An Agent-Based Approach to Integrate Human Dynamics Into Disaster Risk Management
- 1Center of Emergency Management, Chongqing School of Public Service, Chongqing, China
- 2Key Laboratory of Mountain Hazards and Earth Surface Processes, Institute of Mountain Hazards and Environment, Chinese Academy of Sciences, Chengdu, China
- 3China-Pakistan Joint Research Center on Earth Sciences, CAS-HEC, Islamabad, Pakistan
- 4Key Laboratory of Environmental Change and Natural Disaster, Ministry of Education, Beijing Normal University, Beijing, China
- 5School of National Safety and Emergency Management, Beijing Normal University, Beijing, China
- 6Key Laboratory of Land Surface System and Simulation, Institute of Geographic Sciences and Natural Resources Research, Chinese Academy of Sciences, Beijing, China
- 7National Disaster Reduction Center of China, Ministry of Emergency Management, Beijing, China
Disaster risk management (DRM) is the application of disaster risk reduction policies and strategies to reduce existing disaster risk and manage residual risk. However, due to dynamic human factors, it is challenging to depict and assess the effectiveness of DRM measures, and their implementation usually lacks a sufficient evidence-based evaluation process. Therefore, this study developed an agent-based model to integrate dynamic human behaviors into the DRM measures and evaluated their effectiveness in casualty reduction. The model was calibrated to simulate the debris flow event at Longchi town, China in 13 August 2010. The early warning system (EWS) and related DRM measures were taken as examples. The effectiveness of different DRM measures was quantitated by comparing the number of potential casualties. The main findings were: 1) EWS was very effective for community-based DRM as it could significantly decrease the average casualties by 30%. 2) Credibility of EWS was critical to its effectiveness. Less credible EWS might reduce its effectiveness by 9%. 3) EWS could be supplemented by other measures to further reduce casualties by 6%. 4) The downside effects of other DRM measures to EWS might exist and reduce its effectiveness by up to 5%. This study put forward an evidence-based approach to help policymakers select more cost-effective DRM measure, especially in the less developed countries where the available resources for DRM are limited.
Introduction
Disasters can bring devastating loss of life and injuries as well as damages to critical lifelines and infrastructures. Nowadays, disasters are more threatening than we ever expected to the social, environmental, and economic components of sustainable development, and subsequently, there is an increasing demand for taking ambitious actions to disaster risk management (DRM). Communities in mountainous areas are prone to natural hazards due to the complex geological settings that foster favorable conditions to mountain hazards (Cui et al., 2021). Governments are liable to ensure the safety of residents, critical lifelines, and infrastructure. DRM measures have been employed to alleviate the extent of suffering and hardships caused by hazards. However, due to the variety of community settings regarding residents and facilities, DRM measures would not effectively reduce disaster risks without considering these factors. Instead, when implemented to the community level, the DRM needs to precisely tailor its measures for the locals, including the environmental and social conditions of communities and individual differences of their residents.
Take the early warning system (EWS) as an example. As one of the essential DRM measures, EWS is widely utilized in many cases worldwide to provide the lead time for residents to evacuate from disasters (Alcántara-Ayala and Garnica, 2013). The United Nations Office for Disaster Risk Reduction (UNDRR) defined EWS as “the set of capacities needed to generate and disseminate timely and meaningful warning information to enable individuals, communities and organizations threatened by a hazard to prepare and to act appropriately and insufficient time to reduce the possibility of harm or loss” (UNISDR, 2009, p5). Smith (1996) divided the EW process into three inter-related stages: evaluation/forecasting, warning/dissemination, and response. Each stage involves many natural and human aspects that can affect the effectiveness of the EWS. At the community level, its effectiveness is primarily associated with the number of casualties prevented. To improve the effectiveness of EWS, many of current studies focused on natural science and utilized new technologies and scientific advances to improve its accuracy and timeliness, such as geological knowledge and risk scenarios (Intrieri et al., 2012), UAV (unmanned aerial vehicle) and InSAR (Interferometric Synthetic Aperture Radar) (Mantovani et al., 2016; Peppa et al., 2016; Mateos et al., 2017) and rarely considered the influence of human behaviors. As human is also an essential factor constraining the effectiveness of EWS (Twigg, 2003; Intrieri et al., 2012), it is necessary to consider the individual differences in community vulnerability in order to achieve more scientific and efficient DRM.
Human are complex creatures, and the behaviors of each person vary objectively and subjectively. In the context of disasters, their characteristics, such as gender (Bateman and Edwards, 2002), risk perception and awareness (Baker, 1991; Gissing et al., 2008; Smith and McCarty, 2009), social and physical conditions (Baker, 1991; Şahin et al., 2019), experience and knowledge (Nakanishi et al., 2019), can result in various emergency behaviors during disasters events and affect the effectiveness of EWS. These dynamics of human factors make it challenging to simulate and assess the effectiveness of EWS; thus, many models were neglected with an absence of incorporating human dynamic decision-making process (Schwarz and Ernst, 2009; Groeneveld et al., 2017). As a primary tool for modeling individual decision-making and complex interactions (Matthews et al., 2007), the agent-based modeling (ABM) approach offers a quantitative, theoretical, and mechanistic approach to explain and predict the typical findings in disaster risk research, especially when humans are involved during the DRM process.
Therefore, to extend the traditional disaster risk model, this study adopted the ABM approach to relating DRM measures and human behaviors during disaster events. The objectives of this paper include: 1) development of a model to simulate human behaviors and visualize their interactions with each other and the environment during disasters; 2) assessment of the effectiveness and influential factors of EWS and related DRM measures in casualty reduction and 3) provision of scientific-based evidence for better prioritization during DRM and resilience building in disaster-prone areas by examining the effectiveness of DRM measures.
Study Area
Longchi town (31°N, 103°E), Sichuan Province, is located in the southwest of China (Figure 1), approximately 80 km to the northwest of Chengdu. In the 2008 Wenchuan Earthquake, as it neighbored the epicenter (Yingxiu) with a straight-line distance of 3 km, Longchi was hit with 36 people dead, 17 missing, and 1,558 injured.
Afterward, due to post-earthquake fractured rock and loose soil on steep slopes, Longchi repeatedly suffered mass movement processes (Zhang et al., 2019). The debris flow that occurred on August 13th, 2010 (the 813 debris flow) was one of the most destructive debris flows. The 813 debris flow was a flash flood-debris flow disaster chain, and through more than 50 gullies simultaneously occurred debris flows with a total volume of 7.78 × 106 m3 (Xu et al., 2012). This event caused 495 casualties (about 3,000 population in total) and substantial economic losses. Almost all the houses and roads near the river and the gullies were destroyed.
Method and Data
The ABM (Agent-based modeling) approach is a modeling method in which individuals (e.g., people, animals, and cells) and their interaction with each other and their environment are explicitly represented. It includes conceptions from mathematics, physics, biology, sociology, and other disciplines. One feature of the complex system theory was that the global phenomenon came from the macroscopic emergence of micro-interactions (Green and Sadedin, 2005). In disaster event modeling, the challenging task was to predict people’s behaviors from many factors affecting human decisions. Studies in disaster risk management using ABM gained traction and showed that it was possible to integrate scientific theories on human behavior and perception into disaster risk reduction. (Haer et al., 2016a; Haer et al., 2016b; Jenkins et al., 2017; Aerts et al., 2018). The ABM could simulate individual behavior, where agents acted in their interests with decision rules for different adaptation actions (Aerts et al., 2018). The current study adopted the ABM method to consider human risk perception and dynamic behaviors during the 813 debris flow disaster events and generate the potential casualty. The changes in casualties were used to reflect the effectiveness of the EWS and related DRM measures.
Model Components
This study developed the ABM model with two types of components, model environment, and agent. The model environment created the foundation for the agents to move and interact. In this case, the environment was reconstructed based on the physical and social conditions of the 813 debris flow event. There are four types of agents in this model, i.e., disaster, resident, house, shelter. Each type of agent was assigned with its own attributes that would formulate the agents’ behaviors rules.
Model Environment
This model used GIS and RS data, including topography, the river system, village, and building locations to depict Longchi town before the 813 debris flow. The digital elevation model (DEM) of Longchi, at a resolution of 5 m × 5 m, was imported to the ABM modeling platform from ArcGIS. Debris flow volumes during this event were calculated based on the precipitation records.
Agent and Their Attributes
This ABM model included four types of agents: residents, disasters (debris flow), houses, and disaster shelters, among which residents and disasters agents could move and interact with others. Each agent possessed different attributes to simulate the disaster event by encapsulating the psychology, sociology, geography, and disaster movement mechanism. As shown in Table 1, each type of agent was characterized by several attributes.
Agent Behaviors Design
Behavior rules were fundamental to the ABM model simulation. This study defined the behavior rules based on the data collected through field investigation and semi-structured interviews, which included risk perception, willingness to evacuation, emergency choice, knowledge level on disasters, numbers of disaster trainings attended, conditions of DRM measures.
For the design of the semi-structured interview, we conducted a focus group interview with 13 residents to gain an in-depth understanding of their perception and behaviors on disasters and related DRM measures. The follow-up interviews were then conducted in all villages in Longchi with 54 households to investigate their mental condition and behaviors before and during the 813 debris flow. This survey covered nearly 50% of current resident households, widely distributed in locations, professions, gender, and age. Collected data have been processed in SPSS to discover the general behavioral rules for use in the ABM model.
Resident Agent: To Simulate Human Behaviors During the Disaster Event
Each resident was displayed as an autonomous agent in the system and had physical and mental attributes that could influence his or her behaviors during disaster events. In this model, two factors could affect human behaviors: evacuation willingness (EW) and evacuation strategy (ES). The evacuation willingness determined whether the resident agents started to evacuate. Residents with high evacuation willingness were set to evacuate immediately when they received alerts. In contrast, those with lower willingness would hesitate and start to move when the disaster agent was within a visible distance (40 m). Once the resident agent started to evacuate, evacuation strategies would determine how the resident agent would move. There were two types of evacuation strategies in this model: normal and panic. For normal evacuation strategies, resident agents would move toward disaster shelters or places with higher elevations. Otherwise, they would move in any direction as long as it was away from the disaster agent.
This study obtained the probabilistic correlation between the attributes of resident agents (knowledge level, risk perception, experience, training) and EW/ES using the cross-tabulation analysis of the interview results in SPSS (Kamakura and Wedel, 1997). Based on the knowledge level and risk perception, each resident agent was assigned a probability to start evacuation (Table 2). At the same time, each of the resident agents was set to obtain a probability of conducting a normal or panic strategy (Table 3) based on knowledge level and times of training.
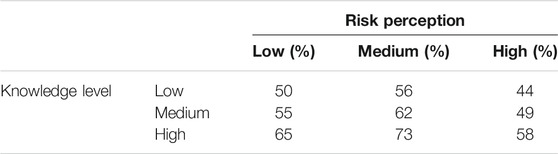
TABLE 2. Evacuation willingness and evacuation strategy probability: The probability of starting evacuation.
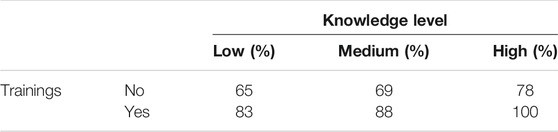
TABLE 3. Evacuation willingness and evacuation strategy probability: The probability of the normal strategy.
Disaster Agent: To Simulate Debris Flow Movement
Disaster agents in this ABM model represented the debris flow disaster, and their attributes included gully locations and debris flow volumes. The disaster agent will move from the upstream of the debris flow gully to the deposition area. The number of disaster agents was calculated based on the debris flow volume calculated for that specific debris flow gully. The gully locations in this model were determined based on the field investigation after the 813 debris flow (Chang et al., 2014).
Data Preparation and Simulation
Resident Data
Each resident was displayed as an autonomous agent in the system and had physical and mental attributes that could influence his or her behaviors during disaster events. These attributes of resident agents (Table 4) were derived from the Sixth National Population Census of the People’s Republic of China (National Bureau of Statistics of China, 2011) and the interviews. The data management and analysis were performed by using SPSS. The analysis results formed the physical and mental attributes of each resident agent in the ABM world (Table 4).
Disaster Data
Hourly precipitation data from the monitoring station at Yingxiu town (Figure 2), located 3 km to Longchi, were used to calculate the debris flow volume. Each gully generated the initial disaster agents based on the debris flow volume calculated (Table 5).
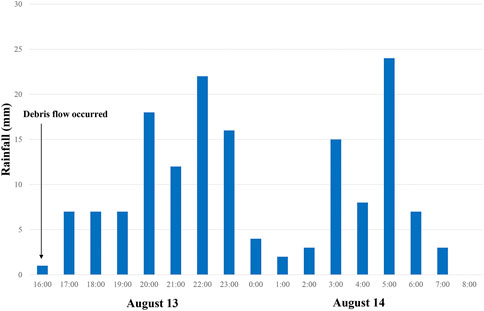
FIGURE 2. Hourly precipitation in Yingxiu town during the 813 debris flow (Xu et al., 2012).
Simulation
The 813 debris flow lasted for 17 h, from 4.00 PM on August 13th to 8.00 AM on August 14th. The model was designed as one tick in the ABM world equaled 15 min in the real world and was run for 76 ticks for each scenario simulation. A total of 18 different scenarios had been simulated by this study (Table 6). Concerning human dynamics in emergency response, each scenario simulation was set to be repeated 50 times. During simulations, all types of agents were allowed to interact with the pre-defined behavior rules (Figure 3). The interaction process simulated the 813 debris flow event, and the average number of casualties was used to evaluate the effectiveness of each DRM measure.
The disaster agents in debris flow gullies moved based on the topographic condition. As ticks went by in the ABM world, disaster agents were set to interact with patches around and move to the lower patch, and the water level of the patch was calculated based on the volume or number of disaster agents. Disaster agents would keep moving for 76 ticks until they reached the lowest patch or move outside the calculation boundary. Once resident agents encountered disaster agents, this resident agent would be counted as a casualty (Figure 4).
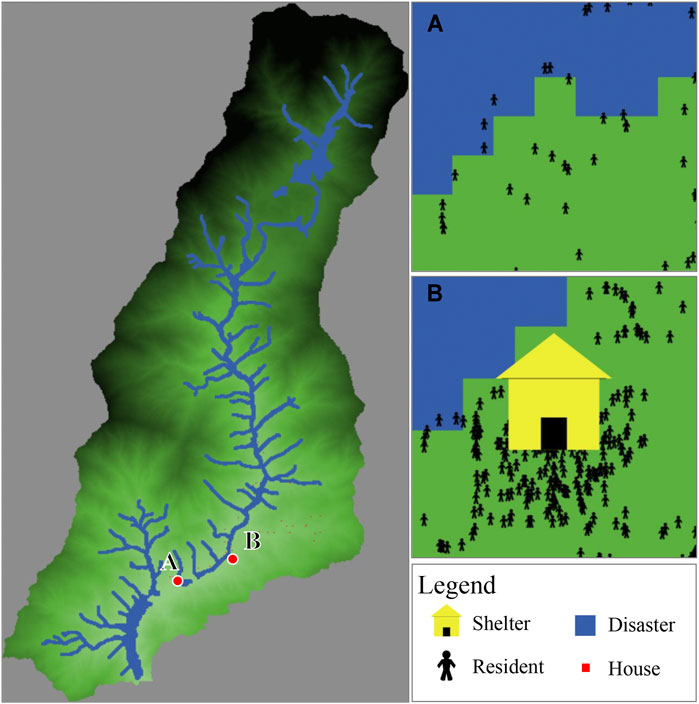
FIGURE 4. Simulation Case No. 10: (A) Residents encountered disaster (man in a blue patch); (B) Residents reach the shelter.
Different DRM measures, including EWS, disaster shelters, and incentive plans, were designed in the ABM model to study their influence on the potential casualty. The EWS would affect the initial time of resident evacuation. In 2010 when the 813 debris flow occurred, there was no EWS available. In this case, the model only allowed resident agents to start the initial emergency response process when the disaster agent appeared in their sight. On the contrary, when the EWS became available, the warning was released 2 h (8 ticks) before the debris flow occurred. It was assumed that all residents could receive alerts and immediately start the emergency response based on their behavior rules. Shelter and incentive plans were set to affect their emergency response. Resident agents would decide which destination they move to base on their perception of the disaster shelter. The incentive plans were to help raise the evacuation willingness of residents after they received disaster warnings. The effectiveness of disaster shelters and incentive plans in casualty reduction was generated assuming that the EWS was available in this model.
Results
Different DRM scenarios were simulated by the proposed model (Table 6). Each scenario was repeated by 50 times, and the average number of casualties was calculated. The case of 813 events without EWS (Case No.1 in Table 6) represented the actual disaster situation in 2013 and generated the number of potential casualties in this scenario (481). In addition, EWS were often implemented along with other DRM measures to supplement each other. In this paper, two types of DRM measures were adopted to explore their effectiveness through the change in casualty, which was 1) provide disaster shelters and 2) set up incentive plans for evacuation. The potential casualties of each scenario are summarized in Table 6 and elaborated in the following sections.
DRM Measure 1: Early Warning System
This study first explored the effect of installing EWS as the DRM measures (Figure 5A). The average casualties significantly decreased from 481 to 337, by 30% compared with no EWS was available. As practices have proved that the EWS could not always bring about early actions (Zaki et al., 2019), this study further explored how the credibility of the EWS can affect the effectiveness of the systems. The 5-point Likert-Scale questions were used to obtain the public perception of the credibility of EWS, among which point 1 was the least credible, and point 5 was the most credible. Figure 5B showed that with the increase of EWS credibility (1–5), casualties dropped from 368 to 321. It can be seen that the public perception of the EWS credibility is influential to its effectiveness. To quantify the influence of other DRM measures in this section, 337 (casualty from case 2 in Table 6) was set as the baseline of assessment. When the public was very satisfied (point 5 on Figure 5B) with the credible EWS, casualties could be further reduced by 5%, from 337 to 321. On the contrary, less credible EWS would cause the casualties to increase by 8%, from 337 to 368.
DRM Measure 2: Early Warning System With Disaster Shelter
Disaster shelters provide residents with a safe place during disaster events. Public perception of the shelter design, such as distance to the current location and facilities provided in the shelter, would affect residents’ choices on the evacuation strategy and result in different casualties. The impact of public perception on shelters design was studied from two aspects: distance from home to shelters, and facilities provided in the shelter.
For public perception on the distance, this study divided the distance from their location to the shelter into four degrees: “<10min walking” (very close), “< 30 min walking” (close), “< 60 min walking” (far), and “> 60min walking” (very far). The number of casualties varied in the range of 315–337 (Figure 6A). The lowest casualties 315) occurred when people felt very close to the shelter, with a casualty reduction rate of about 34% compared to no EWS 481) and 6% compared with EWS alone (337). For the public perception of the facilities provided in the disaster shelters, this study categorized the results into four groups: “few facilities” (basic living needs), “some facilities” (living and medical needs), “many facilities” (living, medical needs, and entertainment), and “not sure” (no idea of what facilities were provided). The results showed that casualties varied from 354 to 320 (Figure 6B). Generally, as shelter facilities improved, the potential casualties reduced. The lowest casualties (320) occurred when there were many facilities available in the shelter with a casualty reduction rate of about 33% compared to no EWS (481) and 5% compared with EWS alone (337).
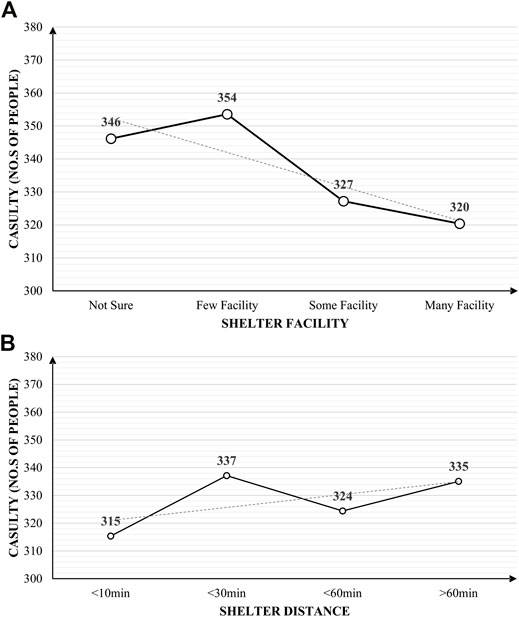
FIGURE 6. Potential casualties with different shelter settings: (A) walking distance from residents to shelters; (B) shelter facilities.
DRM Measure 3: Early Warning and Incentive Plans
The Longchi government set up incentive plans to encourage residents to evacuate after receiving the alert from the EWS. A small number of living expenses was provided to residents who reached the disaster shelter immediately after the EWS. The study found that most respondents showed a positive attitude toward the incentive plan and reported that this measure could attract them to cooperate with the EWS. This study conducted three scenarios where residents were provided with 5 CNY, 20 CNY, and 50 CNY, respectively, and obtained the number of casualties under each scenario.
The results show that when residents were rewarded with 5 CNY, casualties decreased to 330, further reduced 1.84% compared with EWS alone (Figure 7). When rewards increased to 20 CNY and 50 CNY, casualties were 328 and 323, further reduced 1% and 2% compared with when 5 CNY was provided. Although the amount of reward increased dramatically from 5 CNY to 50 CNY, the further reduction of casualties was not significant. Therefore, incentive plans with EWS could help reduce the casualties, but they only worked at a very marginally level.
Discussion
ABM simulates and encapsulates complex systems from the behaviors and interactions of individual agents. One of the main concerns is whether an ABM represents reality well enough to extrapolate into the future. Models are typically calibrated once, using historical data, then projected forward in time to make a prediction (Ward et al., 2016). Some uncertainties in ABM come from dynamics (changing over space and time), stochastic (containing inherent randomness), and unobserved (unseen from the data) conditions of the real system under study (Kieu et al., 2020). The extent of ABM can be validated to reduce the uncertainty through calibration using historical data (Bonabeau, 2002). Therefore, this study adopted the 813 debris flow events data and calibrated the proposed ABM model to best fit the disaster scenario with the local context of Longchi town. However, an agreement between model and data does not always imply that the model accurately describes the processes; it could only indicate that the model is one of several that is plausible to a situation (Papadelis and Flamous, 2019). The model in the current study also suffered from uncertainty such as the population structure, human movement speed during the evacuation, and choices they make when faced with decisions. Studies have found that even a well-calibrated model will diverge from the true state of the underlying system (Clay et al., 2020). In this case, to assure the significance of the conclusion, this study simulated 3,000 entities for each scenario and repeated 50 runs for each scenario. The average of 50 runs was taken as the estimation of the performance measure to reduce the model uncertainty as much as possible.
EWS Was Essential for Community-Based DRM, and Its Credibility Was Critical to Its Effectiveness
EWS is one of the highly-praised DRM measures widely used worldwide. Based on the 813 debris flow simulations, this study found that having EWS could reduce casualties by up to 30%. Since there was no EWS in 2013 during the 813 event, the interview found that many people did not evacuate until they had already faced the debris flow. Many casualties were caused due to a lack of time to evacuate. Thus, EWS can provide residents with lead time for early evacuation and save more lives. However, this study also found that the credibility of EWS would seriously affect its effectiveness (Figure 5), and it could affect the result by 13%. When the public perception of the credibility of EWS was low, people tended not to trust the alerts and not evacuate at all. Therefore, it was necessary to carry out more in-depth scientific research to understand the mechanism of disaster formation and movement to improve the accuracy and credibility of EWS and ultimately improve the effectiveness of DRM.
EWS Could Be Supplemented by Other Measures to Further Reduce Casualties
EWS could be supplemented by other measures to further reduce casualties and improve the effectiveness of DRM. This study explored the effect of DRM measure and found that disaster shelter and incentive plans could further reduce casualties up to 6% and 4%, respectively, compared with EWS alone.
For disaster shelter, its effectiveness depended on the location of shelter and facilities provided in it. Distance between shelter and resident location would affect their willingness to evacuate. When the walking distance from home to the shelters was within 10 min, residents were more willing to evacuate, which resulted in a casualty number at 315. Building shelters closer to residents could raise public evacuation willingness and result in fewer casualties. At the same time, from case No. 12 to No. 15 in Table 6, it can be seen that facilities provided in the shelter also affected its effectiveness by 9%. When the shelter facilities were diversified with living, medical, entertainment facilities, the number of potential casualties could be minimum, while in the case of only basic living being provided, the potential casualties increased.
Since not all residents are willing to evacuate immediately after receiving early warning information, the incentives plan can help them comply with EWS. However, this study showed that increasing the amount of incentive does not effectively reduce casualties further. In this case, residents’ annual income per capita was 8,594 CNY (Dujiangyan Government, 2021). When the incentive increased from 5 CNY to 50 CNY, it could only reduce casualty from 330 to 323, by 2%. Therefore, though the DRM measures could be improved by providing incentive plans for evacuation, the marginal effect was obvious, and raising the allowance could not significantly reduce the casualty.
The Downside Effects of Other DRM Measures to EWS Might Exist
With all kinds of DRM measures, although the potential casualties were all lower than that of the 813 event (Table 6), this study found that there were cases that resulted in more casualties than that of setting up EWS alone. The simulation showed that in some cases, the location and facilities of shelters might constrain the effectiveness of EWS. For example, in the case No.11 and No.13, when shelter distance >60 min or very few facilities were provided in the shelter, there were more casualties (335 and 354 respectively) than that of setting up EWS alone (337). One possible reason could be that the interview showed that when the distance to the shelter was more than 60 min, residents were reluctant to evacuate and tended to walk to nearby “assumed safe places” based on their own judgment, which could lead to potential casualties in the model. In addition, when residents were unsure what kind of facilities were provided or knew there was only basic living, they were more reluctant to evacuate to the shelter or return home from the shelter after evacuation, which caused the increase of potential casualties. Therefore, it was necessary to carefully design DRM measures by considering various human factors in the implementation process to manage their possible downsides effects. Otherwise, spending a large amount of money would, on the contrary, reduce the effectiveness of EWS.
Conclusion
By integrating comprehensive components and mechanisms, the DRM measures are complicated with multiple approaches and numerous influential factors, and therefore it is challenging to quantify the effectiveness. This study developed an ABM evaluation model to consider each resident’s individual differences and simulated their behaviors during the disaster event, and took the EWS as an example of DRM measures to evaluate their effectiveness in casualty reduction.
This study showed that the EWS was an effective tool in community-based DRM, and its credibility was critical to its effectiveness. Credible EWS could reduce the casualties by as much as 33%, from 481 to 321. In addition, when the EWS was supplemented with other measures such as disaster shelter and incentive plan, the casualties could be further reduced by 6%, from 337 to 315. However, its effectiveness was largely affected by the public perception of the design and implementation of those measures. Therefore, when designing disaster shelters, priority should be given to place shelters as close as possible to residential areas. When the resource was adequate to optimize shelter effectiveness, the needs of residents, not only the basic living but also medical care, communications, entertainment, should be considered. To create a more effective incentive plan, resident income should be assessed to provide a design reference. The marginal effect of incentive plans on casualty reduction also should be recognized to avoid wasting resources.
To conclude, human dynamics have a significant impact on the effectiveness of DRM measures. Therefore, stakeholders need to choose proper and effective DRM measures considering local conditions, such as risk perception, education levels, and disaster knowledge. The present study developed a quantitative analytical model to assess the effectiveness of EWS and related DRM measures in terms of casualty reduction. It could provide a tool for stakeholders to develop an evidence-based and risk-informed DRM strategy that best fits the resources.
Data Availability Statement
The raw data supporting the conclusion of this article will be made available by the authors without undue reservation.
Author Contributions
Conceptualization, SW, YL, SY, and PC; Methodology and formal analysis, SW, SY, and YL; Data preparation, WJ, and all authors contributed to writing and editing the manuscript.
Funding
This work was supported by the Key Research Program of Frontier Sciences, CAS (Grant No. QYZDY-SSW-DQC006), National Natural Science Foundation of China (Grant No. 41807509 and 41790433) and the Chinese Academy of Sciences President’s International Fellowship Initiative (Grant No. 2020FYC0004), and Light of West China Program (Grant No. Y9R2140).
Conflict of Interest
The authors declare that the research was conducted in the absence of any commercial or financial relationships that could be construed as a potential conflict of interest.
The reviewer YJ declared a shared affiliation, with no collaboration, with one of the authors, YL, to the handling editor at the time of the review.
Publisher’s Note
All claims expressed in this article are solely those of the authors and do not necessarily represent those of their affiliated organizations, or those of the publisher, the editors and the reviewers. Any product that may be evaluated in this article, or claim that may be made by its manufacturer, is not guaranteed or endorsed by the publisher.
References
Aerts, J. C. J. H., Botzen, W. J., Clarke, K. C., Cutter, S. L., Hall, J. W., Merz, B., et al. (2018). Integrating Human Behaviour Dynamics into Flood Disaster Risk Assessment. Nat. Clim. Change 8 (3), 193–199. doi:10.1038/s41558-018-0085-1
Alcántara-Ayala, I., and Garnica, R. J. (2013). “Landslide Monitoring and Warning Systems in Mexico,” in Landslides: Global Risk Preparedness (Berlin, Heidelberg: Springer), 299–314.
Baker, E. J. (1991). Hurricane Evacuation Behavior. Int. J. Mass Emergencies Disasters 9 (2), 287–310.
Bateman, J. M., and Edwards, B. (2002). Gender and Evacuation: A Closer Look at Why Women Are More Likely to Evacuate for Hurricanes. Nat. Hazards Rev. 3 (3), 107–117. doi:10.1061/(asce)1527-6988(2002)3:3(107)
Bonabeau, E. (2002). Agent-based Modeling: Methods and Techniques for Simulating Human Systems. Proc. Natl. Acad. Sci. 99 (Suppl. 3), 7280–7287. doi:10.1073/pnas.082080899
Chang, M., Tang, C., Zhang, D.-d., and Ma, G. C. (2014). Debris Flow Susceptibility Assessment Using a Probabilistic Approach: A Case Study in the Longchi Area, Sichuan Province, China. J. Mt. Sci. 114, 1001–1014. doi:10.1007/s11629-013-2747-9
Clay, R., Kieu, L.-M., Ward, J. A., Heppenstall, A., and Malleson, N. (2020). “Towards Real-Time Crowd Simulation under Uncertainty Using an Agent-Based Model and an Unscented Kalman Filter,” in Advances in Practical Applications of Agents, Multi-Agent Systems, and Trustworthiness. The PAAMS Collection. PAAMS 2020. Lecture Notes in Computer Science. Editors Y. Demazeau, T. Holvoet, J. Corchado, and S. Costantini (Cham: Springer), 68–79.
Cui, P., Peng, J., Shi, P., Tang, H., Ouyang, C., Zou, Q., et al. (2021). Scientific Challenges of Research on Natural hazard and Disaster Risk. Geogr. Sustainability 2, 216–223. doi:10.1016/j.geosus.2021.09.001
Dujiangyan Government (2021). Data Opening. Available at: http://www.djy.gov.cn/dyjgb_rmzfwz/c135340/sjkf2.shtml (Accessed September 10, 2021).
Gissing, A., Molino, S., Cameron-Smith, C., and Wales, N. S. (2008). How Do You Improve Community Response to Warnings. New South Wales, Australia: State Emergency Service.
Green, D. G., and Sadedin, S. (2005). Interactions Matter-Complexity in Landscapes and Ecosystems. Ecol. Complexity 2 (2), 117–130. doi:10.1016/j.ecocom.2004.11.006
Groeneveld, J., Müller, B., Buchmann, C. M., Dressler, G., Guo, C., Hase, N., et al. (2017). Theoretical Foundations of Human Decision-Making in Agent-Based Land Use Models - A Review. Environ. Model. Softw. 87 (Jan), 39–48. doi:10.1016/j.envsoft.2016.10.008
Haer, T., Botzen, W. J., and Aerts, J. C. J. H. (2016a). Integrating Household Mitigation Behaviour in Food Risk Analysis: an Agent Based Model Approach. Risk Anal. 12740, 1–15. doi:10.1111/risa.12740
Haer, T., Botzen, W. J. W., and Aerts, J. C. J. H. (2016b). The Effectiveness of Flood Risk Communication Strategies and the Influence of Social Networks-Insights from an Agent-Based Model. Environ. Sci. Pol. 60, 44–52. doi:10.1016/j.envsci.2016.03.006
Intrieri, E., Gigli, G., Mugnai, F., Fanti, R., and Casagli, N. (2012). Design and Implementation of a Landslide Early Warning System. Eng. Geology 147-148, 124–136. doi:10.1016/j.enggeo.2012.07.017
Jenkins, K., Surminski, S., Hall, J., and Crick, F. (2017). Assessing Surface Water Flood Risk and Management Strategies under Future Climate Change: Insights from an Agent-Based Model. Sci. Total Environ. 595, 159–168. doi:10.1016/j.scitotenv.2017.03.242
Kamakura, W. A., and Wedel, M. (1997). Statistical Data Fusion for Cross-Tabulation. J. Marketing Res. 34 (4), 485–498. doi:10.1177/002224379703400406
Kieu, L.-M., Malleson, N., and Heppenstall, A. (2020). Dealing with Uncertainty in Agent-Based Models for Short-Term Predictions. R. Soc. Open Sci. 7 (1), 191074. doi:10.1098/RSOS.191074
Mantovani, M., Devoto, S., Piacentini, D., Prampolini, M., Soldati, M., and Pasuto, A. (2016). Advanced SAR Interferometric Analysis to Support Geomorphological Interpretation of Slow-Moving Coastal Landslides (Malta, Mediterranean Sea). Remote Sensing 8 (6), 443. doi:10.3390/rs8060443
Mateos, R. M., Azañón, J. M., Roldán, F. J., Notti, D., Pérez-Peña, V., Galve, J. P., et al. (2017). The Combined Use of PSInSAR and UAV Photogrammetry Techniques for the Analysis of the Kinematics of a Coastal Landslide Affecting an Urban Area (SE Spain). Landslides 14 (2), 743–754. doi:10.1007/s10346-016-0723-5
Matthews, R. B., Gilbert, N. G., Roach, A., Polhill, J. G., and Gotts, N. M. (2007). Agent-based Land-Use Models: a Review of Applications. Landscape Ecol. 22 (10), 1447–1459. doi:10.1007/s10980-007-9135-1
Nakanishi, H., Black, J., and Suenaga, Y. (2019). Investigating the Flood Evacuation Behaviour of Older People: A Case Study of a Rural Town in Japan. Res. Transportation Business Manage. 30, 100376. doi:10.1016/j.rtbm.2019.100376
National Bureau of Statistics of China (2011). Tabulation of the 2010 Population Census of the People's Republic of China. Available at: http://www.stats.gov.cn/tjsj/pcsj/rkpc/6rp/indexch.htm (Accessed April 10, 2020).
Papadelis, S., and Flamos, A. (2019). “An Application of Calibration and Uncertainty Quantification Techniques for Agent-Based Models,” in Understanding Risks and Uncertainties in Energy and Climate Policy. Editors H. Doukas, A. Flamos, and J. Lieu (Cham: Springer), 79–95.
Peppa, M. V., Mills, J. P., Moore, P., Miller, P. E., and Chambers, J. E. (2016). Accuracy Assessment of a Uav-Based Landslide Monitoring System. Int. Arch. Photogramm. Remote Sens. Spat. Inf. Sci. XLI-B5, 895–902. doi:10.5194/isprsarchives-xli-b5-895-2016
Şahin, C., Rokne, J. G., and Alhajj, R. (2019). Human Behavior Modeling for Simulating Evacuation of Buildings during Emergencies, Physica A-statistical Mech. Its Appl. 528, 121432. doi:10.1016/j.physa.2019.121432
Schwarz, N., and Ernst, A. (2009). Agent-based Modeling of the Diffusion of Environmental Innovations - an Empirical Approach. Technol. Forecast. Soc. Change 76, 497–511. doi:10.1016/j.techfore.2008.03.024
Smith, S. K., and McCarty, C. (2009). Fleeing the Storm(s): an Examination of Evacuation Behavior during florida's 2004 hurricane Season. Demography 46 (1), 127–145. doi:10.1353/dem.0.0048
Twigg, J. (2003). “The Human Factor in Early Warnings: Risk Perception and Appropriate Communications,” in Early Warning Systems for Natural Disaster Reduction (Berlin, Heidelberg: Springer), 19–26.
UNISDR (2009). Terminology on Disaster Risk Reduction. Available at: https://www.unisdr.org/files/7817_UNISDRTerminologyEnglish.pdf (Accessed September 13th, 2019).
Ward, J. A., Evans, A. J., and Malleson, N. S. (2016). Dynamic Calibration of Agent-Based Models Using Data Assimilation. R. Soc. Open Sci. 3, 150703. doi:10.1098/rsos.150703
Xu, Q., Zhang, S., Li, W. L., and Van Asch, T. W. J. (2012). The 13 August 2010 Catastrophic Debris Flows after the 2008 Wenchuan Earthquake, China. Nat. Hazards Earth Syst. Sci. 12 (1), 201–216. doi:10.5194/nhess-12-201-2012
Zaki, R., Roffeei, S. N., Hii, Y. L., Yahya, A., Appannan, M., Said, M. A., et al. (2019). Public Perception and Attitude towards Dengue Prevention Activity and Response to Dengue Early Warning in Malaysia. PloS One 14 (2), e0212497. doi:10.1371/journal.pone.0212497
Keywords: disaster risk management, early warning system, agent-based modeling, human dynamics, debris flows
Citation: Wu S, Lei Y, Yang S, Cui P and Jin W (2022) An Agent-Based Approach to Integrate Human Dynamics Into Disaster Risk Management. Front. Earth Sci. 9:818913. doi: 10.3389/feart.2021.818913
Received: 20 November 2021; Accepted: 31 December 2021;
Published: 04 February 2022.
Edited by:
Chong Xu, Ministry of Emergency Management (China), ChinaReviewed by:
Siyuan Ma, China Earthquake Administration, ChinaYuan-Jun Jiang, Institute of Mountain Hazards and Environment (CAS), China
Joern Lauterjung, GFZ German Research Centre for Geosciences, Germany
Copyright © 2022 Wu, Lei, Yang, Cui and Jin. This is an open-access article distributed under the terms of the Creative Commons Attribution License (CC BY). The use, distribution or reproduction in other forums is permitted, provided the original author(s) and the copyright owner(s) are credited and that the original publication in this journal is cited, in accordance with accepted academic practice. No use, distribution or reproduction is permitted which does not comply with these terms.
*Correspondence: Yu Lei, leiyu@imde.ac.cn