- 1Department of Microbiology, Hospital de la Santa Creu i Sant Pau, Institut d’Investigació Biomèdica Sant Pau (IIB Sant Pau), Barcelona, Spain
- 2Department of Medical Microbiology and Infection Prevention, University Medical Center Groningen, University of Groningen, Groningen, Netherlands
- 3ESCMID Study Group for Genomic and Molecular Diagnostics (ESGMD), Basel, Switzerland
- 4Department of Periodontology and Restorative Dentistry, University of Münster, Münster, Germany
- 5Biodiversity and Epidemiology of Bacterial Pathogens, Institut Pasteur, Paris, France
Whole-genome sequencing (WGS)-based typing methods have emerged as promising and highly discriminative epidemiological tools. In this study, we combined gene-by-gene allele calling and core genome single nucleotide polymorphism (cgSNP) approaches to investigate the genetic relatedness of a well-characterized collection of OXA-48-producing Klebsiella pneumoniae isolates. We included isolates from the predominant sequence type ST405 (n = 31) OXA-48-producing K. pneumoniae clone and isolates from ST101 (n = 3), ST14 (n = 1), ST17 (n = 1), and ST1233 (n = 1), obtained from eight Catalan hospitals. Core-genome multilocus sequence typing (cgMLST) schemes from Institut Pasteur’s BIGSdb-Kp (634 genes) and SeqSphere+ (2,365 genes), and a SeqSphere+ whole-genome MLST (wgMLST) scheme (4,891 genes) were used. Allele differences or allelic mismatches and the genetic distance, as the proportion of allele differences, were used to interpret the results from a gene-by-gene approach, whereas the number of SNPs was used for the cgSNP analysis. We observed between 0–10 and 0–14 allele differences among the predominant ST405 using cgMLST and wgMLST from SeqSphere+, respectively, and <2 allelic mismatches when using Institut Pasteur’s BIGSdb-Kp cgMLST scheme. For ST101, we observed 14 and 54 allele differences when using cgMLST and wgMLST SeqSphere+, respectively, and 2–5 allelic mismatches for BIGSdb-Kp cgMLST. A low genetic distance (<0.0035, a previously established threshold for epidemiological link) was generally in concordance with a low number of allele differences (<8) when using the SeqSphere+ cgMLST scheme. The cgSNP analysis showed 6–29 SNPs in isolates with identical allelic SeqSphere+ cgMLST profiles and 16–61 cgSNPs among ST405 isolates. Furthermore, comparison of WGS-based typing results with previously obtained MLST and pulsed-field gel electrophoresis (PFGE) data showed some differences, demonstrating the different molecular principles underlying these techniques. In conclusion, the use of the different WGS-based typing methods that were used to elucidate the genetic relatedness of clonal OXA-48-producing K. pneumoniae all led to the same conclusions. Furthermore, threshold parameters in WGS-based typing methods should be applied with caution and should be used in combination with clinical epidemiological data and population and species characteristics.
Introduction
The accelerated spread of multidrug-resistant bacterial pathogens poses an important threat to public health (Zhao et al., 2010). One example is the marked increase of carbapenem-resistant Klebsiella pneumoniae producing different carbapenemases such as KPC, VIM, IMP, NDM, and OXA-48 (Oteo et al., 2014). Besides, the propensity of this species to cause outbreaks in healthcare institutions is well known (Snitkin et al., 2012). We need to understand the transmission of resistant pathogens to rapidly and effectively implement control measures during outbreak management (Zhou et al., 2016). Cluster identification and pathogen profiling, including antimicrobial resistance and virulence profile characterization, are crucial for effective infection control (Leopold et al., 2014; Zhou et al., 2015).
Pulsed-field gel electrophoresis (PFGE) and multilocus sequence typing (MLST) have been used as gold standards for many years to confirm suspected outbreaks. However, PFGE results are subjective and difficult to interpret (Leopold et al., 2014), and classical seven-gene MLST is an expensive and time-consuming method (Larsen et al., 2012). In contrast, whole-genome sequencing (WGS) is a technology with a proven high discriminatory power and has become more accessible due to recent technological advances (Larsen et al., 2012; Maiden et al., 2013; Bialek-Davenet et al., 2014; Leopold et al., 2014; Zhou et al., 2015, 2017; Rossen et al., 2018). However, defining criteria to interpret the data obtained by WGS in infection control is difficult and requires a comparison of available methods on well-characterized sets of isolates. Such criteria are advisable so that, following an outbreak, differences among isolates can be interpreted easily and accurately.
The objective of this work was to investigate the molecular epidemiology of a well-characterized collection of OXA-48-producing K. pneumoniae – the dissemination of which occurred in eight hospitals in Catalonia in 2012 (Argente et al., 2019) – using different WGS-based typing methods and to compare them with classical MLST and PFGE. We used the following WGS-based typing methods: (i) core-genome MLST (cgMLST), based on two different schemes, SeqSphere+ and BIGSdb-Kp (Snitkin et al., 2012); (ii) whole-genome MLST (wgMLST), based on the SeqSphere+ scheme (Zhou et al., 2016); and (iii) core-genome single nucleotide polymorphism (cgSNP) analysis. In addition, we investigated and compared phenotypic data and genotypic antimicrobial resistance data obtained using PCR and WGS.
Materials and Methods
Sample Collection
Thirty-seven OXA-48-producing K. pneumoniae isolates were selected from a previous study collection from 2012 and eight Catalan hospitals, including representative isolates of each MLST and PFGE profile, and antimicrobial resistance profile (Argente et al., 2019). These isolates were previously typed by PFGE (Argente et al., 2019) and MLST following the Institut Pasteur seven-gene MLST scheme1 (Diancourt et al., 2005). Information on phenotypic resistance and genotyping results from PCR and Sanger sequencing is summarized in Table 1. The selected isolates were from ST405 (n = 31, corresponding to E1–E18 PFGE patterns), ST101 (n = 3, PFGE patterns A1–A3), ST14 (n = 1, PFGE pattern D), ST17 (n = 1, PFGE pattern B), and ST1233 (n = 1, PFGE pattern C). The studied isolates did not belong to an outbreak, and neither had a known epidemiological link; thus, they were considered sporadic cases.
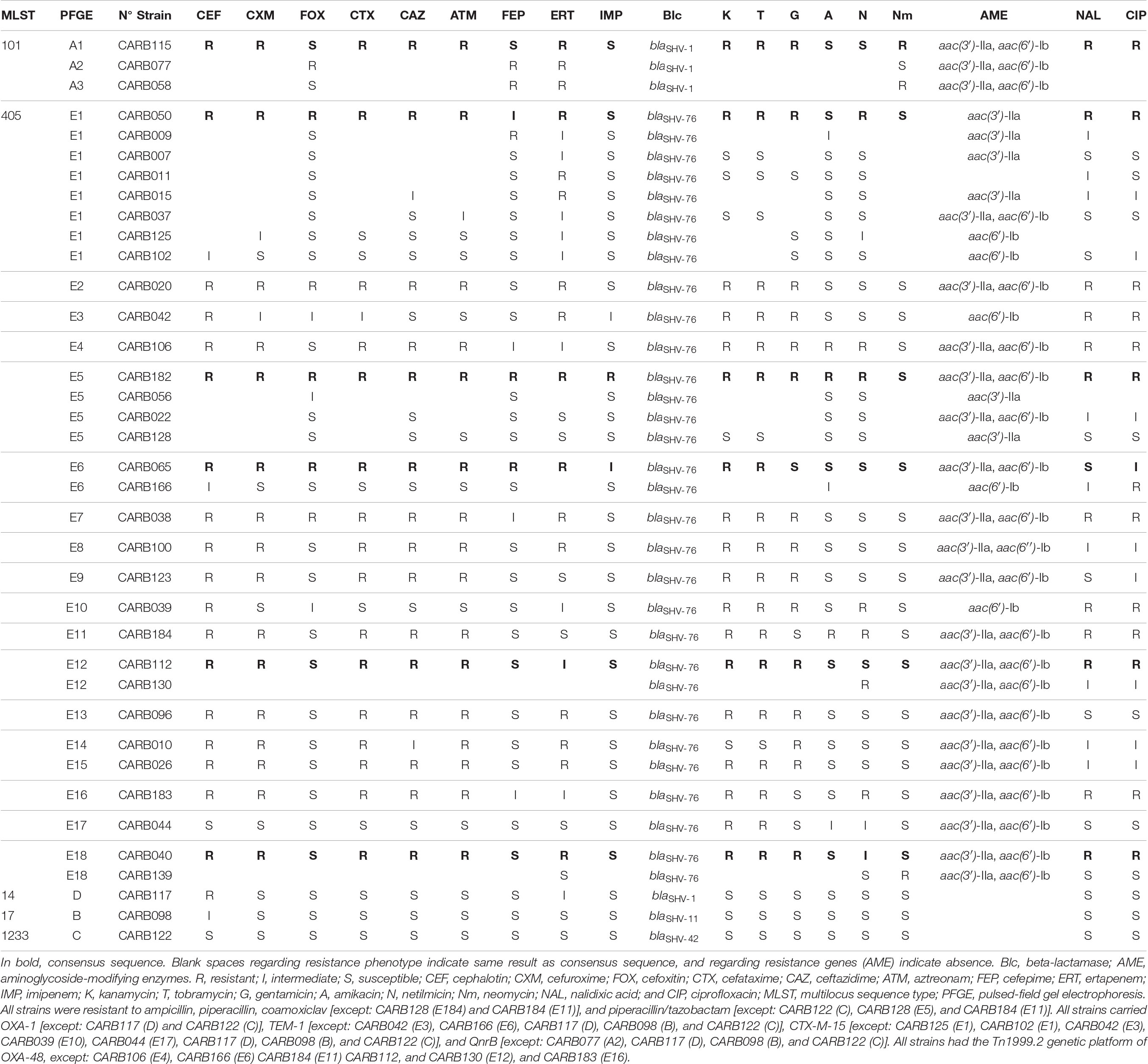
Table 1. Phenotypic and genotypic characters of the 37 OXA-48-producing K. pneumoniae strains obtained by conventional methods, PCR, and Sanger sequencing.
WGS
After DNA extraction using the Ultraclean Microbial DNA Isolation Kit (MO BIO Laboratories, Carlsbad, CA, United States), WGS was applied to the 37 OXA-48-producing K. pneumoniae isolates. The libraries were prepared using a Nextera XT v.01 kit (Illumina Inc., San Diego, CA, United States) and sequenced on an Illumina MiSeq sequencer (Illumina Inc., San Diego, CA, United States) to generate 2 × 250 bp paired-end reads. After sequencing, the reads were quality trimmed and de novo assembled using the CLC Genomics Workbench software v7.0.4 (CLC Bio, Aarhus, Denmark), with the default parameters, except for “removal of low quality sequence limit,” which was set to 0.01 to increase the quality of the called bases.
Assembly quality was assessed using QUAST v4.5 (Gurevich et al., 2013), and a report including assembly metrics was generated (Supplementary Table S1).
Core-Genome and Whole-Genome MLST (cgMLST, wgMLST), Ridom SeqSphere+ Schemes
The assembled genomes were imported into SeqSphere+ software v5.1.0 (Ridom GmbH, Münster, Germany) for a gene-by-gene allele calling comparison using a higher number of loci than the already published Institut Pasteur’s BIGSdb-Kp cgMLST scheme (Bialek-Davenet et al., 2014). A hard defined and stable scheme comprising 2,365 targets or genes of the K. pneumoniae core genome (cgMLST) and 2,526 genes of the K. pneumoniae accessory genome (wgMLST; total of 4,891 targets), available in SeqSphere+, was used for the analysis (targets included in the schemes can be found at cgMLST.org). This scheme was developed using the seed genome K. pneumoniae subsp. pneumoniae NTUH-K2044 (GenBank accession no. NC_012731.1; 15-JUN-2016) and comparing it with 30 query genome sequences that cover the genetic variability of the whole species complex, i.e., K. pneumoniae, K. variicola, and K. quasipneumoniae, as previously defined (Bialek-Davenet et al., 2014). In addition, 61 plasmid sequences were used to exclude chromosomal homologs of plasmid genes. A detailed description of the scheme development is available in the software. In addition, a cgMLST complex type (CT) function was used to identify isolates with very similar cgMLST profiles2. A cluster alert distance of 15 cgMLST allele differences and a cluster alert quality threshold of at least 90% good cgMLST targets were used to detect closely related isolates. These thresholds were based on retrospective analysis of well-defined outbreaks and out-group isolates with the same MLST/MLVA/PFGE profiles. Distance matrices describing pairwise allele differences (ignoring missing values) are included in Supplementary Data Sheet S1 (cgMLST and wgMLST, SeqSphere+).
Furthermore, another parameter named the genetic distance was calculated for paired isolates as the proportion of allele differences, obtained by dividing the number of allele differences between the two genomes by the total number of called genes shared by those two genomes. We considered previously described genetic distance thresholds, 0.0035 for cgMLST and 0.0045 for wgMLST, to discriminate between epidemiologically related and unrelated K. pneumoniae isolates (Kluytmans-van den Bergh et al., 2016).
cgMLST, BIGSdb-Kp Scheme
Assembled genomes were analyzed using the Institut Pasteur BIGSdb-Kp3 634-gene strict core-genome MLST (scgMLST) scheme (Bialek-Davenet et al., 2014). A spanning tree (BioNumerics v6.7.3, Applied-Maths, Belgium) was constructed from the allelic profiles of this cgMLST scheme to visualize the corresponding diversity of genotypes (figure in Supplementary Figure S1).
Core-Genome SNP Analysis
The number of single nucleotide polymorphisms (SNPs) in the core genome (defined as orthologous sequences conserve in all aligned genomes) was calculated from the de novo assembled genomes for isolates with the same ST (ST405 and ST101) using the Harvest software suite (parsnp), including recombination filtration (Treangen et al., 2014). A cgSNP-based tree was built using the Neighbor Joining method.
Antimicrobial Resistance Genes and Plasmid Analysis
In addition to WGS typing, resistance genotypes and plasmid families were investigated in the collection of OXA-48-producing K. pneumoniae and compared with those obtained previously (Argente et al., 2019). Genome assemblies were uploaded to the Center for Genomic Epidemiology to extract information on antimicrobial resistance genes (ARGs) and plasmid replicons for K. pneumoniae using ResFinder 2.1 and PlasmidFinder 1.3, respectively (Zankari et al., 2012; Carattoli et al., 2014).
The de novo assembled genomes were annotated using Prokka 1.12 (Seemann, 2014) and compared with reference K. pneumoniae plasmid pOXA-48 NC_019154.1 using BLASTn analysis. Circular representation of compared genomes was performed with the Circular Genome Viewer (CGView) Comparison Tool program (Grant et al., 2012), in which the coding sequences (CDS) in reference and query sequences were used. In addition, generated blastn output files were further used for visualization using DNAplotter (Carver et al., 2009) and for further analysis using the Artemis Comparison Tool (Carver et al., 2005). Similarity matches were filtered based on their lengths (100 kb segments) and percentage similarity scores, and only the filtered hits with at least 80% sequence similarity were subsequently displayed using the Artemis Comparison Tool.
Results
cgMLST and wgMLST Schemes From Ridom SeqSphere+
The cgMLST analysis of the 37 isolates resulted in nine CTs: 185, 952, and 1149 to 1155 (Figure 1A). Among isolates of MLST type ST405 (n = 31), three different CTs were assigned: CT185 (PFGE subtypes E1, E4, E5, E7, E14, E15, E17, and E18), CT1150 (PFGE subtypes E1, E2, E3, E5, E6, E8, E10–E13, and E16), and CT1153 (PFGE subtype E9). The three ST101 isolates (PFGE subtypes A1–A3) had different CTs (CTs 952, 1152, and 1154) (Figure 1A). ST14, ST17, and ST1233 isolates (n = 1, each ST) (PFGE profiles D, B, and C, respectively) were assigned to CTs 1151, 1155, and 1149, respectively. These minority STs (ST14, ST17, and ST1233) were clearly separated from the ST405 and ST101 isolates, with an allele distance from ST405 isolates of more than 1,875 allele differences when using cgMLST (Figure 1A), and more than 3,618 alleles when using wgMLST (Figure 1B).
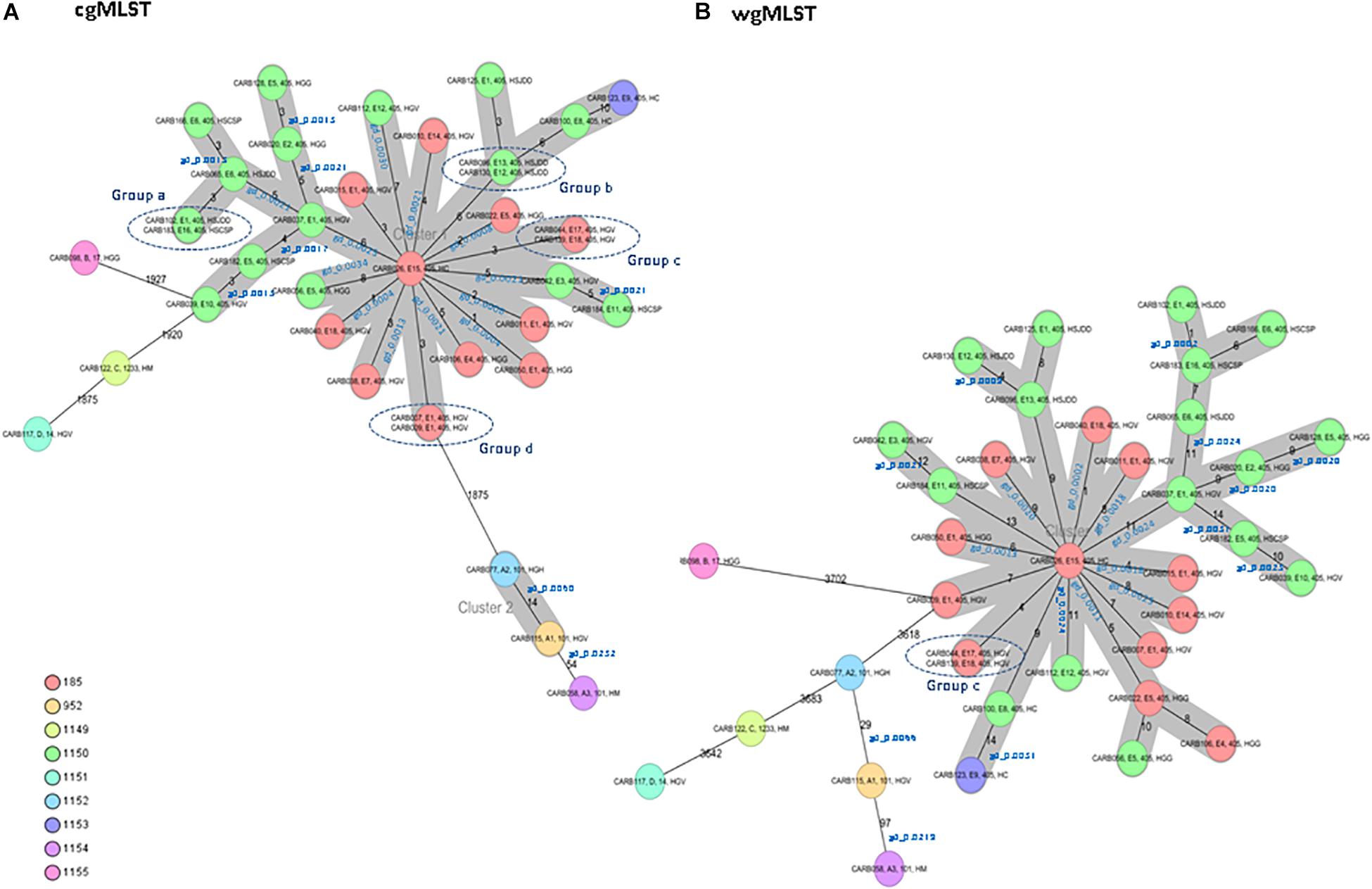
Figure 1. Minimum spanning tree of 37 OXA-48-producing K. pneumoniae isolates obtained from a previous study (Argente et al., 2019). Each circle represents a genotype, and the number of different alleles is indicated on the edges between connected isolates (nodes). In addition, the genetic distance (gd) for specific pairwise comparisons is indicated in blue (see also Table 2). Isolates are presented by their ID, pulsed-field gel electrophoresis (PFGE) profile, sequence type (ST), and hospital origin. Colors indicate the different complex types (CTs). The cluster distance threshold is 15. (A) Distance based on whole-genome multilocus sequence typing (cgMLST) of 2,365 genes using the parameters “pairwise ignoring missing values” during calculation. Groups of isolates (groups a, b, c, and d) with 0 allele differences obtained by cgMLST analysis are highlighted. (B) Distance based on whole-genome multilocus sequence typing (wgMLST) of 4,891 genes (cgMLST of 2,365 core genes and 2,526 accessory genes) using the parameters “pairwise ignoring missing values” during calculation. Isolates of group c that were previously investigated by cgMLST analysis are highlighted.
Isolates within ST101, CT952 (PFGE-A1), and CT1152 (PFGE-A2) showed 14 different alleles by cgMLST (Figure 1A and Table 2) and differed in two bands by PFGE (97% similarity; data not shown). However, these isolates had a genetic distance of 0.0060 indicating no genetic relatedness. Clinical epidemiological data of isolates clustering together by cgMLST (<15 allele differences) are described in Table 3.
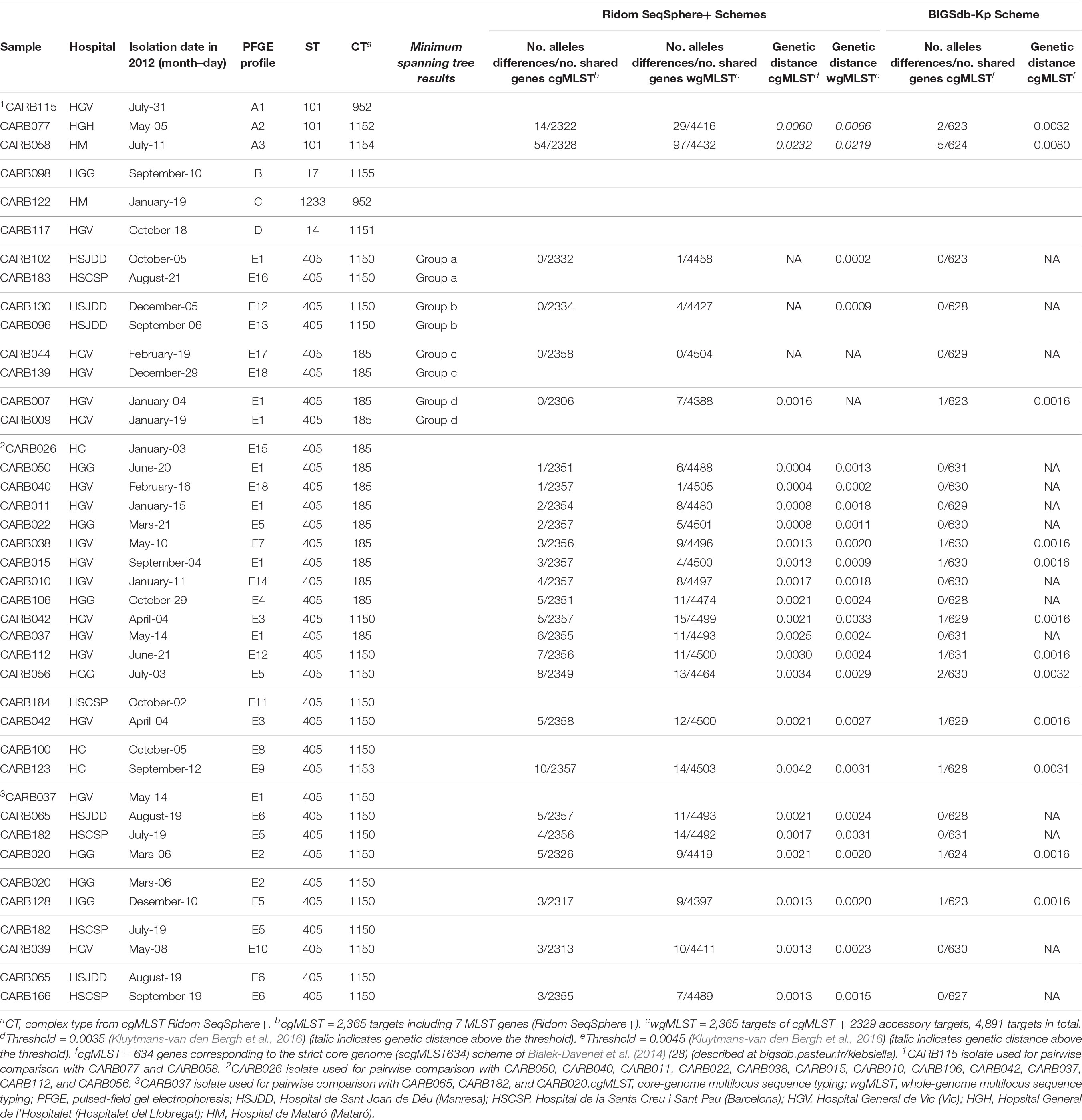
Table 2. Genetic distance for pairwise comparisons of OXA-48- K. pneumoniae isolates using cgMLST/wgMLST schemes from Ridom SeqSphere+ and cgMLST from BIGSdb-Kp (Institut Pasteur).
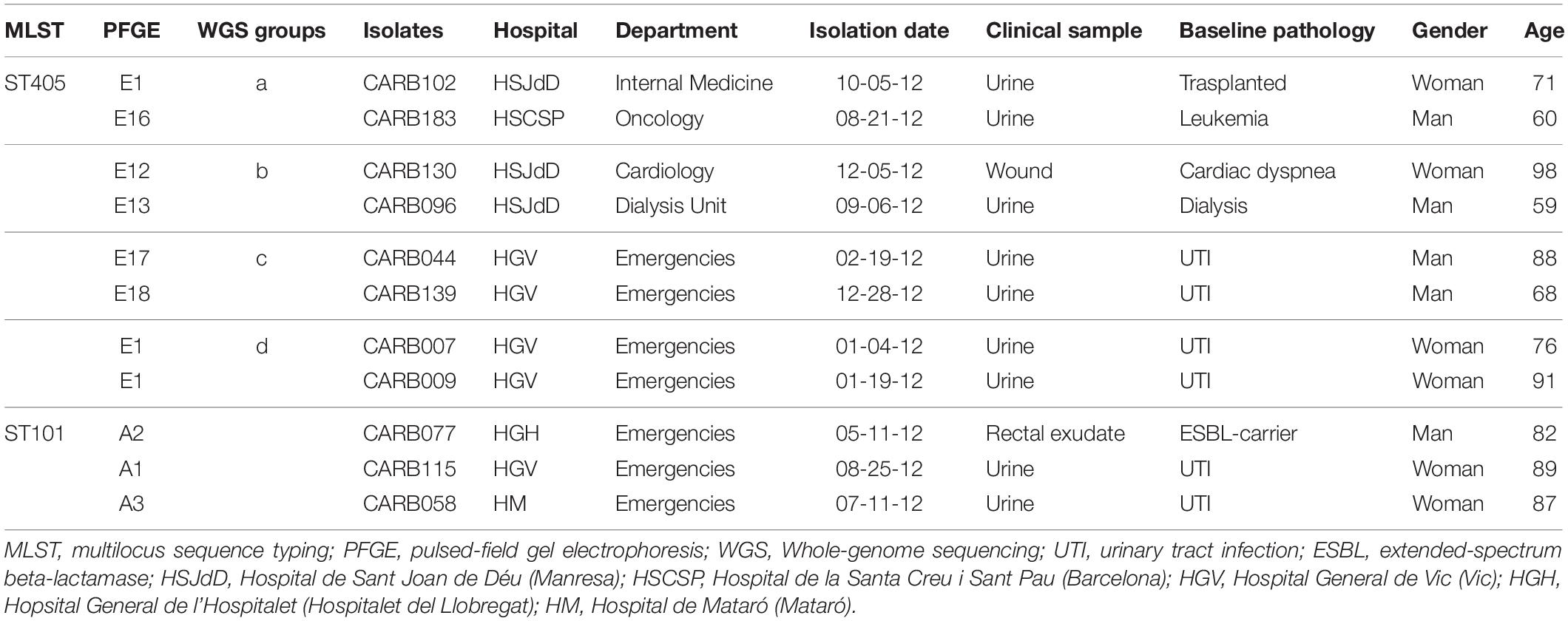
Table 3. Epidemiological and clinical context of OXA-48-producing K. pneumoniae isolates that clustered together using cgMLST.
Isolates within ST405 (PFGE subtypes E1–E18, which differed in less than three bands between them) differed by a maximum of 10 alleles by cgMLST and 14 alleles by wgMLST (Figures 1A,B). All of them had a genetic distance below the threshold (0.0035) and belonged to CT185 or CT1150, except isolates CARB100 (CT1150) and CARB123 (CT1153), which showed a genetic distance of 0.0042 (10 allele differences) by cgMLST (Table 2). In contrast, when considering the wgMLST analysis, CARB100 and CARB123 had a genetic distance of 0.0031 (14 allele differences) indicating genetic relatedness (Table 2). By PFGE analysis, these two isolates showed 95% similarity (two different bands).
Four pairs of isolates belonging to PFGE-E subtypes, CARB102-E1/CARB183-E16 (group a), CARB130-E12/CARB096-E13 (group b), CARB044-E17/CARB139-E18 (group c), and CARB007-E1/CARB009-E1 (group d), showed no allele differences when analyzed by cgMLST (Figure 1A). When using wgMLST only, isolates from group c had zero allele differences (Figure 1B), whereas isolates from group a showed one allele difference, isolates from group b showed four allele differences, and isolates from group d were genetically unrelated (Figure 1B).
Analysis of Isolates Using the BIGSdb-Kp cgMLST Scheme
We obtained identical seven-gene MLST STs for all isolates when using the BIGSdb-Kp database to those previously determined using other WGS analysis tools, confirming the high reproducibility of the seven-gene MLST scheme from genomic assemblies. All ST405 isolates had ≤2 allelic mismatches (mostly 0 allelic mismatches) (Table 2; figure in Supplementary Figure S1). The three ST101 isolates presented between 2 and 5 allelic mismatches, whereas ST14, ST17, and ST1233 isolates were genetically unrelated with more than 480 allelic mismatches (figure in Supplementary Figure S1).
We also calculated the genetic distance for this scheme; all paired isolates had a genetic distance below 0.0032, except for CARB115-A1 and CARB058-A3, which had a genetic distance of 0.0080 (five allelic mismatches) (Table 2).
Core-Genome SNP Analysis
Isolate CARB007-E1 – the oldest isolate – was used as a reference genome for the cgSNP analysis of ST405 isolates (n = 31). The average of core genome alignment was 88.7%. Among ST405 isolates, cgSNP distance ranged between 16 and 61 core SNPs (Supplementary Figure S2 and Supplementary Data Sheet S2). The four groups of two isolates that had identical allelic profiles, as analyzed by cgMLST (Figure 1A), showed 6, 29, 6, and 17 SNPs, respectively, for groups a, b, c, and d. The average of core genome alignment for ST101 isolates was 85.4%. ST101 isolates CARB115-A1 and CARB077-A2 showed 2,427 and 2,673 core SNPs, respectively, compared with isolate CARB058-A3, which was used as reference (Supplementary Figure S2 and Supplementary Data Sheet S2), whereas the number of core SNPs between CARB115-A1 and CARB77-A2 was 54 (Supplementary Figure S2 and Supplementary Data Sheet S2).
Antimicrobial Resistance Genes From WGS Data Compared to PCR and Phenotypic Results
The ResFinder tool from the Center for Genomic Epidemiology detected the presence of multiple resistance genes implicated in β-lactams, aminoglycosides, and quinolone resistance as follows. All isolates were positive for blaOXA–48, except CARB077, and for one of the following blaSHV genes: blaSHV–1 (n = 4), blaSHV–11 (n = 4), blaSHV–42 (n = 1), and blaSHV–76 (n = 31) (Table 1). In addition, 78.4% (29/37) of the isolates contained blaCTX–M–15; 56.4% (22/37), blaOXA–1; and/or 81% (30/37), blaTEM–1 genes (Table 4). We observed some discrepancies with results previously obtained by PCR and Sanger sequencing (Argente et al., 2019). In three isolates (CARB009, CARB050, and CARB056), the blaOXA–1 gene was detected by PCR but not by WGS, whereas in two isolates (CARB039 and CARB044), the blaCTX–M–15 gene was not detected by PCR but detected by WGS. In the latter case, the phenotypes were in concordance with the PCR results; the isolates were susceptible to third-generation cephalosporins. Finally, CARB184 isolate was positive for the blaCTX–M–15 gene by PCR (in concordance with its antimicrobial susceptibility testing results), but this gene was not detected by WGS.
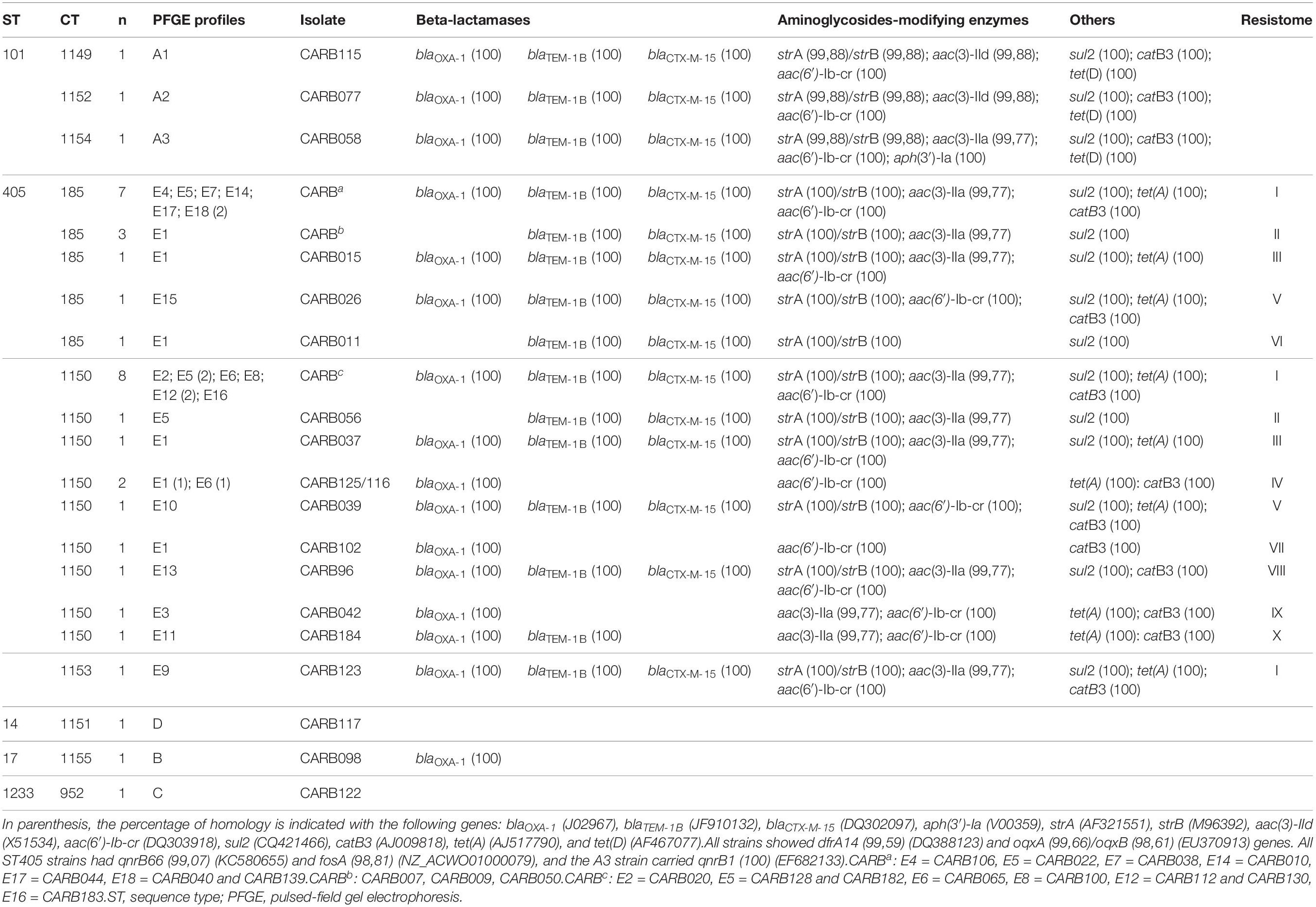
Table 4. Cluster types (CTs) and resistance genes found in the 37 carbapenemase-producing K. pneumoniae (except one isolate, by ResFinder, all were positive for blaOXA–48, and for one of the following genes: blaSHV–1, blaSHV–11, blaSHV–42, and blaSHV–76).
We observed five acquired genes related to aminoglycoside resistance: strA/strB (78.4%; 29/37) and aph(3′)-Ia (2.7%; 1/37) (streptomycin resistance), aac(6′)-Ib-cr (78.4%; 29/37) (kanamycin, tobramycin, amikacin, netilmicin, and fluoroquinolone resistance), aac(3)-IIa (70.3%; 26/37), and aac(3)-IId (5.4%; 2/37) (kanamycin, gentamycin, tobramycin, and netilmicin resistance).
We compared the nucleotide sequences of the gyrA, gyrB, parC, and parE genes and their amino acid sequences, of the entire isolate collection with those of the ATCC reference strain 13883, a quinolone-susceptible strain (Brisse et al., 1999). Of the 37 isolates, 29 had a ciprofloxacin minimal inhibitory concentration ≥2 mg/L, but mutations in gyrA and parC were only found in 5 isolates. The ST101 isolates CARB058, CARB077, and CARB115 had non-silent mutations in the gyrA gene (Ser83Tyr, Asp87Gly, or Asp87Ala) and the parC gene (Ser80Ile, Asn304Ser). The ST405 isolate CARB039 had a non-silent mutation in the gyrA gene (Ser83Ala). In addition, one ST17 isolate (CARB098) showed two new mutations in the gyrA gene (Ala863Val, Thr868Ile); however, the ciprofloxacin minimal inhibitory concentration for this isolate was 1 mg/L. In addition, qnrB66 (81.6%), qnrB1 (27%), and aac(6′)-Ib-cr genes (78.4%) were present, which could explain a certain level of quinolone resistance (Table 4). However, seven isolates carrying the qnrB gene (CARB007, CARB011, CARB037, CARB044, CARB096, CARB128, CARB139) and five isolates carrying the aac(6′)-Ib-cr gene (CARB037, CARB044, CARB096, CARB128, CARB139) were susceptible to ciprofloxacin and nalidixic acid. OqxA/B genes, encoding an efflux pump, were present in all isolates. Nevertheless, this mechanism is associated with a low level of resistance to several antimicrobials, such as conferring low to intermediated resistance to quinoxalines, quinolones, tigecycline, nitrofurantoin, several detergents, and disinfectants (benzalkonium chloride, triclosan, and sodium dodecyl sulfate).
Other resistance genes identified by WGS were sul2 (78.4%) and dfrA14 (91.9%) genes (co-trimoxazole resistance), tet(A) (64.8%) and tet(D) (8.1%) genes (tetracycline resistance), and catB3 (72.9%) (chloramphenicol resistance) and fosA (83.8%) (fosfomycin resistance).
We defined different resistance profiles (resistomes) based on the combination of acquired ARGs (Table 4). Resistome I included 14 ARGs, whereas resistomes XVI to XVIII only included 1 ARG each. We observed three different resistomes (XIII to XV) in ST101 isolates, in concordance with CTs (cgMLST) and PFGE profiles; whereas in ST405 isolates, we observed 12 resistomes (I–XII); the two most predominant ones (I and II) were shared between CT185, CT1150, and CT1153.
ST405 isolates of two of the three groups that showed no allele differences by cgMLST analysis and that had different PFGE profiles displayed a different resistance pattern. Isolates from group a had resistance patterns I/VII, and isolates from group b had resistance patterns I/III, with the difference being the presence of tetA gene (Table 4). In contrast, isolates from group c that had the same resistome (I/I) and the same CT were identified by wgMLST as having identical allelic profiles.
Plasmid Analysis
Plasmid analysis revealed that all but two isolates (CARB039-E10 and CARB077-A2) were positive for a plasmid of the incompatibility group (Inc) L. All isolates also carried plasmids of Inc FIB, and all isolates except those of PFGE profiles B (ST17), C (ST1233), and D (ST14) also carried a plasmid of the Inc FIIk (Supplementary Table S2). In addition, two isolates (ST101; A1 and A2) were positive for an Inc R plasmid, and two (ST405; E4 and E5) had an Inc HI1B plasmid. Inc FIB, FII, and HI1B plasmids were not previously amplified by PCR-based replicon typing (PBRT) (Argente et al., 2019). However, Inc ColE and FIA plasmids, previously detected by PBRT, were not detected by WGS analysis since they are not included in the PlasmidFinder 1.3 tool (Supplementary Table S2). In addition, we found plasmid FIB homology with three previously described plasmids of the same incompatibility group [FIB (PKPHS1) (95.54%), FIB (Mar) (99.77%), FIB (PKPHS1) (96.01%)].
Figure 2 shows a similarity of 84–100% between annotated coding sequences of the plasmid pOXA-48 reference genome and genome assemblies of OXA-48-producing K. pneumoniae isolates from this study. Plasmid L genetic rearrangements, such as deletions, are shown in Supplementary Figure S3 and did not show correlation with the cgMLST tree.
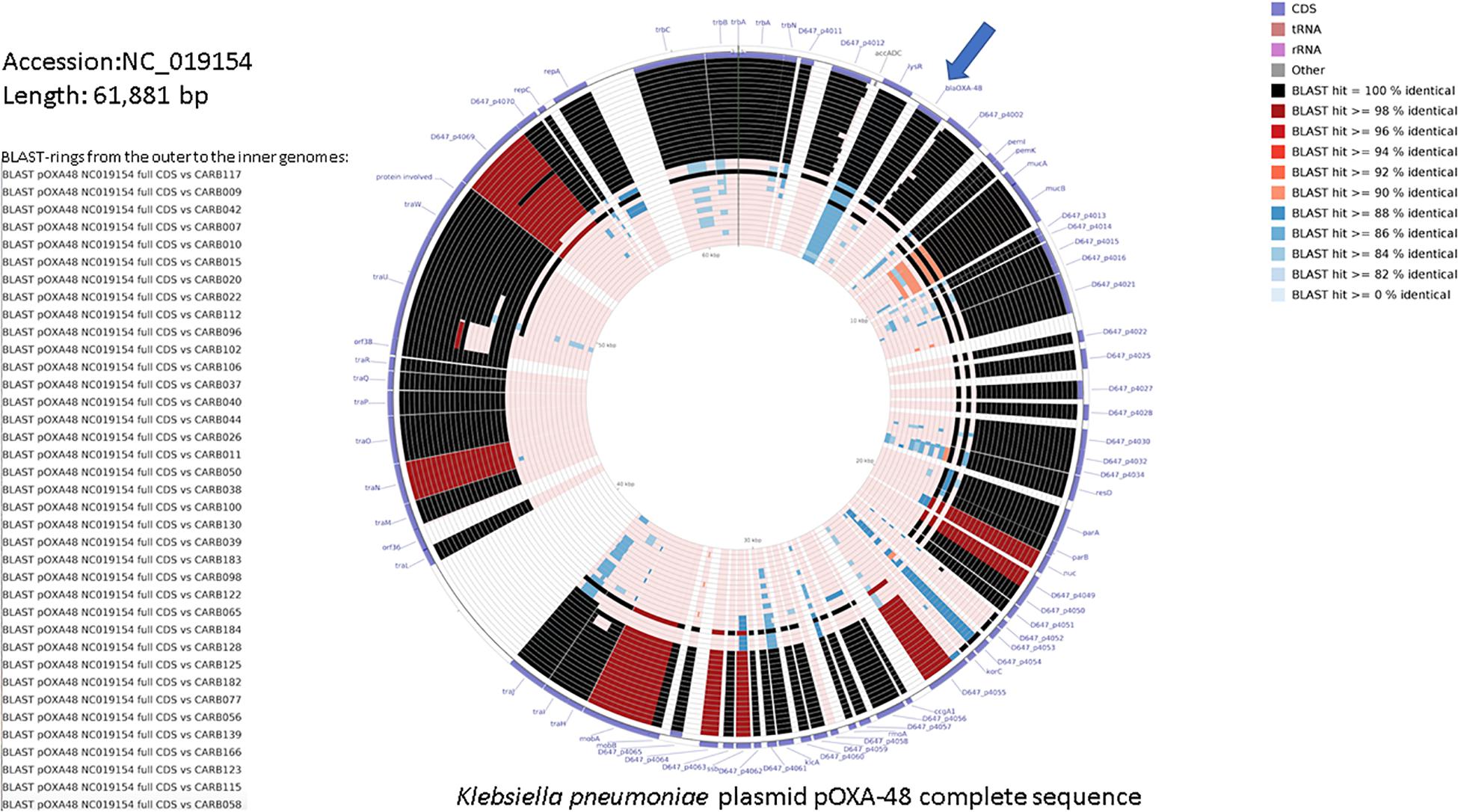
Figure 2. DNA comparison of the reference K. pneumoniae plasmid pOXA-48 (NC_019154.1) with assembled genomes using the CGView Comparison Tool (Grant et al., 2012). The legend on the left lists the 37 genome sequences used for the comparative BLAST-based analysis (genome names from the top to the bottom, and corresponding BLAST rings from the outer to the inner ones). The two outermost rings represent the forward and reverse coding strands of the plasmid reference genome. The color code for the coding strand features is highlighted in the legend on the bottom right. A separate BLAST ring is drawn for each comparison genome toward the inner part of the figure, and region of sequence similarity between the reference sequence and any part of the comparison sequence is shown in gradient color. blaOXA–48 gene is indicated with an arrow.
Nucleotide Sequence Accession Numbers
Raw reads used in this study have been deposited to the European Nucleotide Archive of the European Bioinformatics Institute under the project accession number PRJEB27508.
Discussion
WGS has a higher discriminatory power than do conventional typing methods and allows for a more precise outbreak analyses. This is especially important when analyzing the emergence and evolution of antimicrobial resistance, such as the threat of a worldwide spread of carbapenem resistance.
Previous studies described the molecular epidemiology of carbapenemase- and extended-spectrum beta-lactamase-producing K. pneumoniae isolates by WGS using a gene-by-gene comparison and/or SNP analysis (Snitkin et al., 2012; Lee et al., 2014; Marsh et al., 2015; Mathers et al., 2015; Onori et al., 2015; Zhou et al., 2015; Kluytmans-van den Bergh et al., 2016; Pérez-Vázquez et al., 2016; Zhou et al., 2016; Taboada et al., 2017). Here we combined both approaches, describing for the first time the cgMLST scheme included in SeqSphere+ Ridom software. In addition, we compared the results with those obtained with classical typing methods. For this purpose, we used a collection of OXA-48-producing K. pneumoniae isolates related to an increase in carbapenem resistance (ertapenem and imipenem) in Catalonia, which has been observed since 2012. The ST405 isolates were the most prevalent (77.6%) (Argente et al., 2019) and represented a predominant clone in Spain (Oteo et al., 2014). Previous bacterial-typing analysis of this collection by PFGE and MLST methods showed close genetic relatedness between all ST405 isolates, despite the fact that no clinical epidemiological relationships were established among patients.
First, we performed cgMLST and wgMLST using the BIGSdb-Kp and Ridom SeqSphere+ software tools, using a gene-by-gene approach. This reduced the analysis to coding regions but benefited from a stable nomenclature, which ensures interlaboratory reproducibility (Zhou et al., 2017). Second, we analyzed genome assemblies using the previously described Institut Pasteur cgMLST scheme (Bialek-Davenet et al., 2014), which already demonstrated its ability to subtype isolates with the same MLST denominations, and showed that MLST classification into traditional clonal complexes can merge members of distinct clonal groups (Bialek-Davenet et al., 2014). Last, we performed a cgSNP analysis of isolates of the same ST to investigate whether the isolates that did not show any allele differences by cgMLST were truly identical.
We observed two major clusters when using the cgMLST SeqSphere+ analysis. Cluster 1 included all isolates of the ST405/PFGE-E clone and comprised CTs 185, 1150, and 1153. Within cluster 1, three groups of two isolates each, each with different PFGE pattern subtypes E1/E16, E13/E12, and E17/E18 (groups a to c), showed no single allele difference using cgMLST and had a genetic distance below the threshold of 0.0035, confirming a close genetic relatedness (Kluytmans-van den Bergh et al., 2016). The PFGE and the cgMLST/wgMLST patterns were not the same, which may be explained by the fact that these two subtyping methods examine and distinguish different types of genetic mutation events (Zhou et al., 2017). Indeed, isolates could have mutations in the genome that do not affect restriction sites, and therefore, different cgMLST/wgMLST allelic profiles could have the same PFGE pattern (Tenover et al., 1995). Furthermore, PFGE diversity could be reflecting mostly changes in the accessory genome, for example, gain or loss of mobile genetic elements or recombination events, rather than true genealogies (Zhou et al., 2013).
In this study, ST405 isolates with identical cgMLST allelic profiles (SeqSphere+) showed between 6 and 17 (groups a, c, d) and 29 (group b) cgSNPs. These results are within the range of 1–77 SNPs that was previously described for ST405 isolates as sporadic (Onori et al., 2015). Other studies reported comparable results of cgMLST and SNP-based phylogeny in KPC-producing K. pneumoniae (Lee et al., 2014; Taboada et al., 2017). We observed 50 times more core SNPs between isolates CARB058 and CARB115 (both PFGE-A and ST101) than between isolates CARB058 and CARB077. These results were in concordance with cgMLST and wgMLST results, demonstrating again the higher discriminatory power of these two WGS-based approaches compared to PFGE and MLST.
One of the main challenges with the WGS-based typing methods (cgMLST, wgMLST, and cgSNP analysis) is to define cut-off values that can be used to identify a single-clone outbreak. Previous studies focusing on a gene-by-gene approach have used different cgMLST schemes (Lee et al., 2014; Treangen et al., 2014; Taboada et al., 2017; Zhou et al., 2017). Moreover, some authors have developed bioinformatic tools for an interactive identification of the most appropriate scheme (Silva et al., 2018). Taken together, this suggests that a definition of a unique threshold to define clonal relatedness is questionable. A previous study on K. pneumoniae established a maximum of 10 allele differences to cluster outbreak isolates and to separate unrelated out-group isolates when using a cgMLST scheme of 1,143 genes (Zhou et al., 2017). In this work, we applied two different cgMLST schemes, and both easily identified isolates with close genetic relatedness. However, the number of allele differences/allelic mismatches varied from 0 to 2 when using a 694-gene cgMLST scheme, and from 0 to 14 when using a 2,365-gene cgMLST scheme (Table 2), which indeed suggests that a different cluster alert distance should be applied depending on the cgMLST scheme. No threshold has been defined when using cgMLST from BIGSdb-Kp, but clearly, genetically related isolates are nearly identical (≤2 mismatches) when using this scheme. The analysis using the scgMLST BIGSdb-Kp scheme therefore provided complementary information that may be easier to interpret given the lower variation observed using this scheme.
We used two different thresholds in the gene-by-gene approach, a cluster alert distance of 15 from SeqSphere+ software (see text footnote 2) and a genetic distance of 0.0035 for cgMLST analysis described by Kluytmans-van den Bergh et al. (2016). Both proved to be useful for the description of genetic relatedness. In addition, the latter could be adopted independently of the cgMLST scheme used, which would also facilitate the interpretation of results, since it considers the proportion of shared genes by two isolates that are being compared. CARB100/CARB123 and CARB077/CARB115 isolates, with fewer than 14 allele differences (cluster alert distance 15), had a genetic distance above the threshold, which would have been interpreted as there being no genetic relatedness. However, when using the cgMLST BIGSdb-Kp scheme, the genetic distance was 0.0032 (2 allelic mismatches), indicating instead genetic relatedness when applying the same threshold. This illustrates that thresholds must be used with caution and cannot per se provide a definitive answer as to the genetic relatedness.
A threshold assessment is even more difficult for an SNP analysis; most authors analyzing K. pneumoniae isolates described the number of different SNPs detected, but no clear cut-off value has been assigned (Snitkin et al., 2012; Marsh et al., 2015; Mathers et al., 2015; Onori et al., 2015; Zhou et al., 2015; Pérez-Vázquez et al., 2016). Moreover, the number of SNPs detected depends on the reference genome used for the analysis and on the degree to which artifactual SNPs, due to paralogous sequences, for example, are filtered out during the SNP analysis. Some studies investigated the mutation rate of different species in order to define a threshold for the number of SNPs between related isolates (Köser et al., 2012; Zhou et al., 2013; Ruppitsch et al., 2015; Moura et al., 2017; Silva et al., 2018). Pérez-Vázquez et al. (2016) described an accumulation of approximately 11 SNPs per genome per year for OXA-48-K. pneumoniae isolates of ST405. Public Health England has published a reproducible and scalable pathogen population SNP-based analysis, including an “SNP address” nomenclature (Okoro et al., 2012).
The advantages of WGS-based typing are undeniable, however, “unique” or “one-fits-all” thresholds, by means of either gene-by-gene or SNP analysis, seem to be difficult to establish; it may be not only species-specific but also population-specific, which requires the careful and flexible application of proposed thresholds as previously discussed (Dallman et al., 2017). Nevertheless, efforts within the scientific community and public health authorities to unify the parameters and values used to define clonality would facilitate a common practice in data interpretation. This could be achieved by adopting a threshold range to be interpreted in combination with clinical epidemiological data and species population characteristics.
Regarding antimicrobial resistance mechanisms and possible vectors for the dissemination of those, we described 18 ARGs and the presence of up to four plasmids as possible disseminators of these. We established that quinolone resistance was not determined by mutations in topoisomerases as expected, but was probably due to the interplay between different mechanisms, i.e., plasmid factor QnrB66, the aminoglycoside-modifying enzyme AAC(6′)-Ib-cr, and the efflux pump OqxAB. The antibiotic resistance genotype profiles obtained by classical PCR (Argente et al., 2019) were mostly congruent with WGS results. Detection of genes by PCR, but not by WGS, could be explained by the loss of the plasmid harboring the corresponding gene or by incorrect assembly or low coverage of the respective gene (Leopold et al., 2014). Multiple factors could cause a negative PCR result, including inadvertently contamination of PCR tubes with glove power, which can inhibit steps during the PCR process (Schürch et al., 2018).
Our findings confirmed the conserved IncL plasmid as the major vector for the dissemination of OXA-48 carbapenemase. However, genetic rearrangements, such as deletions and point mutations, have also been observed within our 1-year isolate collection, reflecting genome plasticity and dynamics of horizontal gene transfer (Lomas et al., 1992). In addition, WGS allows us to identify the degree of homology between plasmid incompatibility groups, whereas by classical PBRT analysis, we only showed the presence or absence of the replicon genes. Nevertheless, the rapid advance in sequencing techniques will be decisive to describe plasmid features and sequences in accurate detail.
Conclusion
In conclusion, we observed an overall correlation between cgMLST and wgMLST, and cgSNP-analysis results to determine similarity of OXA-48-producing K. pneumoniae clinical isolates. CgMLST produced high-resolution allelic profiles and different cgMLST schemes provided complementary results that allowed for a better interpretation of results. Furthermore, cgSNP-based typing was useful to resolve and better discriminate OXA-48-producing K. pneumoniae clusters within the same ST. Differences between classical and WGS-based typing methods highlight the different molecular principle of these techniques, and we emphasize the importance to include clinical epidemiological data to elucidate clonal spread.
Data Availability Statement
The datasets generated for this study can be found in the ENA.
Author Contributions
EM, JR, and SG-C: conception and design. EM, SB, and SG-C: acquisition of data. EM and SG-C: laboratory analysis. EM, MC, VP, and SG-C: data analysis. EM, JR, MC, DH, SB, VP, and SG-C: data interpretation. EM and SG-C: writing of the draft manuscript. EM, JR, MC, DH, SB, VP, FN, AF, and SG-C: review of the manuscript.
Funding
This work was partly supported by Interreg IVa, EurSafety Health-net (III-1-02D73), and EurHealth-1Health projects, which are part of a Dutch-German cross-border network supported by the European Commission; the Dutch Ministry of Health, Welfare and Sport (VWS); the Ministry of Economy, Innovation, Digitalisation and Energy of the German Federal State of North Rhine-Westphalia; and the German Federal State of Lower Saxony. BIGSdb-Kp receives institutional funding from Institut Pasteur.
Conflict of Interest
JR consults for IDbyDNA.
The remaining authors declare that the research was conducted in the absence of any commercial or financial relationships that could be construed as a potential conflict of interest.
Acknowledgments
We want to thank the group of microbiologists at Hospitals Comarcals in Catalonia and the Balearic Islands (http://www.scmimc.org/grupstreball02.php) for collecting the clinical isolates and clinical epidemiological data: Carles Alonso (Hospital Creu Roja de l’Hospitalet), Frederic Ballester (Hospital Sant Joan de Reus), Carmen Gallés (Corporació de Salut del Maresme I la Selva), Carmina Martí (Hospital General de Granollers), Montserrat Morta (Hospital Sant Joan de Déu de Manresa), Montserrat Olsina (Hospital General Universitari de Catalunya-Quironsalud), Goretti Sauca (Hospital de Mataró), Montserrat Sierra (Hospital de Barcelona, SCIAS), Alba Rivera (Hospital de la Santa Creu i Sant Pau), and Anna Vilamala (Hospital General de Vic).
Supplementary Material
The Supplementary Material for this article can be found online at: https://www.frontiersin.org/articles/10.3389/fmicb.2019.02961/full#supplementary-material
FIGURE S1 | Minimum spanning tree (BioNumerics v6,7,3, Applied-Maths, Belgium) based on BIGSdb-Kp scgMLST allelic profile comparisons. Each circle represents a single profile. The number of allelic mismatches among profiles are given along links (excluding missing data in one or both profiles). The large circle corresponds to the dominant genotype.
FIGURE S2 | (A) SNP-based tree of ST405 isolates using the Neighbour-Joining method. The genome reference is the first ST405 isolate collected (CARB007-E1). The number of SNPs compared to the reference (*) for the isolates that clustered together using a gene-by-gene approach (groups a, b, c and d) is indicated. The number of SNPs between these isolates themselves is also indicated in brackets. (B) SNP-based tree of ST101 isolates using the Neighbour-Joining method. The genome reference was the first ST101 isolate collected (CARB0058-A3). The number of SNPs compared to the reference (*) is indicated.
FIGURE S3 | Phylogenetic tree based on cgMLST-SeqSphere+ (2,365 targets) and BLASTn comparison of de novo assembled genomes with K. pneumoniae plasmid pOXA-48 NC_019154.1 with at least 80% sequence similarity using DNAplotter (Carver et al., 2009) and visualized using Artemis Comparison Tool (ACT) (Carver et al., 2005). Information of hospital, isolation, and date of isolation is also included.
TABLE S1 | Assembly quality using QUAST 4.5.
TABLE S2 | PlasmidFinder v.1.3 and PBRT-PCR results.
DATA SHEET S1 | CgMLST distance matrices for pairwise comparisons of 37 OXA-48 K. pneumoniae isolates.
DATA SHEET S2 | VCF files for ST101, including SNP, indel, and structural variation calls information generated by Harvest software suuite (parsnp) (Treangen et al., 2014).
Footnotes
- ^ https://bigsdb.pasteur.fr/
- ^ https://www.ridom.de/u/Core_Genome_MLST_Complex_Type.html
- ^ https://bigsdb.pasteur.fr/klebsiella
References
Argente, M., Miró, E., Vilamala, A., Marti, C., Alonso-Tarres, C., Ballester, F., et al. (2019). Molecular characterization of OXA-48 carbapenemase-producing Klebsiella pneumoniae strains after an increase in carbapenem resistance in Catalonia. Enferm. Infecc. Microbiol. Clin. 37, 82–88. doi: 10.1016/j.eimc.2018.02.003
Bialek-Davenet, S., Criscuolo, A., Ailloud, F., Passet, V., Jones, L., Delannoy-Vieillard, A. S., et al. (2014). Genomic definition of hypervirulent and multidrug-resistant Klebsiella pneumoniae clonal groups. Emerg. Infect. Dis. 20, 1812–1820. doi: 10.3201/eid2011.140206
Brisse, S., Milatovic, D., Fluit, A. C., Verhoef, J., Martin, N., Scheuring, S., et al. (1999). Comparative in vitro activities of ciprofloxacin, clinafloxacin, gatifloxacin, levofloxacin, moxifloxacin, and trovafloxacin against Klebsiella pneumoniae, Klebsiella oxytoca, Enterobacter cloacae, and Enterobacter aerogenes clinical isolates with alterations in GyrA and ParC proteins. Antimicrob. Agents Chemother. 43, 2051–2055. doi: 10.1128/aac.43.8.2051
Carattoli, A., Zankari, E., Garcia-Fernandez, A., Voldby-Larsen, M., Lund, O., Villa, L., et al. (2014). In silico detection and typing of plasmids using PlasmidFinder and plasmid multilocus sequence typing. Antimicrob. Agents Chemother. 58, 3895–3903. doi: 10.1128/AAC.02412-14
Carver, T. J., Rutherford, K. M., Berriman, M., Rajandream, M. A., Barrell, B. G., and Parkhill, J. (2005). ACT: the artemis comparison tool. Bioinformatics 21, 3422–3423. doi: 10.1093/bioinformatics/bti553
Carver, T. J., Thomson, N., Bleasby, A., Berriman, M., and Parkhill, J. (2009). DNAPlotter: circular and linear interactive genome visualization. Bioinformatics 25, 119–120. doi: 10.1093/bioinformatics/btn578
Dallman, T., Ashton, P., Schafer, U., Jironkin, A., Painset, A., Shaaban, S., et al. (2017). SnapperDB: a database solution for routine sequencing analysis of bacterial isolates. Bioinformatics 34, 3028–3029. doi: 10.1093/bioinformatics/bty212
Diancourt, L., Passet, V., Verhoef, J., Grimont, P. A., and Brisse, S. (2005). Multilocus sequence typing of Klebsiella pneumoniae nosocomial isolates. J. Clin. Microbiol. 43, 4178–4182. doi: 10.1128/jcm.43.8.4178-4182.2005
Grant, J. R., Arantes, A. S., and Stothard, P. (2012). Comparing thousands of circular genomes using the CGView comparison tool. BMC Genomics 13:202. doi: 10.1186/1471-2164-13-202
Gurevich, A., Saveliev, V., Vyahhi, N., and Tesler, G. (2013). QUAST: quality assessment tool for genome assemblies. Bioinformatics 29, 1072–1075. doi: 10.1093/bioinformatics/btt086
Kluytmans-van den Bergh, M. F., Rossen, J. W., Bruijning-Verhagen, P. C., Bonten, M. J., Friedrich, A. W., Vandenbroucke-Grauls, C. M., et al. (2016). Whole genome multilocus sequence typing of extended-spectrum beta-lactamase-producing Enterobacteriaceae. J. Clin. Microbiol. 54, 2919–2927. doi: 10.1128/jcm.01648-16
Köser, C. U., Holden, M. T., Ellington, M. J., Cartwright, E. J., Brown, N. M., Ogilvy-Stuart, A. L., et al. (2012). Rapid whole-genome sequencing for investigation of a neonatal MRSA outbreak. N. Engl. J. Med. 366, 2267–2275. doi: 10.1056/NEJMoa1109910
Larsen, M. V., Consentino, S., Rasmussen, S., Friis, C., Hasman, H., Lykke Marvig, R., et al. (2012). Multilocus sequence typing of total-genome-sequenced bacteria. J. Clin. Microbiol. 50, 1355–1361. doi: 10.1128/JCM.06094-11
Lee, Y., Kim, B. S., Chun, J., Yong, J. H., Lee, Y. S., Yoo, J. S., et al. (2014). Clonality and Resistome analysis of KPC-producing Klebsiella pneumoniae strain isolated in Korea using whole genome sequencing. Biomed. Res. Int. 2014:352862. doi: 10.1155/2014/352862
Leopold, S. R., Goering, R. V., Witten, A., Harmsen, D., and Mellmann, A. (2014). Bacterial whole-genome sequencing revisited: portable, scalable, and standardized analysis for typing and detection of virulence and antibiotic resistance genes. J. Clin. Microbiol. 52, 2365–2370. doi: 10.1128/JCM.00262-14
Lomas, J. G., Sunzeri, F. J., and Busch, M. P. (1992). False-negative results by polymerase chain reaction due to contamination by glove powder. Transfusion 32, 83–85. doi: 10.1046/j.1537-2995.1992.32192116439.x
Maiden, M. C. J., Jansen van Rensburg, M. J., Bray, J. E., Earle, S. G., Ford, S. A., Jolley, K. A., et al. (2013). MLST revisited: the gene-by-gene approach to bacterial genomics. Nat. Rev. Microbiol. 11, 728–736. doi: 10.1038/nrmicro3093
Marsh, J. W., Krauland, M. G., Nelson, J. S., Schlackman, J. L., Brooks, A. M., Pasculle, A. W., et al. (2015). Genomic epidemiology of an endoscope-associated outbreak of Klebsiella pneumoniae carbapenemase (KPC)-producing K. pneumoniae. Plos One 10:e0144310. doi: 10.1371/journal.pone.0144310
Mathers, A. J., Stoesser, N., Sheppard, A. E., Pankhurst, L., Giess, A., Yeh, A. J., et al. (2015). Klebsiella pneumoniae carbapenemase (KPC)-producing K. pneumoniae at a single institution: insights into endemicity from whole-genome sequencing. Antimicrob. Agents Chemother. 59, 1656–1663. doi: 10.1128/AAC.04292-14
Moura, A., Tourdjman, M., Leclercq, A., Hamelin, E., Laurent, E., Fredriksen, N., et al. (2017). Real-time whole-genome sequencing for surveillance of Listeria monocytogenes. France. Emerg. Infect. Dis. 23, 1462–1470. doi: 10.3201/eid2309.170336
Okoro, C. K., Kingsley, R. A., Connor, T. R., Harris, S. R., Parry, C. M., Al-Mashhadani, M. N., et al. (2012). Intracontinental spread of human invasive Salmonella typhimurium pathovariants in sub-Saharan Africa. Nat. Genet. 44, 1215–1221. doi: 10.1038/ng.2423
Onori, R., Gaiarsa, S., Comandatore, F., Pongolini, S., Brisse, S., Colombo, A., et al. (2015). Tracking nosocomial Klebsiella pneumoniae infections and outbreaks by whole-genome analysis: small-scale Italian scenario within a single hospital. J. Clin. Microbiol. 53, 2861–2868. doi: 10.1128/JCM.00545-15
Oteo, J., Miro, E., Pérez-Vázquez, M., and Navarro, F. (2014). Evolution of carbapenemase-producing Enterobacteriaceae at the global and national level: what should be expected in the future? Enferm. Infecc. Microbiol. Clin. 32, 17–23. doi: 10.1016/S0213-005X(14)70170-3
Pérez-Vázquez, M., Oteo, J., García-Cobos, S., Aracil, B., Harris, S. R., Ortega, A., et al. (2016). Phylogeny, resistome and mobile genetic elements of emergent OXA-48 and OXA-245 Klebsiella pneumoniae clones circulating in Spain. J. Antimicrob. Chemother. 71, 887–896. doi: 10.1093/jac/dkv458
Rossen, J. W. A., Friedrich, A. W., and Moran-Gilad, J., Escmid Study Group for Genomic, and Molecular Diagnostics (Esgmd). (2018). Practical issues in implementing whole-genome-sequencing in routine diagnostic microbiology. Clin. Microbiol. Infect 24, 355–360. doi: 10.1016/j.cmi.2017.11.001
Ruppitsch, W., Pietzka, A., Prior, K., Bletz, S., Fernandez, H. L., Allerberger, F., et al. (2015). Defining and evaluating a core genome multilocus sequence typing scheme for whole-genome sequence-based typing of Listeria monocytogenes. J. Clin. Microbiol. 53, 2869–2876. doi: 10.1128/JCM.01193-15
Schürch, A. C., Arredondo-Alonso, S., Willems, R. J. L., and Goering, R. V. (2018). Whole genome sequencing options for bacterial strain typing and epidemiologic analysis based on single nucleotide polymorphism versus gene-by-gene-based approaches. Clin. Microb. Infect. 24, 350–354. doi: 10.1016/j.cmi.2017.12.016
Seemann, T. (2014). Prokka: rapid prokaryotic genome annotation. Bioinformatics 30, 2068–2069. doi: 10.1093/bioinformatics/btu153
Silva, M., Machado, M., Silva, D., Rossi, M., Moran-Gilad, J., Santos, S., et al. (2018). chewBBACA: a complete suite for gene-by-gene schema creation and strain identification. Microb. Genom. 4:e000166. doi: 10.1099/mgen.0.000166
Snitkin, E. S., Zelazny, A. M., Thomas, P. J., Stock, F. Nisc Comparative Sequencing Program, Henderson, D. K., et al. (2012). Tracking a hospital outbreak of carbapenems-resistant Klebsiella pneumoniae with whole-genome sequencing. Sci. Transl. Med. 4:148ra116. doi: 10.1126/scitranslmed3004129
Taboada, E. N., Graham, M. R., Carriço, J. A., and Van Domselaa, R. G. (2017). Food safety in the age of next generation sequencing, bioinformatics, and open data access. Front. Microbiol. 8:909. doi: 10.3389/fmicb.2017.00909
Tenover, F. C., Arbeit, R. D., Goering, R. V., Mickelsen, P. A., Murray, B. E., Persing, D. H., et al. (1995). Interpreting chromosomal DNA restriction patterns produced by pulsed-field gel electrophoresis: criteria for bacterial strain typing. J. Clin. Microbiol. 33, 2233–2239.
Treangen, T. J., Ondov, B. D., Koren, S., and Phillippy, A. M. (2014). The Harvest suite for rapid core-genome alignment and visualization of thousands of intraspecific microbial genomes. Genome Biol. 15:524. doi: 10.1186/preaccept-2573980311437212
Zankari, E., Hasman, H., Cosentino, S., Vestergaard, M., Rasmussen, S., Lund, O., et al. (2012). Identification of acquired antimicrobial resistance genes. J. Antimicrob. Chemother. 67, 2640–2644. doi: 10.1093/jac/dks261
Zhao, F., Bai, J., Wu, J., Liu, J., Zhou, M., Xia, S., et al. (2010). Sequencing and genetic variation of multidrug resistance plasmids in Klebsiella pneumoniae. PLoS One 5:e10141. doi: 10.1371/journal.pone.0010141
Zhou, H., Liu, W., Qin, T., Liu, C., and Ren, H. (2017). Defining and evaluating a core genome multilocus sequence typing scheme for whole-genome sequence-based typing of Klebsiella pneumoniae. Front. Microbiol. 8:371. doi: 10.3389/fmicb.2017.00371
Zhou, K., Lokate, M., Deurenberg, R. H., Arends, J., Lo-Ten Foe, J., Grundmann, H., et al. (2015). Characterization of a CTX-M-15 Producing Klebsiella pneumoniae Outbreak Strain Assigned to a Novel Sequence Type (1427). Front. Microbiol. 6:1250. doi: 10.3389/fmicb.2015.01250
Zhou, K., Lokate, M., Deurenberg, R. H., Tepper, M., Arends, J. P., Raangs, E. G., et al. (2016). Use of whole-genome sequencing to trace, control and characterize the regional expansion of extended-spectrum β-lactamase producing ST15 Klebsiella pneumoniae. Sci. Rep. 6:20840. doi: 10.1038/srep20840
Keywords: K. pneumoniae, OXA-48, cgMLST, wgMLST, WGS, molecular epidemiology
Citation: Miro E, Rossen JWA, Chlebowicz MA, Harmsen D, Brisse S, Passet V, Navarro F, Friedrich AW and García-Cobos S (2020) Core/Whole Genome Multilocus Sequence Typing and Core Genome SNP-Based Typing of OXA-48-Producing Klebsiella pneumoniae Clinical Isolates From Spain. Front. Microbiol. 10:2961. doi: 10.3389/fmicb.2019.02961
Received: 10 September 2019; Accepted: 09 December 2019;
Published: 31 January 2020.
Edited by:
Ruiting Lan, University of New South Wales, AustraliaReviewed by:
Yvonne Pfeifer, Robert Koch Institute, GermanyJane Fiona Turton, Public Health England, United Kingdom
Copyright © 2020 Miro, Rossen, Chlebowicz, Harmsen, Brisse, Passet, Navarro, Friedrich and García-Cobos. This is an open-access article distributed under the terms of the Creative Commons Attribution License (CC BY). The use, distribution or reproduction in other forums is permitted, provided the original author(s) and the copyright owner(s) are credited and that the original publication in this journal is cited, in accordance with accepted academic practice. No use, distribution or reproduction is permitted which does not comply with these terms.
*Correspondence: Elisenda Miro, emiro@santpau.cat; S. García-Cobos, s.garcia.cobos@umcg.nl
†These authors have contributed equally to this work
‡Present address: S. García-Cobos, Reference and Research Laboratory on Antimicrobial Resistance and Healthcare-Associated Infections, National Microbiology Center, Institute of Health Carlos III, Madrid, Spain