Monitoring Long Term Noninvasive Ventilation: Benefits, Caveats and Perspectives
- 1Division of Pulmonary Diseases, Department of Medicine, Geneva University Hospitals, Geneva, Switzerland
- 2Hôpital de La Tour, Centre Cardio-Respiratoire, Geneva, Switzerland
- 3Faculty of Medicine, University of Geneva, Geneva, Switzerland
- 4Service de Pneumologie, Hôpital Pitié-Salpêtrière AP-HP – Sorbonne Université, Paris, France
- 5Pulmonary Department and Respiratory Critical Care Unit, University Hospital Dijon, Dijon, France
Long term noninvasive ventilation (LTNIV) is a recognized treatment for chronic hypercapnic respiratory failure (CHRF). COPD, obesity-hypoventilation syndrome, neuromuscular disorders, various restrictive disorders, and patients with sleep-disordered breathing are the major groups concerned. The purpose of this narrative review is to summarize current knowledge in the field of monitoring during home ventilation. LTNIV improves symptoms related to CHRF, diurnal and nocturnal blood gases, survival, and health-related quality of life. Initially, patients with LTNIV were most often followed through elective short in-hospital stays to ensure patient comfort, correction of daytime blood gases and nocturnal oxygenation, and control of nocturnal respiratory events. Because of the widespread use of LTNIV, elective in-hospital monitoring has become logistically problematic, time consuming, and costly. LTNIV devices presently have a built-in software which records compliance, leaks, tidal volume, minute ventilation, cycles triggered and cycled by the patient and provides detailed pressure and flow curves. Although the engineering behind this information is remarkable, the quality and reliability of certain signals may vary. Interpretation of the curves provided requires a certain level of training. Coupling ventilator software with nocturnal pulse oximetry or transcutaneous capnography performed at the patient's home can however provide important information and allow adjustments of ventilator settings thus potentially avoiding hospital admissions. Strategies have been described to combine different tools for optimal detection of an inefficient ventilation. Recent devices also allow adapting certain parameters at a distance (pressure support, expiratory positive airway pressure, back-up respiratory rate), thus allowing progressive changes in these settings for increased patient comfort and tolerance, and reducing the requirement for in-hospital titration. Because we live in a connected world, analyzing large groups of patients through treatment of “big data” will probably improve our knowledge of clinical pathways of our patients, and factors associated with treatment success or failure, adherence and efficacy. This approach provides a useful add-on to randomized controlled studies and allows generating hypotheses for better management of HMV.
Introduction
Long term noninvasive ventilation (LTNIV) is widely accepted for treatment of chronic hypercapnic respiratory failure (CHRF) related to obstructive or restrictive disorders. Its development was triggered in the early 1980's by the report by Sullivan et al. of the use of CPAP for obstructive sleep apnea syndrome (1). Since then devices have undergone major evolutions, with a progressive shift from volume-cycled to pressure-cycled then multimodal devices, and appearance of auto-titrating modes which can adapt to changes in airway resistance, reactance, or compliance of the respiratory system and aim to ensure a target tidal volume and minute ventilation (2). One of the major contributions of medical engineering for these devices has been the development of built-in software, allowing to monitor in detail items such as compliance, leaks, tidal volume, residual respiratory events, or percentage of cycles triggered/cycled by the device (3–7). Moreover, for some devices, analysis of raw data for flow, pressure and even thoraco-abdominal movements on a breath-by-breath basis is possible. Strategies for monitoring LTNIV have also evolved: historically, elective evaluations of patients on LTNIV were initially most often hospital-based or even ICU-based; at present, they rely more and more on tools such as pulse oximetry, transcutaneous capnography (PtcCO2) and built-in software that can be assessed at home (4). New developments in telemedicine and the growing contribution of health care providers make these data easily available, allowing to remotely assess quality of ventilation.
In this review, we will discuss the benefits, but also the possible pitfalls of monitoring patients under LTNIV, and perspectives as to future options. This review will focus on an adult population under LTNIV.
Why Monitor LTNIV?
The goals of long-term NIV (LTNIV) are to improve symptoms related to chronic hypercapnia and—when present—sleep-related breathing disorders (SRBD), to improve health-related quality of life (HRQL), decrease hospital admissions, correct hypoventilation and optimize SpO2 in order to prevent the development of pulmonary hypertension and cor pulmonale, and improve survival (8). In several indications (chronic obstructive pulmonary diseases (COPD), amyotrophic lateral sclerosis (ALS), restrictive thoracic disorders), improvement of ABG and control of nocturnal hypoventilation are significantly associated with a better prognosis (9–13). In order to achieve these goals, it is mandatory to ensure that: 1/ LTNIV improves daytime and nocturnal arterial blood gases, 2/ compliance to LTNIV is sufficient to have a significant physiological effect, 3/ LTNIV does not generate per se undesired respiratory events or side effects and discomfort, and 4/ HRQL and symptom scores improve over time. Guidelines usually recommend at least one annual elective assessment for patients under LTNIV: the frequency of evaluations depends however on the underlying pathology, its severity and rate of progression, and may be required as often as every 3 months (8, 14, 15).
Monitoring LTNIV: A Step by Step Approach
Daytime and Nocturnal Blood Gases: Capillary, Transcutaneous or Arterial Samples
Normalizing daytime arterial blood gases (ABG) is a major physiological goal for patients under LTNIV (9, 10). Thus, performing daytime measurements of ABG—by puncture of the radial artery—is a standard procedure when monitoring LTNIV. Measurements are performed without NIV, at least 30 min after interrupting NIV (when possible), preferably in a sitting position. Capillary arterialized samples, taken at the earlobe after application of a vasodilating gel, are considered reliable surrogates of arterial ABG for pH and PaCO2 (16–19). Results for PaO2 are less reliable, with a possible underestimation by the capillary technique (20).
In stable chronic respiratory failure, pH is usually within a normal range (2, 21–24). The contribution of bicarbonates to the detection of residual nocturnal hypoventilation is debated because of the impact of a large panel of drugs and comorbidities on HCO3 levels (25, 26). HCO3 levels should not be used as an isolated criterion for detecting nocturnal hypoventilation, but can be indicative of nocturnal hypoventilation and should lead to nocturnal capnography if available.
Daytime ABG measurements under NIV are indicative of correction of PaCO2 by NIV but have been shown to be a poor reflection of correction of nocturnal hypoventilation (27–29). Thus, assessing nocturnal pCO2 is essential for identifying periods of hypoventilation related to leaks, undesired respiratory events including patient-ventilator asynchrony or inappropriate settings.
Nocturnal arterial punctures are a source of discomfort, awaken the patient, and are not recommended. Surrogates of nocturnal ABG assessment are end-tidal CO2 (ETCO2) and transcutaneous capnography (TcPCO2). Reliability of ETCO2 measurements is subject to V/Q mismatch, physiological dead space and ventilatory mode. Importantly, a normal ETCO2 does not exclude hypercapnia, while an elevated ETCO2 is strongly suggestive of hypercapnia but may underestimate actual PaCO2 value (4, 30). Thus, the use of ETCO2 as a surrogate measurement for nocturnal PaCO2 is not recommended in most circumstances (4).
Transcutaneous capnography (TcPCO2) is considered reliable for continuous (nocturnal) monitoring of PaCO2 when patients are hemodynamically stable, and devices are used by an experienced team. A probe temperature of 42°C is tolerated for 8 h without any risk of skin burns in adults (31). Several studies have reviewed the performances of commercialized capnographs and show acceptable biases and limits of agreement (27, 29). A review of the recent literature on transcutaneous capnography suggested that bias values up to ± 1 kPa (7.5 mmHg), and limits of agreement up to ± 1.33 kPa (10 mmHg) were acceptable (27). PtcCO2 however has a few drawbacks: 1/ its lag time which precludes the detection of very short events (32) and 2/ the occurrence of occasional errand values, largely dependent on the expertise of the users.
Consensual—although arbitrary—definitions for hypoventilation have been published by the American Association for Sleep Medicine (33). However, minor changes in definitions of hypoventilation can have a major impact on clinical assessment (34–36).
Pulse oximetry has the advantage of simplicity and low cost: its major drawback is the lack of specificity of desaturations, which do not allow to distinguish hypoventilation from other causes of drops in SpO2.
Contribution of Built-In Monitoring Devices
Compliance
Compliance is directly related to efficacy of NIV (on ABG and symptom control), and prognosis; it may also reflect patient discomfort, which impairs health related quality of life. Analysis of use of LTNIV (compliance) has 3 components: total time spent with the ventilator, pattern of use and evolution of these parameters (Figure 1). Total time spent under NIV has to be long enough for NIV to have a beneficial impact on daytime and nocturnal ABG, and, in some cases, on total energy expenditure (37, 38). It is usually considered that using NIV <3:30 h per 24 h is insufficient and does not allow resetting of respiratory centers and resting of respiratory muscles. Borel et al. described a “U-shaped curve” relationship between compliance and prognosis (39). Too low values provide insufficient benefit, while higher values indicate more severe respiratory impairment (39). In COPD, a meta-analysis by Struik et al. suggests that a daily use of NIV for at least 5 h is required to improve PaCO2 (40). In neuromuscular disorders such as amyotrophic lateral sclerosis or myotonic dystrophy, there is a clear relation between adherence to treatment and survival (41–44). Thus, the best compromise between patient comfort and efficacy must be sought for. Pattern of use (fragmentation vs. continuous use) can be an indicator of discomfort and side-effects (leaks, pain or discomfort related to interface), co-morbidities (nycturia, use of diuretics) or symptomatic patient-ventilator asynchrony (Figure 1) (7, 45, 46). Evolution of total time spent under NIV and changes in pattern of use may be indicative of an exacerbation with a risk of hospital admission (47).
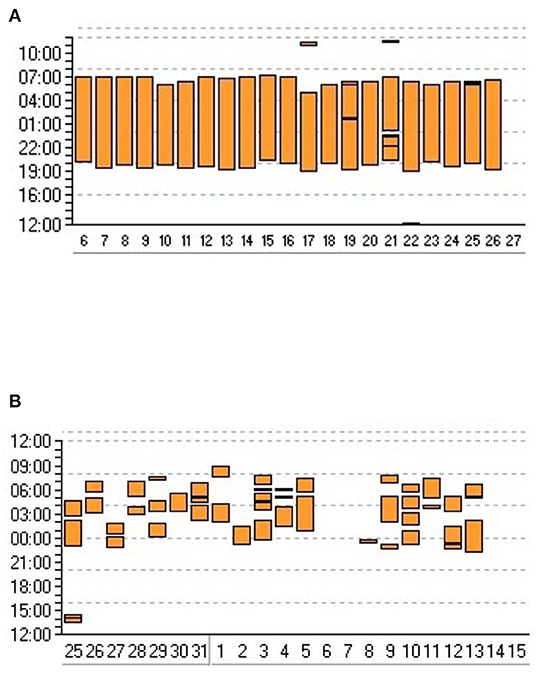
Figure 1. Adherence to NIV. Graphic transcription of ventilator use provided by ventilator software. Y axis: time of the day/night; X axis: days. Each vertical bar represents time spent using the ventilator for a given day. (A) Very regular use suggesting that patient is adherent and comfortable with his/her treatment. (B) Frequent interruptions and irregular use of ventilator suggesting discomfort and/or comorbidity.
Leaks
Leaks in LTNIV are either intentional (i.e., related to exhalation valves to avoid rebreathing) or unintentional (i.e., caused for instance by a poorly adapted interface, too high insufflation pressures, or mouth leaks). Intentional leaks depend on the type of mask and insufflation pressures: interfaces have their predefined leak vs. pressure curves (48). In barometric modes, which are presently by far the most frequently used, ventilators adapt the airflow generated by the turbine to the intentional leak to ensure that the pressure attained in the airways reflects settings determined by the clinicians. Unintentional leaks are by definition unpredictable, most often variable, may differ between inspiratory and expiratory phases, and can be influenced by elements such as facial morphology, type of interface (facial vs. nasal mask), body position, sleep stages or ventilator settings. More importantly, they may interfere with the detection of patient's changes in inspiratory flow (which triggers pressurization or induces cycling). Leaks are thus the most frequent etiology of patient-ventilator asynchrony. They may induce significant and sometimes severe desaturations due to hypoventilation or to a “dilution effect” in patients with supplemental oxygen and can impact on quality of sleep (49). Also, leaks have a detrimental effect on reliability of data provided by ventilator software such as tidal volume, minute ventilation, or percentage of cycles triggered or cycled by the ventilator (3). This may be particularly problematic in automated modes with volume targeting or providing a continuous assessment of airway resistance.
Most built-in monitoring systems coupled to LTNIV ventilators provide data about leaks. However, leaks are estimated and reported differently according to manufacturers, which may misguide the clinician (i.e., average leaks, average leak only during expiratory phase, average leaks with or without intentional leaks) (3, 50, 51). Bench test studies have shown that reliability of reported leaks may vary considerably according to device used and to absolute value of leaks (3, 5, 52). Arbitrary threshold values for acceptable leaks are commonly reported by several manufacturers: the threshold of 24 L/min often referred to was first mentioned in a study by Teschler et al. (53): the relevance of this value is questionable today since the efficacy of ventilator turbines and their capacity to compensate for leaks has improved substantially. A statement by the French GAV-O2 group suggested that leaks are considered troublesome essentially 1/ if they impact on patient comfort and compliance and 2/ if they cause recurrent nocturnal desaturations and episodes of hypercapnia (46).
For the patient, the variability of leaks and peak levels even of short duration may be a more important source of discomfort than the mean or median leak level.
Tidal Volume and Minute Ventilation
Tidal volume (VT) and minute ventilation (MV) are important goals for the clinician when determining ventilator settings: a usual target for VT is 8–10 ml/kg of ideal body weight. The accuracy of VT, and thus MV, depends on the reliability of ventilator software, on pressure values and on leaks (54, 55). In a bench study of 7 home ventilators, bias between measured and reported VT ranged from 66 to 236 ml (3). This may be problematic in automated volume-targeted modes. A high variability of tidal volume may per se be suggestive of leaks.
Cycles Triggered or Cycled by the Device
Quantifying the percentage of cycles triggered by the patient is theoretically an important information. It allows to determine to what extent the patient's respiratory rate (RR) is captured by the ventilator, i.e., to what extent ventilatory support approaches a controlled mode (Figure 2). This may be a goal for clinicians in neuromuscular disorders for instance, to rest inspiratory muscles, or to decrease residual apneas in obesity hypoventilation (56). However, in the presence of leaks, and/or upper airway obstruction, a low percentage of triggered cycles may reflect the fact that the ventilator does not detect the patient's inspiratory efforts. Conversely, a high percentage of cycles triggered by the patient, i.e., a spontaneous mode, may be an indicator of patient-ventilatory synchronization. This parameter must therefore be interpreted with caution, especially in the presence of leaks.
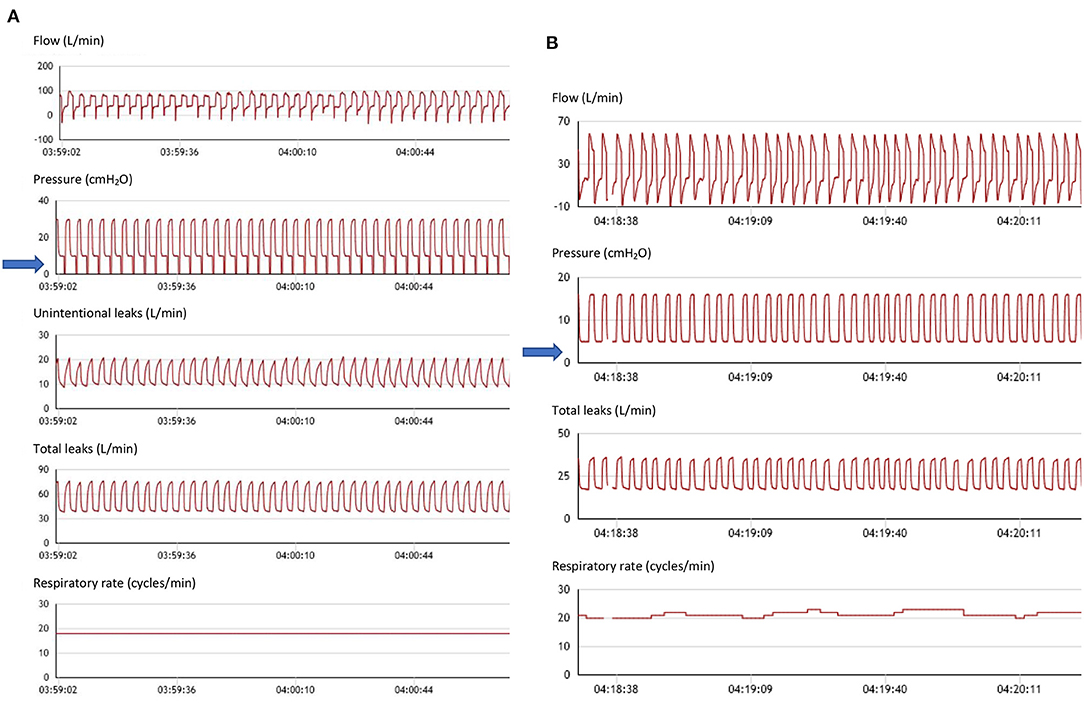
Figure 2. Spontaneous vs. controlled cycles. (A) From top to bottom: flow (including leaks), pressure, unintentional leaks (i.e.: difference between total leak flow and estimated flow through the leak valve (small holes) of the mask), total leaks, and respiratory rate. Seventy six-year-old female subject with kyphoscoliosis, obstructive sleep apnea and obesity; ventilator settings: ST mode (spontaneous/timed); IPAP (Inspiratory positive airway pressure): 30 cmH2O; EPAP: 10 cmH2O (Expiratory positive airway pressure); Back-up respiratory rate (BURR): 18 cycles/min. Nasal mask (facial mask not tolerated). Flow tracing shows intermittent flattening of inspiratory curve suggesting persisting flow limitation (upper airway collapse to be compensated by increasing EPAP). Blue arrow: pressure tracing shows vertical marks associated with each cycle indicating controlled cycle (i.e., delivered by ventilator). On this segment, patient is continuously on back-up respiratory rate. Low level of intentional leaks. (B) From top to bottom: flow (including leaks), pressure, total leaks, and respiratory rate. Fifty four-year-old male subject with restrictive disorder. Ventilator settings: ST mode (spontaneous/timed); IPAP (Inspiratory positive airway pressure): 16 cmH2O; EPAP: 5 cmH2O (Expiratory positive airway pressure); Back-up respiratory rate (BURR): 16 cyc/min; nasal mask. Blue arrow: as opposed to (A), all cycles are triggered by the patient (i.e., spontaneous). Normal aspect of flow and pressure tracings. DirectView software, Philips Respironics.
Ventilators also provide the percentage of respiratory cycles cycled by the patient i.e., for which the predefined percentage of peak inspiratory flow is reached. A high percentage of respiratory cycles cycled by the patient may be considered as evidence of appropriate patient ventilator synchronization. Conversely, a high percentage of cycling by the device may reflect either a “controlled mode” or leaks with or without patient ventilator asynchrony.
Residual Respiratory Events Under LTNIV
The presence of residual respiratory events is frequent and has a documented impact on quality of sleep and sleep structure (23, 57–60) (Figures 3–5). Severe patient-ventilator asynchrony may compromise the efficacy of NIV. Also, events such as recurrent upper airway obstruction may affect survival in ALS (9). It is thus important to evaluate, and correct these events.
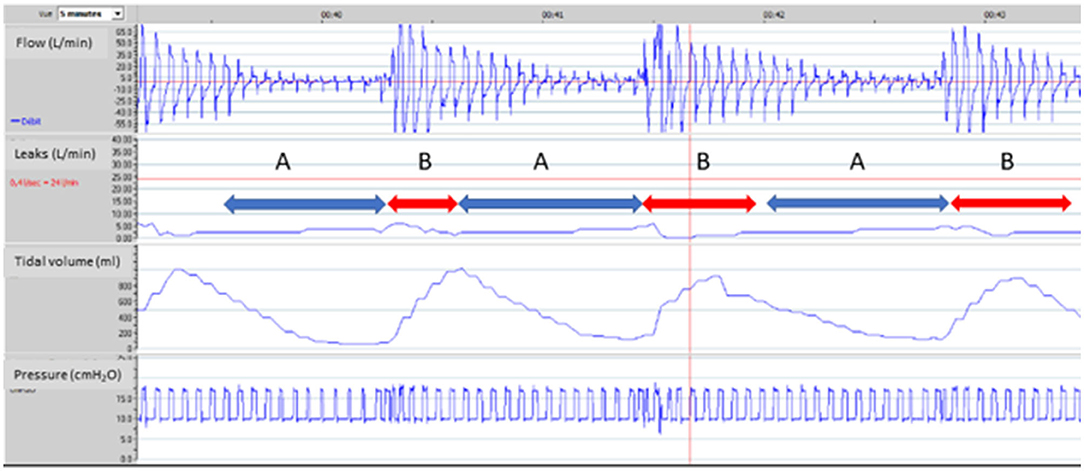
Figure 3. Upper airway obstruction under NIV. 65-year-old male subject, obesity-hypoventilation with obstructive sleep apnea syndrome (OSAS). Bi-level pressure support ventilator, ResScan software, ResMed. Facial mask. Ventilator settings: ST Mode (spontaneous/timed), IPAP (Inspiratory positive airway pressure): 20 cmH2O; EPAP (Expiratory positive airway pressure): 10 cmH2O; BURR (Back-up respiratory rate): 16 cycles/min. From top to bottom: flow, unintentional leaks (i.e.: difference between total leak flow and estimated flow through the leak valve of the mask), tidal volume, pressure. 5 min window. Red line on leaks tracing (24L/min) is a threshold value suggested by manufacturer for upper limit of acceptable leaks (see text for comments). Event A: marked decrease in flow with tracing suggesting increase in upper airway resistance (leading to intermittent complete obstruction); simultaneous decrease in tidal volume without increase in leaks. Event B: sudden transient resumption of flow with a simultaneous increase in tidal volume. Increase in upper airway resistance could be related to an insufficient “pneumatic splint” effect in spite of rather high insufflation pressures, or to glottic closure (further characterization would require respiratory polygraphy). In a patient with a known OSAS, a pragmatic trial of increasing EPAP is an option. Value of IPAP should be increased accordingly to maintain same level of pressure support (if tolerated). Use of a facial mask may also contribute to these events, and may be replaced by nasal mask with chin strap if tolerated.
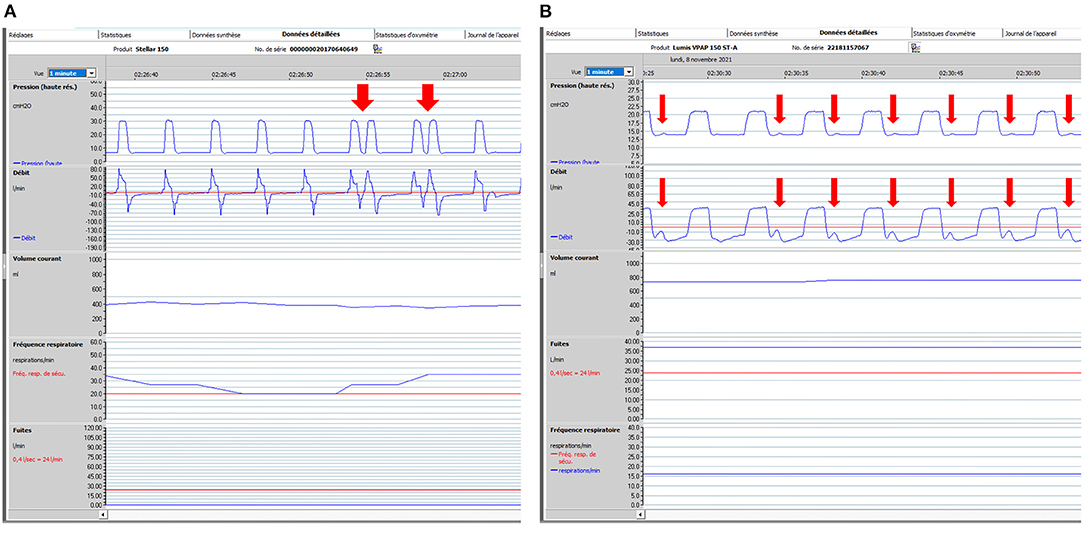
Figure 4. Patient ventilator asynchrony (PVA): illustrative ventilator tracings. One-minute windows. From top to bottom: pressure, flow, tidal volume, respiratory rate and unintentional leaks (i.e., without intentional leak through exhalation valve of mask). Rescan software, ResMed. (A) 74-year-old male subject with severe COPD (FEV1: 20% of predicted). Bi-level pressure support ventilator, Rescan software, Resmed. Ventilator settings: ST (spontaneous/timed) mode; IPAP (Inspiratory positive airway pressure): 30 cmH2O; EPAP (Expiratory positive airway pressure): 7 cmH2O; BURR (back-up respiratory rate) 20 cycles/min. Red arrows show intermittent double-triggering. In this case, leaks are not in cause. Possible causes are dysfunction of ventilator, too high inspiratory trigger sensitivity, too short minimal inspiratory time (TIMIN) with prolonged inspiratory efforts. Adjustments of settings (if required) to be considered are: to increase TIMIN; to adapt cycling sensitivity (at a lower percentage of peak inspiratory flow, which delays cycling); to increase IPAP and reduce rise time; or to decrease sensitivity of inspiratory trigger. (B) 67-year-old male subject with obesity hypoventilation and severe OSAS. Bi-level pressure support ventilator, Rescan software, Resmed. Ventilator settings: ST (spontaneous/timed) mode; IPAP (Inspiratory positive airway pressure): 21 cmH2O; EPAP (Expiratory positive airway pressure): 14 cmH2O; BURR (back-up respiratory rate) 16 cycles/min. Red arrows show low amplitude repeated increases in flow and pressure which represent unrewarded efforts (i.e., inspiratory efforts by the patient which do not trigger the ventilator). Among possible causes are: inappropriate setting of inspiratory trigger sensitivity, increase in upper airway resistance, leaks, intrinsic PEEP (Positive end expiratory pressure), decrease in inspiratory muscle function. For all PVA, control of leaks is mandatory before adjusting other settings.
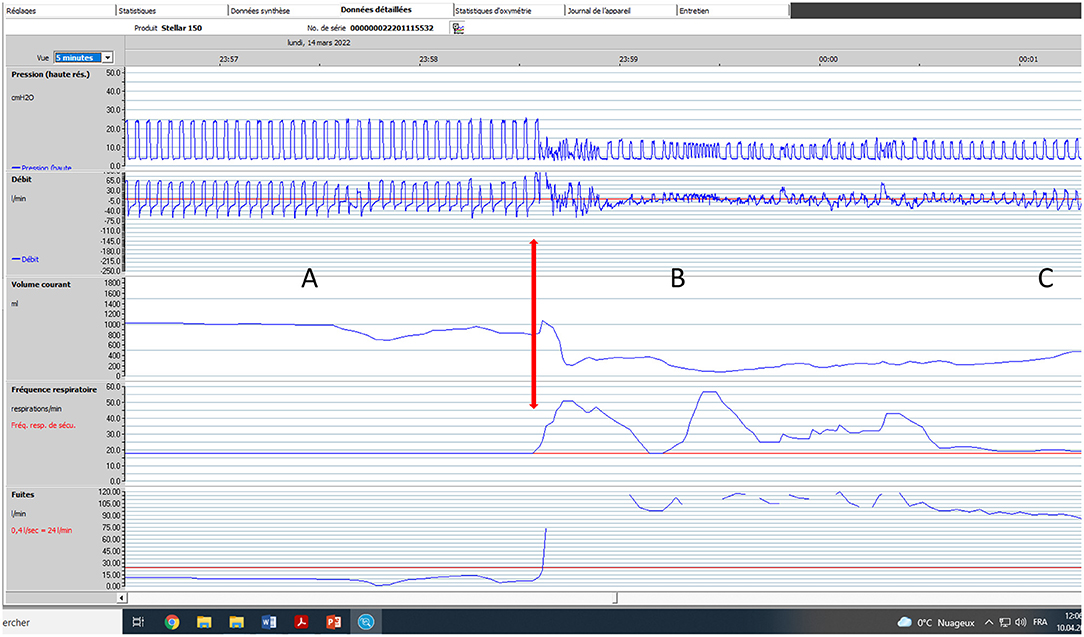
Figure 5. Impact of major leaks. Seventy five-year old male subject with severe COPD (GOLD D; FEV1: 17% of predicted). Bi-level pressure support ventilator, S/T mode (spontaneous/timed); IPAP (Inspiratory positive airway pressure): 24 cmH2O; EPAP (Expiratory positive airway pressure): 4 cmH2O; Back-up respiratory rate (BURR): 18 cycles/min. Facial mask. Five-minute window, Rescan software, ResMed. (A) normal tracing albeit for a few cycles with decrease in flow; (B) Vertical arrow marks appearance of major leaks (could be related to transient displacement of interface). Pressure tracing shows episodes of auto-triggering, and double triggering. Marked drop in pressure, flow and VT (explained by magnitude of leaks). (C) As leaks progressively decrease, breathing pattern becomes more regular; pressure and flow increase progressively.
Most devices report residual respiratory events occurring under NIV: they are labeled as apnea, hypopnea, and sometimes even classified as “central” or “obstructive” according to complex algorithms and estimation of upper airway resistance using the force oscillation technique, the shape of the flow curves or both. The relevance of these parameters in LTNIV is not well-established: these parameters are derived mainly from the use of CPAP in sleep-related disordered breathing (SRDB), and do not take into account the higher level of complexity of undesired residual (or de novo) respiratory events associated with LTNIV. Very few studies have assessed the reliability of these data. Georges et al. showed that the apnea-hypopnea index (AHI) reported by one specific device in patients with obesity-hypoventilation was well-correlated with a manually read polysomnographic assessment, and that a threshold value of 10/hour for AHI allowed to discriminate with a high sensitivity and specificity subjects who were correctly ventilated vs. those for whom adjustments were required (61). In a similar study, Alvarez et al. compared manually scored polygraphy with built-in software data in 26 patients with obesity-hypoventilation: automated analysis of residual events was well-correlated with polygraphy; however, manually scored tracings from ventilator software provided more consistent results than automated software (62). More recently Aarrestad et al. compared manually scored polygraphy and data from ventilator software in 67 subjects on LTNIV for restrictive disorders: a value of AHI above 7.2/hour reported by ventilator software had a sensitivity of 93% (95%CI: 68–100) and a specificity of 92% (95%CI: 81–98) for detecting patients with an AHI > 10/hour scored by polygraphy (34).
Detailed Analysis of Flow and Pressure Curves and Coupling With Pulse Oximetry and Leaks
Ventilator software provides the possibility of a detailed cycle-by-cycle analysis of pressure and flow curves, while simultaneously providing curves of estimated leaks and tidal volume. Oximetry can be added to most devices to complete this analysis. This allows the detection of residual obstructive events with or without persistence of respiratory drive (63), may show periodic breathing, and patient ventilator asynchronies (rate asynchrony such as multiple triggering, auto-triggering, ineffective efforts, or intracycle asynchronies such as underassistance, or overshoot) (49). Coupling the detailed analysis of ventilator curves with oximetry and leaks allows a better assessment of relevance and mechanisms involved, and provides a rationale to adapt ventilator settings. Illustrative cases of contribution of pressure and flow curves are shown in Figures 2–5.
Contribution of Respiratory Polygraphy or Polysomnography
Respiratory polygraphy (PG) or polysomnography (PSG), preferably coupled with TcPCO2, are considered as gold-standard for documenting undesired respiratory events occurring under LTNIV. These events have been reported by several studies (34, 45, 57, 58, 64, 65) and defined in detail by the SomnoNIV group (63). However, PG or PSG under NIV requires expertise. PSG is not easily available in many countries, and thus use of PSG for titrating and/or monitoring LTNIV, although recommended by the AASM (American Association of Sleep Medicine), is not standard practice (66). Some specialized centers use PSG for titration and follow-up (67). Proposed algorithms recommend PSG or PG only after a thorough analysis of symptoms, PtcCO2, ventilator software and correction of leaks (4, 68) (Figure 6).
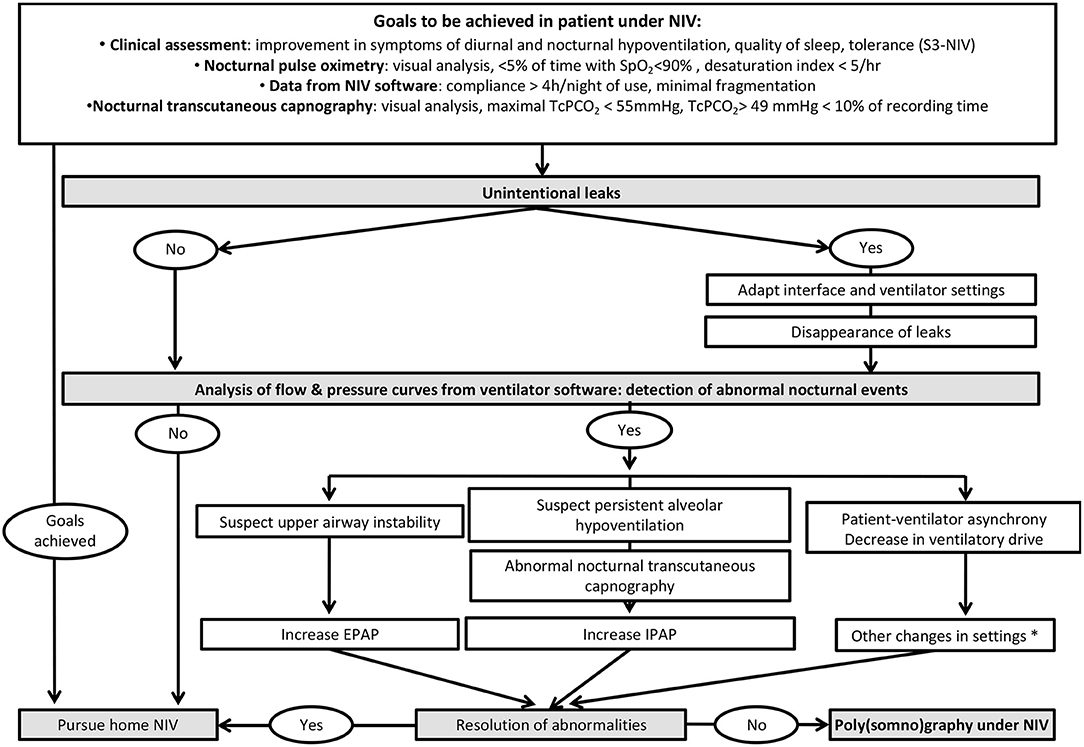
Figure 6. Proposed algorithm for a systematic approach to monitoring of patients under LNIV and analysis of ventilator software. *: the reader is referred to references 4 and 8 for further details.
Symptom Scores and Assessment of Health-Related Quality of Life (HRQL)
Specific HRQL questionnaires have been designed for patients under LTNIV, such as the SRI (Severe Respiratory Insufficiency Questionnaire) which is the most widely used in this setting, with 16 translations and an “App” version available (69, 70). This type of questionnaire is however mostly designed for clinical studies, and not for follow-up of individuals.
A disease-specific symptom score (S3-NIV) has been recently developed (71) and is undergoing several translations: it is an 11-item Lickert scale type questionnaire which can be auto-administered, and provides 2 sub-scores: “respiratory symptoms” and “sleep and NIV-related side effects.” This score is perfectly suitable for clinical practice. It can be coupled with simple tools for assessing mood disturbances such as the Hospital Anxiety and Depression scale (72). These tools do not replace however the necessary face-to-face exchange with the patient and/or his/her caregivers to appreciate the relevance of findings downloaded from ventilator software and mentioned in symptom scores such as the S3-NIV.
Strategies to Detect Ineffective Control of Nocturnal Hypoventilation by NIV
NIV should be systematically monitored (4, 46). As mentioned previously, residual nocturnal hypoventilation, unintentional leaks, patient-ventilator asynchrony or abnormal respiratory events are frequent under NIV and have a negative impact on patient-related outcomes such as symptoms, HRQL and survival. It has been estimated that approximately one third of ventilated patients with normal daytime ABG and nocturnal SpO2 had residual nocturnal hypoventilation under NIV (28, 35, 73). Withholding from performing regular testing of NIV efficacy could therefore be detrimental.
Optimal modalities for monitoring of long-term ventilated patients remain a matter of debate. As previously mentioned, complete polysomnography (PSG) under NIV is performed by some groups, but is not feasible in most centers on a routine basis (74).
Alternative tools (such as oximetry, TcPCO2, or ventilator software) can be used alone or in combination. A step-by-step strategy starting by ABG and nocturnal SpO2 has been proposed by the SomnoNIV group (4) (Figure 6).
Over the past years, the use of TcPCO2 has been simplified (less frequent changes of probes, improved software and estimation of drift). Failure to retrieve data is rare (75) and instrumental drift of TcPCO2 is a minor problem when used by an experienced team (27). Several studies have shown that continuous TcPCO2 recording provides an accurate picture of the overnight time course of PaCO2 in CHRF under NIV. Experts propose different thresholds to define significant nocturnal hypercapnia: maximal TcPCO2 >49 mmHg, TcPCO2 >49 mmHg for >10% of recording time, TcPCO2 >55 mmHg for ≥10 min or an increase in TcPCO2 ≥10 mmHg above awake supine value to a value exceeding 50 mmHg for ≥10 min (33). The choice of a clinically relevant threshold may be influenced by 1/the bias between arterial and transcutaneous PCO2 according to the device used, 2/the etiology of the underlying chronic respiratory failure and 3/PaCO2 levels when NIV is started. Beyond this debate, capnography is currently a well-accepted surrogate procedure for diagnosing nocturnal residual hypoventilation in ventilated patients. Simultaneous recording of SpO2 with the same ear probe improves the clinical contribution of nocturnal transcutaneous capnography. Indeed, sampling rate and averaging of SpO2 and TcPCO2 recordings are different. Therefore, SpO2 traces can detect brief desaturations related to short ventilatory events while TcPCO2 traces allow evaluating overnight trends because of a longer lag time (4).
Significant leaks and/or abnormal residual respiratory events (i.e., flow reduction or patient-ventilator asynchronism) are frequently detected in patients with normal nocturnal TcPCO2 and SpO2 (23, 59, 64).
In a study comparing different tools and their combination, Georges et al. demonstrated that combining the signals provided by TcPCO2 and data from ventilator software provides the best noninvasive assessment of NIV efficacy (73). This approach allows monitoring of LTNIV at the hospital or at home without complex logistics. Interpretation of the results is simple and further analysis of detailed raw data provided by ventilator software can help clarify the underlying mechanism to optimize NIV settings, thus limiting the use of PSG to more complex cases.
A suggested strategy for nocturnal NIV monitoring and parameter optimization is summarized in Figure 6.
Patient-Ventilator Asynchrony and Its' Relevance
Several studies have reported the—sometimes frequent—occurrence of patient ventilator asynchrony (PVA) in patients under LTNIV (23, 57–59, 63, 64, 76) (Figures 4, 5). Their specific semiology and a suggested framework for their analysis has been proposed by the SomnoNIV group (49). Briefly, after correction of leaks and obstructions, which are the major contributors to PVA, a visual analysis of tracings is necessary to detect the presence of rate asynchrony and/or intracycle asynchrony. Rate asynchrony is further subdivided into multiple triggering, uncoupling and ineffective efforts, while intracycle asynchrony can reveal either flow asynchrony (under-assistance) or phase asynchrony (premature or delayed cycling). Clinical relevance of PVA is still a matter of debate, especially if not associated with discomfort and hypoventilation and/or nocturnal desaturation. Scoring of PVA is not yet standardized in the medical literature, and prevalence can vary considerably according to working definitions (23). Seeking for PVA seems however appropriate after correction of leaks in the presence of symptoms and/or unexplained desaturations.
Tele-Monitoring
Although the use of tele-monitoring has become in many countries standard practice for following patients treated by CPAP and Sleep-related breathing disorders (SRBD), and, in some countries, is even required for its reimbursement, this is not the case for LTNIV. Many devices used for LTNIV have the necessary technology built-in, but each manufacturer has its software, and way of reporting certain parameters, representing a challenge for clinicians. Reliability of data remains an issue for some data, as previously discussed (77). In spite of these limitations, home titration and follow-up of LTNIV are emerging strategies. Use of tele-monitoring as an adjunct to home initiation of LTNIV is also an option, and has been shown to be cost-effective in selected indications such as CHRF related to neuromuscular disorders or other restrictive lung diseases (78, 79). Several studies suggest a benefit on healthcare utilization (emergency visits, hospital admissions) and cost-effectiveness of tele-monitoring in ALS (80–82). Preliminary studies have explored the possibility of early detection of exacerbations of COPD under LTNIV (47). For instance, changes in number of hours of NIV use may be a sign of imminent exacerbation, of worsening of underlying disease, or of discomfort, and can be a useful signal for early home intervention (7, 47). On-going trials (reviewed by Borel et al.) (77) will help clarify the modalities of a cost-effective use of tele-monitoring in NIV, and importantly, its benefit (or absence of) on relevant outcomes (such as HRQL, survival, hospital admissions). Points of potential interest for telemonitoring are: follow-up after initiation of NIV following an acute episode of respiratory failure (effective daily use, leaks etc.), progressive titration of NIV after home initiation of LTNIV, and, once specific algorithms will have been validated, early detection of exacerbations or deterioration of disorder causing CRF. The present tools for tele-monitoring already provide a limited “traffic light system” (red, yellow and green icons) usually based on leaks, AHI and compliance. A more elaborate signaling system, using limits for tidal volume, minute ventilation, respiratory rate etc. has yet to be validated in clinical practice, the risk being an unwarranted increase of “red lights.” The possibility of adapting certain parameters at a distance (pressure support, expiratory positive airway pressure, or back-up respiratory rate) allows progressive changes in these settings for increased patient comfort and tolerance, and reduces the requirement for in-hospital titration (83).
The Contribution of “Big Data”
The concept of “Big data” refers to access to a massive quantity of data, its speed of acquisition, and the diversity and heterogeneity of data sources, with potential uncertainties regarding data quality (84). The widespread use of telemonitoring for CPAP has opened the way to a new approach for exploring patient pathways, identifying potentially relevant phenotypes, and elements impacting on adherence and efficacy. For instance, Liu et al. provided very useful data for a better understanding of trajectories of patients with central sleep apnea (emergent, transient, persistent) after initiation of CPAP (85). Technical information such as the impact of changes of interface can be easily assessed through daily longitudinal follow-up data at a large scale. Detailed analysis of day by day use of devices reveals behavioral patterns which improve our understanding of how patients adhere and accept their treatment (86, 87). Recently, it has been possible through telemonitoring to evaluate the impact of the lockdown during the COVID pandemic on adherence to CPAP or NIV (88, 89). Undoubtedly, in a near future, this type of information will become increasingly available and provide a useful adjunct to clinical trials.
Conclusions
The relevance and necessity of a regular monitoring of patients under LTNIV is clearly established, and has an impact on prognosis and HRQL. The progressive shift from hospital-based to home-based assessment over the past years is important for patient comfort and cost-effectiveness of LTNIV. Strategies for monitoring LTNIV include clinical assessment, symptom scores, simple tools such as TcPCO2 or oximetry, ventilator software, and in specific situations, respiratory polygraphy or polysomnography. Although telemonitoring of patients treated by CPAP for SRBD is widely accepted, its use in LTNIV still has to be explored and shown to be cost-effective. Access to big data may provide additional information to better clarify phenotypes of responders and non-responders to LTNIV.
Author Contributions
J-PJ, CC, PP, MG, and CR have contributed to the writing, designing, and revision of the final version of this manuscript and are accountable for the content of this manuscript. All authors contributed to the article and approved the submitted version.
Conflict of Interest
The authors declare that the research was conducted in the absence of any commercial or financial relationships that could be construed as a potential conflict of interest.
Publisher's Note
All claims expressed in this article are solely those of the authors and do not necessarily represent those of their affiliated organizations, or those of the publisher, the editors and the reviewers. Any product that may be evaluated in this article, or claim that may be made by its manufacturer, is not guaranteed or endorsed by the publisher.
References
1. Sullivan CE, Issa FG, Berthon-Jones M, Eves L. Reversal of obstructive sleep apnoea by continuous positive airway pressure applied through the nares. Lancet. (1981) 1:862–5. doi: 10.1016/S0140-6736(81)92140-1
2. Cantero C, Adler D, Pasquina P, Uldry C, Egger B, Prella M, et al. Long term noninvasive ventilation in the Geneva Lake area: indications, prevalence and modalities. Chest. (2020) 158:279–91. doi: 10.1016/j.chest.2020.02.064
3. Contal O, Vignaux L, Combescure C, Pepin JL, Jolliet P, Janssens JP. Monitoring of noninvasive ventilation by built-in software of home bilevel ventilators: a bench study. Chest. (2012) 141:469–76. doi: 10.1378/chest.11-0485
4. Janssens JP, Borel JC, Pepin JL, Somno NIVG. Nocturnal monitoring of home non-invasive ventilation: the contribution of simple tools such as pulse oximetry, capnography, built-in ventilator software and autonomic markers of sleep fragmentation. Thorax. (2011) 66:438–45. doi: 10.1136/thx.2010.139782
5. Rabec C, Georges M, Kabeya NK, Baudouin N, Massin F, Reybet-Degat O, et al. Evaluating noninvasive ventilation using a monitoring system coupled to a ventilator: a bench-to-bedside study. Eur Respir J. (2009) 34:902–13. doi: 10.1183/09031936.00170508
6. Mansell SK, Cutts S, Hackney I, Wood MJ, Hawksworth K, Creer DD, et al. Using domiciliary non-invasive ventilator data downloads to inform clinical decision-making to optimise ventilation delivery and patient compliance. BMJ Open Respir Res. (2018) 5:e000238. doi: 10.1136/bmjresp-2017-000238
7. Pasquina P, Adler D, Farr P, Bourqui P, Bridevaux PO, Janssens JP. What does built-in software of home ventilators tell us? An observational study of 150 patients on home ventilation. Respiration. (2012) 83:293–9. doi: 10.1159/000330598
8. Janssens JP, Michel F, Schwarz EI, Prella M, Bloch K, Adler D, et al. Long-term mechanical ventilation: recommendations of the swiss society of pulmonology. Respiration. (2020) 99:867–902. doi: 10.1159/000510086
9. Georges M, Attali V, Golmard JL, Morelot-Panzini C, Crevier-Buchman L, Collet JM, et al. Reduced survival in patients with ALS with upper airway obstructive events on non-invasive ventilation. J Neurol Neurosurg Psychiatry. (2016) 87:1045–50. doi: 10.1136/jnnp-2015-312606
10. Gonzalez-Bermejo J, Morelot-Panzini C, Arnol N, Meininger V, Kraoua S, Salachas F, et al. Prognostic value of efficiently correcting nocturnal desaturations after one month of non-invasive ventilation in amyotrophic lateral sclerosis: a retrospective monocentre observational cohort study. Amyotroph Lateral Scler Frontotemporal Degener. (2013) 14:373–9. doi: 10.3109/21678421.2013.776086
11. Murphy PB, Rehal S, Arbane G, Bourke S, Calverley PMA, Crook AM, et al. Effect of home noninvasive ventilation with oxygen therapy vs oxygen therapy alone on hospital readmission or death after an acute COPD exacerbation: a randomized clinical trial. JAMA. (2017) 317:2177–86. doi: 10.1001/jama.2017.4451
12. Kohnlein T, Windisch W, Kohler D, Drabik A, Geiseler J, Hartl S, et al. Non-invasive positive pressure ventilation for the treatment of severe stable chronic obstructive pulmonary disease: a prospective, multicentre, randomised, controlled clinical trial. Lancet Respir Med. (2014) 2:698–705. doi: 10.1016/S2213-2600(14)70153-5
13. Tsuboi T, Oga T, Sumi K, Machida K, Ohi M, Chin K. The importance of controlling PaCO(2) throughout long-term noninvasive ventilation. Respir Care. (2014) 59:1671–8. doi: 10.4187/respcare.02829
14. Windisch W, Geiseler J, Simon K, Walterspacher S, Dreher M, on behalf of the Guideline C. German national guideline for treating chronic respiratory failure with invasive and non-invasive ventilation: revised edition 2017 - part 1. Respiration. (2018) 96:66–97. doi: 10.1159/000488001
15. Windisch W, Geiseler J, Simon K, Walterspacher S, Dreher M, on behalf of the Guideline C. German national guideline for treating chronic respiratory failure with invasive and non-invasive ventilation - revised edition 2017: part 2. Respiration. (2018) 96:171–203. doi: 10.1159/000488667
16. Ekkernkamp E, Welte L, Schmoor C, Huttmann SE, Dreher M, Windisch W, et al. Spot check analysis of gas exchange: invasive versus noninvasive methods. Respiration. (2015) 89:294–303. doi: 10.1159/000371769
17. Zavorsky GS, Cao J, Mayo NE, Gabbay R, Murias JM. Arterial versus capillary blood gases: a meta-analysis. Respir Physiol Neurobiol. (2007) 155:268–79. doi: 10.1016/j.resp.2006.07.002
18. Mollard P, Bourdillon N, Letournel M, Herman H, Gibert S, Pichon A, et al. Validity of arterialized earlobe blood gases at rest and exercise in normoxia and hypoxia. Respir Physiol Neurobiol. (2010) 172:179–83. doi: 10.1016/j.resp.2010.05.017
19. Sauty A, Uldry C, Debetaz LF, Leuenberger P, Fitting JW. Differences in PO2 and PCO2 between arterial and arterialized earlobe samples. Eur Respir J. (1996) 9:186–9. doi: 10.1183/09031936.96.09020186
20. Magnet FS, Majorski DS, Callegari J, Schwarz SB, Schmoor C, Windisch W, et al. Capillary PO2 does not adequately reflect arterial PO2 in hypoxemic COPD patients. Int J Chron Obstruct Pulmon Dis. (2017) 12:2647–53. doi: 10.2147/COPD.S140843
21. Masa JF, Mokhlesi B, Benitez I, Gomez de Terreros FJ, Sanchez-Quiroga MA, Romero A, et al. Long-term clinical effectiveness of continuous positive airway pressure therapy versus non-invasive ventilation therapy in patients with obesity hypoventilation syndrome: a multicentre, open-label, randomised controlled trial. Lancet. (2019) 393:1721–32. doi: 10.1016/S0140-6736(18)32978-7
22. Masa JF, Corral J, Caballero C, Barrot E, Teran-Santos J, Alonso-Alvarez ML, et al. Non-invasive ventilation in obesity hypoventilation syndrome without severe obstructive sleep apnoea. Thorax. (2016) 71:899–906. doi: 10.1136/thoraxjnl-2016-208501
23. Aarrestad S, Qvarfort M, Kleiven AL, Tollefsen E, Skjonsberg OH, Janssens JP. Sleep related respiratory events during non-invasive ventilation of patients with chronic hypoventilation. Respir Med. (2017) 132:210–6. doi: 10.1016/j.rmed.2017.10.025
24. Janssens JP, Derivaz S, Breitenstein E, De Muralt B, Fitting JW, Chevrolet JC, et al. Changing patterns in long-term noninvasive ventilation: a 7-year prospective study in the Geneva Lake area. Chest. (2003) 123:67–79. doi: 10.1378/chest.123.1.67
25. Borel JC, Guerber F, Jullian-Desayes I, Joyeux-Faure M, Arnol N, Taleux N, et al. Prevalence of obesity hypoventilation syndrome in ambulatory obese patients attending pathology laboratories. Respirology. (2017) 22:1190–8. doi: 10.1111/resp.13051
26. Jullian-Desayes I, Borel JC, Guerber F, Borel AL, Tamisier R, Levy P, et al. Drugs influencing acid base balance and bicarbonate concentration readings. Expert Rev Endocrinol Metab. (2016) 11:209–16. doi: 10.1586/17446651.2016.1147951
27. Aarrestad S, Tollefsen E, Kleiven AL, Qvarfort M, Janssens JP, Skjonsberg OH. Validity of transcutaneous PCO2 in monitoring chronic hypoventilation treated with non-invasive ventilation. Respir Med. (2016) 112:112–8. doi: 10.1016/j.rmed.2016.01.017
28. Paiva R, Krivec U, Aubertin G, Cohen E, Clement A, Fauroux B. Carbon dioxide monitoring during long-term noninvasive respiratory support in children. Intensive Care Med. (2009) 35:1068–74. doi: 10.1007/s00134-009-1408-5
29. Georges M, Nguyen-Baranoff D, Griffon L, Foignot C, Bonniaud P, Camus P, et al. Usefulness of transcutaneous PCO2 to assess nocturnal hypoventilation in restrictive lung disorders. Respirology. (2016) 21:1300–6. doi: 10.1111/resp.12812
30. Nassar BS, Schmidt GA. Estimating arterial partial pressure of carbon dioxide in ventilated patients: how valid are surrogate measures? Ann Am Thorac Soc. (2017) 14:1005–14. doi: 10.1513/AnnalsATS.201701-034FR
31. Janssens JP, Perrin E, Bennani I, de Muralt B, Titelion V, Picaud C. Is continuous transcutaneous monitoring of PCO2 (TcPCO2) over 8 h reliable in adults? Respir Med. (2001) 95:331–5. doi: 10.1053/rmed.2001.1045
32. Janssens JP, Howarth-Frey C, Chevrolet JC, Abajo B, Rochat T. Transcutaneous PCO2 to monitor noninvasive mechanical ventilation in adults: assessment of a new transcutaneous PCO2 device. Chest. (1998) 113:768–73. doi: 10.1378/chest.113.3.768
33. Berry RB, Budhiraja R, Gottlieb DJ, Gozal D, Iber C, Kapur VK, et al. Rules for scoring respiratory events in sleep: update of the 2007 AASM manual for the scoring of sleep and associated events. Deliberations of the sleep apnea definitions Task Force of the American Academy of Sleep Medicine. J Clin Sleep Med. (2012) 8:597–619. doi: 10.5664/jcsm.2172
34. Aarrestad S, Qvarfort M, Kleiven AL, Tollefsen E, Skjonsberg OH, Janssens JP. Diagnostic accuracy of simple tools in monitoring patients with chronic hypoventilation treated with non-invasive ventilation; a prospective cross-sectional study. Respir Med. (2018) 144:30–5. doi: 10.1016/j.rmed.2018.09.015
35. Ogna A, Quera Salva MA, Prigent H, Mroue G, Vaugier I, Annane D, et al. Nocturnal hypoventilation in neuromuscular disease: prevalence according to different definitions issued from the literature. Sleep Breath. (2016) 20:575–81. doi: 10.1007/s11325-015-1247-2
36. Orlikowski D, Prigent H, Quera Salva MA, Heming N, Chaffaut C, Chevret S, et al. Prognostic value of nocturnal hypoventilation in neuromuscular patients. Neuromusc Disord. (2017) 27:326–30. doi: 10.1016/j.nmd.2016.12.006
37. Gonzalez-Bermejo J, Lofaso F, Falaize L, Lejaille M, Raphael JC, Similowski T, et al. Resting energy expenditure in Duchenne patients using home mechanical ventilation. Eur Respir J. (2005) 25:682–7. doi: 10.1183/09031936.05.00031304
38. Georges M, Morelot-Panzini C, Similowski T, Gonzalez-Bermejo J. Noninvasive ventilation reduces energy expenditure in amyotrophic lateral sclerosis. BMC Pulm Med. (2014) 14:17. doi: 10.1186/1471-2466-14-17
39. Borel JC, Pepin JL, Pison C, Vesin A, Gonzalez-Bermejo J, Court-Fortune I, et al. Long-term adherence with non-invasive ventilation improves prognosis in obese COPD patients. Respirology. (2014) 19:857–65. doi: 10.1111/resp.12327
40. Struik FM, Lacasse Y, Goldstein RS, Kerstjens HA, Wijkstra PJ. Nocturnal noninvasive positive pressure ventilation in stable COPD: a systematic review and individual patient data meta-analysis. Respir Med. (2014) 108:329–37. doi: 10.1016/j.rmed.2013.10.007
41. Pinto A, de Carvalho M, Evangelista T, Lopes A, Sales-Luis L. Nocturnal pulse oximetry: a new approach to establish the appropriate time for non-invasive ventilation in ALS patients. Amyotroph Lateral Scler Other Motor Neuron Disord. (2003) 4:31–5. doi: 10.1080/14660820310006706
42. Seijger C, Raaphorst J, Vonk J, van Engelen B, Heijerman H, Stigter N, et al. New insights in adherence and survival in myotonic dystrophy patients using home mechanical ventilation. Respiration. (2021) 100:154–63. doi: 10.1159/000511962
43. Walsh LJ, Deasy KF, Gomez F, O'Sullivan E, Eustace J, Ryan AM, et al. Use of non-invasive ventilation in motor neuron disease - a retrospective cohort analysis. Chron Respir Dis. (2021) 18:1–9. doi: 10.1177/14799731211063886
44. Aboussouan LS, Khan SU, Meeker DP, Stelmach K, Mitsumoto H. Effect of noninvasive positive-pressure ventilation on survival in amyotrophic lateral sclerosis. Ann Intern Med. (1997) 127:450–3. doi: 10.7326/0003-4819-127-6-199709150-00006
45. Fanfulla F, Delmastro M, Berardinelli A, Lupo ND, Nava S. Effects of different ventilator settings on sleep and inspiratory effort in patients with neuromuscular disease. Am J Respir Crit Care Med. (2005) 172:619–24. doi: 10.1164/rccm.200406-694OC
46. Rabec C, Cuvelier A, Cheval C, Jaffre S, Janssens JP, Mercy M, et al. [Noninvasive ventilation. The 2015 guidelines from the Groupe Assistance Ventilatoire (GAV) of the Societe de Pneumologie de Langue Francaise (SPLF)]. Rev Mal Respir. (2016) 33:905–10. doi: 10.1016/j.rmr.2016.07.003
47. Borel JC, Pelletier J, Taleux N, Briault A, Arnol N, Pison C, et al. Parameters recorded by software of non-invasive ventilators predict COPD exacerbation: a proof-of-concept study. Thorax. (2015) 70:284–5. doi: 10.1136/thoraxjnl-2014-206569
48. Borel JC, Sabil A, Janssens JP, Couteau M, Boulon L, Levy P, et al. Intentional leaks in industrial masks have a significant impact on efficacy of bilevel noninvasive ventilation: a bench test study. Chest. (2009) 135:669–77. doi: 10.1378/chest.08-1340
49. Gonzalez-Bermejo J, Janssens JP, Rabec C, Perrin C, Lofaso F, Langevin B, et al. Framework for patient-ventilator asynchrony during long-term non-invasive ventilation. Thorax. (2019) doi: 10.1136/thoraxjnl-2018-213022
50. Lujan M, Lalmolda C, Ergan B. Basic concepts for tidal volume and leakage estimation in non-invasive ventilation. Turk Thorac J. (2019) 20:140–6. doi: 10.5152/TurkThoracJ.2018.177
51. Lujan M, Sogo A, Grimau C, Pomares X, Blanch L, Monso E. Influence of dynamic leaks in volume-targeted pressure support noninvasive ventilation: a bench study. Respir Care. (2015) 60:191–200. doi: 10.4187/respcare.03413
52. Lujan M, Pomares X. Noninvasive mechanical ventilation. Reflections on home monitoring. Arch Bronconeumol. (2014) 50:85–6. doi: 10.1016/j.arbr.2013.11.003
53. Teschler H, Stampa J, Ragette R, Konietzko N, Berthon-Jones M. Effect of mouth leak on effectiveness of nasal bilevel ventilatory assistance and sleep architecture. Eur Respir J. (1999) 14:1251–7. doi: 10.1183/09031936.99.14612519
54. Lujan M, Sogo A, Pomares X, Monso E, Sales B, Blanch L. Effect of leak and breathing pattern on the accuracy of tidal volume estimation by commercial home ventilators: a bench study. Respir Care. (2013) 58:770–7. doi: 10.4187/respcare.02010
55. Sogo A, Montanya J, Monso E, Blanch L, Pomares X, Lujan M. Effect of dynamic random leaks on the monitoring accuracy of home mechanical ventilators: a bench study. BMC Pulm Med. (2013) 13:75. doi: 10.1186/1471-2466-13-75
56. Contal O, Adler D, Borel JC, Espa F, Perrig S, Rodenstein D, et al. Impact of different backup respiratory rates on the efficacy of noninvasive positive pressure ventilation in obesity hypoventilation syndrome: a randomized trial. Chest. (2013) 143:37–46. doi: 10.1378/chest.11-2848
57. Fanfulla F, Taurino AE, Lupo ND, Trentin R, D'Ambrosio C, Nava S. Effect of sleep on patient/ventilator asynchrony in patients undergoing chronic non-invasive mechanical ventilation. Respir Med. (2007) 101:1702–7. doi: 10.1016/j.rmed.2007.02.026
58. Guo YF, Sforza E, Janssens JP. Respiratory patterns during sleep in obesity-hypoventilation patients treated with nocturnal pressure support: a preliminary report. Chest. (2007) 131:1090–9. doi: 10.1378/chest.06-1705
59. Ramsay M. Patient ventilator asynchrony and sleep disruption during non-invasive ventilation. J Thorac Dis. (2018) 10(Suppl. 1):S80–85. doi: 10.21037/jtd.2017.11.31
60. Vrijsen B, Buyse B, Belge C, Vanpee G, Van Damme P, Testelmans D. Randomized cross-over trial of ventilator modes during non-invasive ventilation titration in amyotrophic lateral sclerosis. Respirology. (2017) 22:1212–8. doi: 10.1111/resp.13046
61. Georges M, Adler D, Contal O, Espa F, Perrig S, Pepin JL, et al. Reliability of apnea-hypopnea index measured by a home bi-level pressure support ventilator versus a polysomnographic assessment. Respir Care. (2015) 60:1051–6. doi: 10.4187/respcare.03633
62. Fernandez Alvarez R, Rabec C, Rubinos Cuadrado G, Cascon Hernandez JA, Rodriguez P, Georges M, et al. Monitoring noninvasive ventilation in patients with obesity hypoventilation syndrome: comparison between ventilator built-in software and respiratory polygraphy. Respiration. (2017) 93:162–9. doi: 10.1159/000454954
63. Gonzalez-Bermejo J, Perrin C, Janssens JP, Pepin JL, Mroue G, Leger P, et al. Proposal for a systematic analysis of polygraphy or polysomnography for identifying and scoring abnormal events occurring during non-invasive ventilation. Thorax. (2012) 67:546–52. doi: 10.1136/thx.2010.142653
64. Ramsay M, Mandal S, Suh ES, Steier J, Douiri A, Murphy PB, et al. Parasternal electromyography to determine the relationship between patient-ventilator asynchrony and nocturnal gas exchange during home mechanical ventilation set-up. Thorax. (2015) 70:946–52. doi: 10.1136/thoraxjnl-2015-206944
65. Crescimanno G, Canino M, Marrone O. Asynchronies and sleep disruption in neuromuscular patients under home noninvasive ventilation. Respir Med. (2012) 106:1478–85. doi: 10.1016/j.rmed.2012.05.013
66. Berry RB, Chediak A, Brown LK, Finder J, Gozal D, Iber C, et al. Best clinical practices for the sleep center adjustment of noninvasive positive pressure ventilation (NPPV) in stable chronic alveolar hypoventilation syndromes. J Clin Sleep Med. (2010) 6:491–509. doi: 10.5664/jcsm.27941
67. Vrijsen B, Chatwin M, Contal O, Derom E, Janssens JP, Kampelmacher MJ, et al. Hot topics in noninvasive ventilation: report of a working group at the international symposium on sleep-disordered breathing in leuven, belgium. Respir Care. (2015) 60:1337–62. doi: 10.4187/respcare.03796
68. Adler D, Perrig S, Takahashi H, Espa F, Rodenstein D, Pepin JL, et al. Polysomnography in stable COPD under non-invasive ventilation to reduce patient-ventilator asynchrony and morning breathlessness. Sleep Breath. (2012) 16:1081–90. doi: 10.1007/s11325-011-0605-y
69. Windisch W, Freidel K, Schucher B, Baumann H, Wiebel M, Matthys H, et al. The Severe Respiratory Insufficiency (SRI) Questionnaire: a specific measure of health-related quality of life in patients receiving home mechanical ventilation. J Clin Epidemiol. (2003) 56:752–9. doi: 10.1016/S0895-4356(03)00088-X
70. Majorski DS, Schwarz SB, Magnet FS, Ahmad R, Mathes T, Windisch W. The Severe Respiratory Insufficiency Application (SRI App): a pilot randomised controlled trial. Thorax. (2021) 76:832–4. doi: 10.1136/thoraxjnl-2020-216319
71. Dupuis-Lozeron E, Gex G, Pasquina P, Bridevaux PO, Borel JC, Soccal PM, et al. Development and validation of a simple tool for the assessment of home noninvasive ventilation: the S(3)-NIV questionnaire. Eur Respir J. (2018) 52:1801182. doi: 10.1183/13993003.011822018
72. Zigmond AS, Snaith RP. The hospital anxiety and depression scale. Acta Psychiatr Scand. (1983) 67:361–70. doi: 10.1111/j.1600-0447.1983.tb09716.x
73. Georges M, Rabec C, Monin E, Aho S, Beltramo G, Janssens JP, et al. Monitoring of noninvasive ventilation: comparative analysis of different strategies. Respir Res. (2020) 21:324. doi: 10.1186/s12931-020-01586-8
74. Borel JC, Gonzalez-Bermejo J. Is it still relevant to consider polysomnography as essential for noninvasive ventilation titration? Eur Respir J. (2019) 53:1900619. doi: 10.1183/13993003.00619-2019
75. Bauman KA, Kurili A, Schmidt SL, Rodriguez GM, Chiodo AE, Sitrin RG. Home-based overnight transcutaneous capnography/pulse oximetry for diagnosing nocturnal hypoventilation associated with neuromuscular disorders. Arch Phys Med Rehabil. (2013) 94:46–52. doi: 10.1016/j.apmr.2012.08.215
76. Duiverman ML, Huberts AS, van Eykern LA, Bladder G, Wijkstra PJ. Respiratory muscle activity and patient-ventilator asynchrony during different settings of noninvasive ventilation in stable hypercapnic COPD: does high inspiratory pressure lead to respiratory muscle unloading? Int J Chron Obstruct Pulmon Dis. (2017) 12:243–57. doi: 10.2147/COPD.S119959
77. Borel JC, Palot A, Patout M. Technological advances in home non-invasive ventilation monitoring: reliability of data and effect on patient outcomes. Respirology. (2019) 24:1143–51. doi: 10.1111/resp.13497
78. van den Biggelaar RJM, Hazenberg A, Cobben NAM, Gaytant MA, Vermeulen KM, Wijkstra PJ. A randomized trial of initiation of chronic noninvasive mechanical ventilation at home vs in-hospital in patients with neuromuscular disease and thoracic cage disorder: the dutch homerun trial. Chest. (2020) 158:2493–501. doi: 10.1016/j.chest.2020.07.007
79. Hazenberg A, Kerstjens HA, Prins SC, Vermeulen KM, Wijkstra PJ. Initiation of home mechanical ventilation at home: a randomised controlled trial of efficacy, feasibility and costs. Respir Med. (2014) 108:1387–95. doi: 10.1016/j.rmed.2014.07.008
80. Ando H, Ashcroft-Kelso H, Halhead R, Chakrabarti B, Young CA, Cousins R, et al. Experience of telehealth in people with motor neurone disease using noninvasive ventilation. Disabil Rehabil Assist Technol. (2021) 16:490–6. doi: 10.1080/17483107.2019.1659864
81. Lopes de Almeida JP, Pinto A, Pinto S, Ohana B, de Carvalho M. Economic cost of home-telemonitoring care for BiPAP-assisted ALS individuals. Amyotroph Lateral Scler. (2012) 13:533–7. doi: 10.3109/17482968.2012.703675
82. Pinto A, Almeida JP, Pinto S, Pereira J, Oliveira AG, de Carvalho M. Home telemonitoring of non-invasive ventilation decreases healthcare utilisation in a prospective controlled trial of patients with amyotrophic lateral sclerosis. J Neurol Neurosurg Psychiatry. (2010) 81:1238–42. doi: 10.1136/jnnp.2010.206680
83. Manis E, Cheng H, Shelgikar AV. Elevated residual apnea-hypopnea index on continuous positive airway pressure download after transition to full-face mask. Ann Am Thorac Soc. (2021) 18:524–6. doi: 10.1513/AnnalsATS.202006-725CC
84. Pepin JL, Bailly S, Tamisier R. Big data in sleep apnoea: opportunities and challenges. Respirology. (2020) 25:486–94. doi: 10.1111/resp.13669
85. Liu D, Armitstead J, Benjafield A, Shao S, Malhotra A, Cistulli PA, et al. Trajectories of emergent central sleep apnea during CPAP therapy. Chest. (2017) 152:751–60. doi: 10.1016/j.chest.2017.06.010
86. Cistulli PA, Armitstead J, Pepin JL, Woehrle H, Nunez CM, Benjafield A, et al. Short-term CPAP adherence in obstructive sleep apnea: a big data analysis using real world data. Sleep Med. (2019) 59:114–6. doi: 10.1016/j.sleep.2019.01.004
87. Patel SR, Bakker JP, Stitt CJ, Aloia MS, Nouraie SM. Age and sex disparities in adherence to CPAP. Chest. (2021) 159:382–9. doi: 10.1016/j.chest.2020.07.017
88. Cantero C, Pasquina P, Dao MD, Cedraschi C, Adler D, Plojoux J, et al. Impact of confinement in patients under long-term noninvasive ventilation during the first wave of the SARS-CoV-2 pandemic: a remarkable resilience. Respiration. (2021) 100:909–17. doi: 10.1159/000516327
Keywords: non-invasive ventilation, chronic hypercapnic respiratory failure, monitoring, home ventilation, long term mechanical ventilation
Citation: Janssens J-P, Cantero C, Pasquina P, Georges M and Rabec C (2022) Monitoring Long Term Noninvasive Ventilation: Benefits, Caveats and Perspectives. Front. Med. 9:874523. doi: 10.3389/fmed.2022.874523
Received: 12 February 2022; Accepted: 26 April 2022;
Published: 19 May 2022.
Edited by:
Laurent Pierre Nicod, University of Lausanne, SwitzerlandReviewed by:
Anita Simonds, Royal Brompton Hospital, United KingdomKonrad E. Bloch, University Hospital Zürich, Switzerland
Copyright © 2022 Janssens, Cantero, Pasquina, Georges and Rabec. This is an open-access article distributed under the terms of the Creative Commons Attribution License (CC BY). The use, distribution or reproduction in other forums is permitted, provided the original author(s) and the copyright owner(s) are credited and that the original publication in this journal is cited, in accordance with accepted academic practice. No use, distribution or reproduction is permitted which does not comply with these terms.
*Correspondence: Jean-Paul Janssens, jp.janssens56@gmail.com