiency still needs further investigation.
Author Contributions
SL, YW, ZC, HY, HS, and JZ conceived and designed the experiments. JZ and HS conducted the experiments and performed all statistical analyses. Finally, the paper was written by JZ, and was revised by SL, YW, ZC, and HY. All authors read and approved the final manuscript.
Funding
This study was funded by grants from the National Natural Science Foundation of China (grants no. 31772628 and 31402099), National Dairy Industry and Technology System (CARS-37), and National Key R&D Program of China (S2016G4513).
Conflict of Interest Statement
The authors declare that the research was conducted in the absence of any commercial or financial relationships that could be construed as a potential conflict of interest.
Acknowledgments
We are thankful for the help of workers from the Sanyuan Dairy Group as well as members in SL lab for their assistance in sample collections.
Supplementary Material
The Supplementary Material for this article can be found online at: https://www.frontiersin.org/articles/10.3389/fmicb.2018.00976/full#supplementary-material
Table S1. Ingredient and chemical composition of experimental diets. 1OM, organic matter; CP, crude protein; NDF, neutral detergent fiber; NFC, non-fibrous carbohydrates; ME, metabolizable energy. 2Pre-experimental diet. 3Contained 18.50% Ca, 6.00% P, 4.2% Mg, 1.4% K, 2.6% S, 7.5% Na, 12.0% Cl, 30 mg/kg of Se, 0.25% Zn, 0.25% Fe, 0.25% Mn, 1,100 mg/kg of Cu, 15 mg/kg of I, 265,000 IU/kg of vitamin A, 110,200 IU/kg of vitamin D, and 2,300 IU/kg of vitamin E. 4NFC = 100 – (NDF + CP + ether extract + ash). 5Estimated as ME = total digestible nutrients × 0.04409 × 0.82.
Table S2. Real-time PCR primers used in this study. 1W = A or T; B = T or G or C, R = A or G.
Table S3. The identified core OTUs in this study. The numbers of fecal bacterial and archaeal OTUs that appeared in all the samples with an abundance > 0.001 were displayed. OTUs, operational taxonomic units. C20, diet containing 20% of concentrate; C40, diet containing 40% of concentrate; C60, diet containing 60% of concentrate; C80, diet containing 80% of concentrate.
Figure S1. Venn diagram illustrating the overlap of microbial OTUs at 3% dissimilarity level among treatments. Venn diagram of bacteria (A) and archaea (B) operational taxonomic units (OTUs). C20, diet containing 20% of concentrate; C40, diet containing 40% of concentrate; C60, diet containing 60% of concentrate; C80, diet containing 80% of concentrate.
Figure S2. The shared bacterial and archaeal genera across fecal samples of heifers. (A) Relative abundance of shared bacterial genera across all fecal samples. (B) Relative abundance of shared archaeal genera across all fecal samples. Percentage is shown on the X-axis. Boxes represent the interquartile range (IQR) between the first and third quartiles (25th and 75th percentiles, respectively); the line inside the box defines the median. Whiskers represent the lowest and highest values within 1.5 times the IQR from the 1st and 3rd quartiles, respectively. Samples with a relative abundance for a given genus exceeding those values are represented as points beside the boxes.
References
Aoac, I. (2000). Association of Official Analytical Chemists, 17th Edn. Arlington, VA: AOAC International.
Berry, D., and Widder, S. (2014). Deciphering microbial interactions and detecting keystone species with co-occurrence networks. Front. Microbiol. 5:219. doi: 10.3389/fmicb.2014.00219
Bui, T. P., Shetty, S. A., Lagkouvardos, I., Ritari, J., Chamlagain, B., Douillard, F. P., et al. (2016). Comparative genomics and physiology of the butyrate-producing bacterium Intestinimonas butyriciproducens. Environ. Microbiol. Rep. 8, 1024–1037. doi: 10.1111/1758-2229.12483
Callaway, T. R., Dowd, S. E., Edrington, T. S., Anderson, R. C., Krueger, N., Bauer, N., et al. (2010). Evaluation of bacterial diversity in the rumen and feces of cattle fed different levels of dried distillers grains plus solubles using bacterial tag-encoded FLX amplicon pyrosequencing. J. Anim. Sci. 88, 3977–3983. doi: 10.2527/jas.2010-2900
Caporaso, J. G., Bittinger, K., Bushman, F. D., DeSantis, T. Z., Andersen, G. L., and Knight, R. (2010a). PyNAST: a flexible tool for aligning sequences to a template alignment. Bioinformatics 26, 266–267. doi: 10.1093/bioinformatics/btp636
Caporaso, J. G., Kuczynski, J., Stombaugh, J., Bittinger, K., Bushman, F. D., Costello, E. K., et al. (2010b). QIIME allows analysis of high-throughput community sequencing data. Nat. Methods 7, 335–336. doi: 10.1038/nmeth.f.303
Caporaso, J. G., Lauber, C. L., Walters, W. A., Berg-Lyons, D., Huntley, J., Fierer, N., et al. (2012). Ultra-high-throughput microbial community analysis on the Illumina HiSeq and MiSeq platforms. ISME J. 6, 1621–1624. doi: 10.1038/ismej.2012.8
Danielsson, R., Dicksved, J., Sun, L., Gonda, H., Müller, B., Schnürer, A., et al. (2017). Methane production in dairy cows correlates with rumen methanogenic and bacterial community structure. Front. Microbiol. 8:226. doi: 10.3389/fmicb.2017.00226
Daquiado, A. R., Cho, K. M., Kim, T. Y., Kim, S. C., Chang, H. H., and Lee, Y. B. (2014). Methanogenic archaea diversity in Hanwoo (Bos taurus coreanae) rumen fluid, rectal dung, and barn floor manure using a culture-independent method based on mcrA gene sequences. Anaerobe 27, 77–81. doi: 10.1016/j.anaerobe.2014.01.008
de Oliveira, M. N., Jewell, K. A., Freitas, F. S., Benjamin, L. A., Tótola, M. R., Borges, A. C., et al. (2013). Characterizing the microbiota across the gastrointestinal tract of a Brazilian Nelore steer. Vet. Microbiol. 164, 307–314. doi: 10.1016/j.vetmic.2013.02.013
Deng, Y. F., Wang, Y. J., Zou, Y., Azarfar, A., Wei, X. L., Ji, S. K., et al. (2017). Influence of dairy by-product waste milk on the microbiomes of different gastrointestinal tract components in pre-weaned dairy calves. Sci. Rep. 7:42689. doi: 10.1038/srep42689
Dowd, S. E., Callaway, T. R., Wolcott, R. D., Sun, Y., McKeehan, T., Hagevoort, R. G., et al. (2008). Evaluation of the bacterial diversity in the feces of cattle using 16S rDNA bacterial tag-encoded FLX amplicon pyrosequencing (bTEFAP). BMC Microbiol. 8:125. doi: 10.1186/1471-2180-8-125
Durand, G., Afouda, P., Raoult, D., and Dubourg, G. (2017). “Intestinimonas massiliensis” sp. nov, a new bacterium isolated from human gut. New Microbes New Infect 15, 1–2. doi: 10.1016/j.nmni.2016.09.014
Durso, L. M., Wells, J. E., Harhay, G. P., Rice, W. C., Kuehn, L., Bono, J. L., et al. (2012). Comparison of bacterial communities in faeces of beef cattle fed diets containing corn and wet distillers' grain with solubles. Lett. Appl. Microbiol. 55, 109–114. doi: 10.1111/j.1472-765X.2012.03265.x
Edgar, R. C. (2013). UPARSE: highly accurate OTU sequences from microbial amplicon reads. Nat. Methods 10, 996–998. doi: 10.1038/nmeth.2604
Guo, X., Li, S., Zhang, J., Wu, F., Li, X., Wu, D., et al. (2017). Genome sequencing of 39 Akkermansia muciniphila isolates reveals its population structure, genomic and functional diverisity, and global distribution in mammalian gut microbiotas. BMC Genomics 18:800. doi: 10.1186/s12864-017-4195-3
Henderson, G., Cox, F., Ganesh, S., Jonker, A., Young, W., Global Rumen Census, C., et al. (2015). Rumen microbial community composition varies with diet and host, but a core microbiome is found across a wide geographical range. Sci. Rep. 5:14567. doi: 10.1038/srep14567
Holman, D. B., Hao, X., Topp, E., Yang, H. E., and Alexander, T. W. (2016). Effect of co-composting cattle manure with construction and demolition waste on the archaeal, bacterial, and fungal microbiota, and on antimicrobial resistance determinants. PLoS ONE 11:e0157539. doi: 10.1371/journal.pone.0157539
Hua, C., Tian, J., Tian, P., Cong, R., Luo, Y., Geng, Y., et al. (2017). Feeding a high concentration diet induces unhealthy alterations in the composition and metabolism of ruminal microbiota and host response in a goat model. Front. Microbiol. 8:138. doi: 10.3389/fmicb.2017.00138
Ji, S., Jiang, T., Yan, H., Guo, C., Liu, J., Su, H., et al. (2018). Ecological restoration of antibiotic-disturbed gastrointestinal microbiota in foregut and hindgut of cows. Front. Cell. Infect. Microbiol. 8:79. doi: 10.3389/fcimb.2018.00079
Jin, D., Kang, K., Wang, H., Wang, Z., Xue, B., Wang, L., et al. (2017). Effects of dietary supplementation of active dried yeast on fecal methanogenic archaea diversity in dairy cows. Anaerobe 44, 78–86. doi: 10.1016/j.anaerobe.2017.02.007
Johnson, K. A., and Johnson, D. E. (1995). Methane emissions from cattle. J. Anim. Sci. 73, 2483–2492. doi: 10.2527/1995.7382483x
Kim, M., Kim, J., Kuehn, L. A., Bono, J. L., Berry, E. D., Kalchayanand, N., et al. (2014). Investigation of bacterial diversity in the feces of cattle fed different diets. J. Anim. Sci. 92, 683–694. doi: 10.2527/jas.2013-6841
Klein-Jöbstl, D., Schornsteiner, E., Mann, E., Wagner, M., Drillich, M., and Schmitz-Esser, S. (2014). Pyrosequencing reveals diverse fecal microbiota in Simmental calves during early development. Front. Microbiol. 5:622. doi: 10.3389/fmicb.2014.00622
Knapp, J. R., Laur, G. L., Vadas, P. A., Weiss, W. P., and Tricarico, J. M. (2014). Invited review: enteric methane in dairy cattle production: quantifying the opportunities and impact of reducing emissions. J. Dairy Sci. 97, 3231–3261. doi: 10.3168/jds.2013-7234
Kopecný, J., Hodrová, B., and Stewart, C. S. (1996). The effect of rumen chitinolytic bacteria on cellulolytic anaerobic fungi. Lett. Appl. Microbiol. 23, 199–202. doi: 10.1111/j.1472-765X.1996.tb00064.x
Kumar, S., Indugu, N., Vecchiarelli, B., and Pitta, D. W. (2015). Associative patterns among anaerobic fungi, methanogenic archaea, and bacterial communities in response to changes in diet and age in the rumen of dairy cows. Front. Microbiol. 6:781. doi: 10.3389/fmicb.2015.00781
Lascano, G. J., Koch, L. E., and Heinrichs, A. J. (2016). Precision-feeding dairy heifers a high rumen-degradable protein diet with different proportions of dietary fiber and forage-to-concentrate ratios. J. Dairy Sci. 99, 7175–7190. doi: 10.3168/jds.2016-11190
Lee, J. E., Lee, S., Sung, J., and Ko, G. (2011). Analysis of human and animal fecal microbiota for microbial source tracking. ISME J. 5, 362–365. doi: 10.1038/ismej.2010.120
Ley, R. E., Turnbaugh, P. J., Klein, S., and Gordon, J. I. (2006). Human gut microbes associated with obesity. Nature 444, 1022–1023. doi: 10.1038/4441022a
Li, S., Khafipour, E., Krause, D. O., Kroeker, A., Rodriguez-Lecompte, J. C., Gozho, G. N., et al. (2012). Effects of subacute ruminal acidosis challenges on fermentation and endotoxins in the rumen and hindgut of dairy cows. J. Dairy Sci. 95, 294–303. doi: 10.3168/jds.2011-4447
Li, Y., Meng, Q., Zhou, B., and Zhou, Z. (2017). Effect of ensiled mulberry leaves and sun-dried mulberry fruit pomace on the fecal bacterial community composition in finishing steers. BMC Microbiol. 17:97. doi: 10.1186/s12866-017-1011-9
Liu, J., Zhang, M., Xue, C., Zhu, W., and Mao, S. (2016). Characterization and comparison of the temporal dynamics of ruminal bacterial microbiota colonizing rice straw and alfalfa hay within ruminants. J. Dairy Sci. 99, 9668–9681. doi: 10.3168/jds.2016-11398
Lukas, M., Südekum, K. H., Rave, G., Friedel, K., and Susenbeth, A. (2005). Relationship between fecal crude protein concentration and diet organic matter digestibility in cattle. J. Anim. Sci. 83, 1332–1344. doi: 10.2527/2005.8361332x
Mao, S., Zhang, M., Liu, J., and Zhu, W. (2015). Characterising the bacterial microbiota across the gastrointestinal tracts of dairy cattle: membership and potential function. Sci. Rep. 5:16116. doi: 10.1038/srep16116
Mao, S., Zhang, R., Wang, D., and Zhu, W. (2012). The diversity of the fecal bacterial community and its relationship with the concentration of volatile fatty acids in the feces during subacute rumen acidosis in dairy cows. BMC Vet. Res. 8:237. doi: 10.1186/1746-6148-8-237
Meale, S. J., Li, S., Azevedo, P., Derakhshani, H., Plaizier, J. C., Khafipour, E., et al. (2016). Development of ruminal and fecal microbiomes are affected by weaning but not weaning strategy in dairy calves. Front. Microbiol. 7:582. doi: 10.3389/fmicb.2016.00582
Moody, M. L., Zanton, G. I., Daubert, J. M., and Heinrichs, A. J. (2007). Nutrient utilization of differing forage-to-concentrate ratios by growing Holstein heifers. J. Dairy Sci. 90, 5580–5586. doi: 10.3168/jds.2007-0570
Nathani, N. M., Patel, A. K., Mootapally, C. S., Reddy, B., Shah, S. V., Lunagaria, P. M., et al. (2015). Effect of roughage on rumen microbiota composition in the efficient feed converter and sturdy Indian Jaffrabadi buffalo (Bubalus bubalis). BMC Genomics 16:1116. doi: 10.1186/s12864-015-2340-4
Ozutsumi, Y., Hayashi, H., Sakamoto, M., Itabashi, H., and Benno, Y. (2005). Culture-independent analysis of fecal microbiota in cattle. Biosci. Biotechnol. Biochem. 69, 1793–1797. doi: 10.1271/bbb.69.1793
Patra, A. K., and Yu, Z. (2015). Essential oils affect populations of some rumen bacteria in vitro as revealed by microarray (RumenBactArray) analysis. Front. Microbiol. 6:297 doi: 10.3389/fmicb.2015.00297
Peng, L., He, Z., Chen, W., Holzman, I. R., and Lin, J. (2007). Effects of butyrate on intestinal barrier function in a Caco-2 cell monolayer model of intestinal barrier. Pediatr. Res. 61, 37–41. doi: 10.1203/01.pdr.0000250014.92242.f3
Plaizier, J. C., Li, S., Danscher, A. M., Derakshani, H., Andersen, P. H., and Khafipour, E. (2017). Changes in microbiota in rumen digesta and feces due to a grain-based Subacute Ruminal Acidosis (SARA) challenge. Microb. Ecol. 74, 485–495. doi: 10.1007/s00248-017-0940-z
Plaizier, J. C., Li, S., Tun, H. M., and Khafipour, E. (2016). Nutritional Models of Experimentally-Induced Subacute Ruminal Acidosis (SARA) differ in their impact on rumen and hindgut bacterial communities in dairy cows. Front. Microbiol. 7, 2128. doi: 10.3389/fmicb.2016.02128
Popova, M., McGovern, E., McCabe, M. S., Martin, C., Doreau, M., Arbre, M., et al. (2017). The structural and functional capacity of ruminal and cecal microbiota in growing cattle was unaffected by dietary supplementation of linseed oil and nitrate. Front. Microbiol. 8:937. doi: 10.3389/fmicb.2017.00937
Pruesse, E., Quast, C., Knittel, K., Fuchs, B. M., Ludwig, W., Peplies, J., et al. (2007). SILVA: a comprehensive online resource for quality checked and aligned ribosomal RNA sequence data compatible with ARB. Nucleic Acids Res. 35, 7188–7196. doi: 10.1093/nar/gkm864
Pryde, S. E., Duncan, S. H., Hold, G. L., Stewart, C. S., and Flint, H. J. (2002). The microbiology of butyrate formation in the human colon. FEMS Microbiol. Lett. 217, 133–139. doi: 10.1111/j.1574-6968.2002.tb11467.x
R Core Team (2014). R: A Language and Environment for Statistical Computing. R Foundation for Statistical Computing. Vienna: Available online at: online at: http://www.R-project.org/
Rajilić-Stojanović, M., and de Vos, W. M. (2014). The first 1000 cultured species of the human gastrointestinal microbiota. FEMS Microbiol. Rev. 38, 996–1047. doi: 10.1111/1574-6976.12075
Rice, W. C., Galyean, M. L., Cox, S. B., Dowd, S. E., and Cole, N. A. (2012). Influence of wet distillers grains diets on beef cattle fecal bacterial community structure. BMC Microbiol. 12:25. doi: 10.1186/1471-2180-12-25
Russell, J. B., and Rychlik, J. L. (2001). Factors that alter rumen microbial ecology. Science 292, 1119–1122. doi: 10.1126/science.1058830
Schloss, P. D., Westcott, S. L., Ryabin, T., Hall, J. R., Hartmann, M., Hollister, E. B., et al. (2009). Introducing mothur: open-source, platform-independent, community-supported software for describing and comparing microbial communities. Appl. Environ. Microbiol. 75, 7537–7541. doi: 10.1128/AEM.01541-09
Schneeberger, M., Everard, A., Gómez-Valadés, A. G., Matamoros, S., Ramírez, S., Delzenne, N. M., et al. (2015). Akkermansia muciniphila inversely correlates with the onset of inflammation, altered adipose tissue metabolism and metabolic disorders during obesity in mice. Sci. Rep. 5:16643. doi: 10.1038/srep16643
Shanks, O. C., Kelty, C. A., Archibeque, S., Jenkins, M., Newton, R. J., McLellan, S. L., et al. (2011). Community structures of fecal bacteria in cattle from different animal feeding operations. Appl. Environ. Microbiol. 77, 2992–3001. doi: 10.1128/AEM.02988-10
Shen, J., Liu, Z., Yu, Z., and Zhu, W. (2017). Monensin and nisin affect rumen fermentation and microbiota differently in vitro. Front. Microbiol. 8:1111. doi: 10.3389/fmicb.2017.01111
Shin, N. R., Lee, J. C., Lee, H. Y., Kim, M. S., Whon, T. W., Lee, M. S., et al. (2014). An increase in the Akkermansia spp. population induced by metformin treatment improves glucose homeostasis in diet-induced obese mice. Gut 63, 727–735. doi: 10.1136/gutjnl-2012-303839
Suarez-Mena, F. X., Lascano, G. J., Rico, D. E., and Heinrichs, A. J. (2015). Effect of forage level and replacing canola meal with dry distillers grains with solubles in precision-fed heifer diets: digestibility and rumen fermentation. J. Dairy Sci. 98, 8054–8065. doi: 10.3168/jds.2015-9636
Sun, W., Qian, X., Gu, J., Wang, X. J., Zhang, L., and Guo, A. Y. (2017). Mechanisms and effects of arsanilic acid on antibiotic resistance genes and microbial communities during pig manure digestion. Bioresour. Technol. 234, 217–223. doi: 10.1016/j.biortech.2017.03.025
Thornton, P. K. (2010). Livestock production: recent trends, future prospects. Philos. Trans. R. Soc. Lond. B. Biol. Sci. 365, 2853–2867. doi: 10.1098/rstb.2010.0134
Turnbaugh, P. J., Ley, R. E., Mahowald, M. A., Magrini, V., Mardis, E. R., and Gordon, J. I. (2006). An obesity-associated gut microbiome with increased capacity for energy harvest. Nature 444, 1027–1031. doi: 10.1038/nature05414
Van Soest, P. J., Robertson, J. B., and Lewis, B. A. (1991). Methods for dietary fiber, neutral detergent fiber, and nonstarch polysaccharides in relation to animal nutrition. J. Dairy Sci. 74, 3583–3597. doi: 10.3168/jds.S0022-0302(91)78551-2
Wang, C. J., Tas, B. M., Glindemann, T., Rave, G., Schmidt, L., Weissbach, F., et al. (2009). Fecal crude protein content as an estimate for the digestibility of forage in grazing sheep. Anim. Feed Sci. Tech. 149, 199–208. doi: 10.1016/j.anifeedsci.2008.06.005
Wei, M. M., Zhang, R. B., Wang, Y. G., Ji, H. G., Zheng, J., Chen, X. H., et al. (2013). Microbial community structure and diversity in deep-sea hydrothermal vent sediments along the Eastern Lau Spreading Centre. Acta Oceanol. Sin. 32, 42–51. doi: 10.1007/s13131-013-0276-6
Ye, H., Liu, J., Feng, P., Zhu, W., and Mao, S. (2016). Grain-rich diets altered the colonic fermentation and mucosa-associated bacterial communities and induced mucosal injuries in goats. Sci. Rep. 6:20329. doi: 10.1038/srep20329
Zanton, G. I., and Heinrichs, A. J. (2009). Digestion and nitrogen utilization in dairy heifers limit-fed a low or high forage ration at four levels of nitrogen intake. J. Dairy Sci. 92, 2078–2094. doi: 10.3168/jds.2008-1712
Zanton, G. I., and Heinrichs, A. J. (2016). Efficiency and rumen responses in younger and older Holstein heifers limit-fed diets of differing energy density. J. Dairy Sci. 99, 2825–2836. doi: 10.3168/jds.2015-10316
Zhang, J., Shi, H., Wang, Y., Li, S., Cao, Z., Ji, S., et al. (2017). Effect of dietary forage to concentrate ratios on dynamic profile changes and interactions of ruminal microbiota and metabolites in holstein heifers. Front. Microbiol. 8:2206. doi: 10.3389/fmicb.2017.02206
Zhang, J., Shi, H., Wang, Y., Li, S., Zhang, H., Cao, Z., et al. (2018). Effects of limit-feeding diets with different forage-to-concentrate ratios on nutrient intake, rumination, ruminal fermentation, digestibility, blood parameters and growth in Holstein heifers. Anim. Sci. J. 89, 527–536. doi: 10.1111/asj.12959
Zhou, M., Hernandez-Sanabria, E., and Guan, L. L. (2009). Assessment of the microbial ecology of ruminal methanogens in cattle with different feed efficiencies. Appl. Environ. Microbiol. 75, 6524–6533. doi: 10.1128/AEM.02815-08
Keywords: bacteria, archaea, heifer, forage to concentrate ratio, feces
Citation: Zhang J, Shi H, Wang Y, Cao Z, Yang H and Li S (2018) Effect of Limit-Fed Diets With Different Forage to Concentrate Ratios on Fecal Bacterial and Archaeal Community Composition in Holstein Heifers. Front. Microbiol. 9:976. doi: 10.3389/fmicb.2018.00976
Received: 29 January 2018; Accepted: 25 April 2018;
Published: 15 May 2018.
Edited by:
Antonio Faciola, University of Florida, United StatesReviewed by:
Paul James Weimer, United States Department of Agriculture, United StatesElie Jami, Agricultural Research Organization (Israel), Israel
Copyright © 2018 Zhang, Shi, Wang, Cao, Yang and Li. This is an open-access article distributed under the terms of the Creative Commons Attribution License (CC BY). The use, distribution or reproduction in other forums is permitted, provided the original author(s) and the copyright owner are credited and that the original publication in this journal is cited, in accordance with accepted academic practice. No use, distribution or reproduction is permitted which does not comply with these terms.
*Correspondence: Shengli Li, lisheng0677@163.com