- 1School of Geography and Resource Science, Neijiang Normal University, Neijiang, China
- 2Neijiang Center for Disease Control and Prevention, Neijiang, China
- 3School of Economics and Management, Neijiang Normal University, Neijiang, China
- 4Tuojiang River Basin High-Quality Development Research Center, Neijiang, China
Public concern over major agricultural product safety incidents, such as swine flu and avian flu, can intensify financial losses in the livestock and poultry industries. Crawler technology were applied to reviewed the Weibo social media discussions on the African Swine Fever (ASF) incident in China that was reported on 3 August 2018, and used content analysis and network analysis to specifically examine the online public opinion network dissemination characteristics of verified individual users, institutional users and ordinary users. It was found that: (1) attention paid to topics related to “epidemic,” “treatment,” “effect” and “prevent” decrease in turn, with the interest in “prevent” increasing significantly when human infections were possible; (2) verified individual users were most concerned about epidemic prevention and control and play a supervisory role, the greatest concern of institutional users and ordinary users were issues related to agricultural industry and agricultural products price fluctuations respectively; (3) among institutional users, media was the main opinion leader, and among non-institutional users, elites from all walks of life, especially the food safety personnel acted as opinion leaders. Based on these findings, some policy suggestions are given: determine the nature of the risk to human health of the safety incident, stabilizing prices of relevant agricultural products, and giving play to the role of information dissemination of relevant institutions.
Introduction
The last decade had seen several public health food safety incidents, such as swine flu in North America in 2009 (Garten Rebecca et al., 2009), avian influenza in China in 2013 (Gao et al., 2013) and the African swine fever (ASF) outbreak in 2018 (Zhou et al., 2018), all of which directly impacted the livestock and poultry industries and raised public health concerns over food safety, which in turn indirectly led to price volatility in pork, eggs, and other related agricultural products (Chmielewski and Swayne, 2011; Hassouneh et al., 2012; Wen et al., 2019; Yi et al., 2019b). ASF, a highly contagious viral hemorrhagic disease of swine, appeared and spread in most parts of the world, with significant economic implications for the pig industry and pork prices (Zhao et al., 2022a). As China is the world’s largest pork producer and consumer, accounting for about 50% of the global pork supply, ASF had a huge impact on China’s agricultural economy (Muñoz and Tabarés, 2022). From October 2018 to September 2019, pork prices rose 85.7% year-on-year in China (Xu et al., 2022). Effective prevention and control of the epidemic is an inevitable trend to ensure sustainable social and economic development.
Extensive media and social media coverage quickly made the public aware of these major agricultural product safety incidents and played a significant role in fanning the flames and expanding the economic impacts (Wen et al., 2019; Yi et al., 2019b). In the past, information about agricultural product safety incidents was mainly accessed from traditional media, such as newspapers, radio, and television (De Jonge et al., 2010; Attavanich et al., 2011; Carducci et al., 2011; Hilton and Hunt, 2011; Vasterman and Ruigrok, 2013); however, the rise in social media means that people are no longer unilaterally receiving messages from official organizations but are participating in the information dissemination (Neuman et al., 2014).
Due to its ease of use, timeliness and openness, Twitter has become a major global platform for spreading information and expressing opinions (Simon et al., 2015). However, in China, Weibo, which is similar to Twitter, is the most widely used social media (Fung et al., 2013; Song et al., 2020), with 462 million monthly active users in 2018 and 130 million, 120 million, and 1.5 million average daily texts, pictures, and video/live streams (The 2018 Weibo User Development Report). Therefore, the latest news on agricultural product safety incidents can be rapidly spread on Weibo; however, the excessive spread of unverified news and rumors can result in panic and huge economic losses (Gu et al., 2014; Martínez-Rojas et al., 2018; Stieglitz et al., 2018). The ASF epidemic in China has caused huge online discussion, causing a great deal of misinformation and panic. Timely understanding online public discussion topics and communication characteristics in ASF events is not only of great significance for relevant institutions to effectively guide and control public opinions but also provides targeted directions for epidemic prevention and control.
Therefore, because it was a recent Chinese food safety and public health incident, the ASF Weibo content from 2 August 2018 to 2 August 2019 was studied to: (1) analyze the discussion and ASF information sharing by different Weibo user types; and (2) analyze the social network structures of the Weibo users discussing the ASF.
The remainder of this paper is organized as follows: section Literature Review reviews the online public opinion research on agricultural product safety incidents, section Research Background gives the background to the ASF outbreak, section Research Design and Method details the research steps and methods, section Results and Discussion discusses the analysis results, and section Conclusions and Recommendations gives the conclusions and recommendations.
Literature Review
The agricultural product safety social media discussions were summarized under three main headings: content analysis of social media discussions; network analysis of social media discussions; and the main social media discussion subjects/stakeholders.
Content Analysis of Social Media Discussions
Social media data are mostly unstructured textual data that need to be converted into structured data for analysis (Lipizzi et al., 2015; Aswani et al., 2018), with most previous studies having adopted manual content analyses that divided the text content into predetermined types using manual coding (Collier et al., 2010; Ding and Zhang, 2010; Gao et al., 2011; Tirkkonen and Luoma-aho, 2011; Freberg et al., 2013; Luoma-Aho et al., 2013). For example, Chew and Eysenbach (2010) analyzed the 2009 swine flu Twitter discussions using manual content analysis and divided the tweets into several types, such as resources, personal experience, personal opinions, and interests. However, this approach can be relatively subjective and the number of tweets that can be analyzed is limited. Computerized automatic content analyses can also be employed to assess the topics, sentiments, and other content in large amounts of text, with the most common methods being topical analysis, semantic network analysis, sentiment analysis, and machine learning methods (Krippendorff, 2004) such as support vector machines (SVM) and Naive Bayes (Lyons and Lăzăroiu, 2020; Mircică, 2020; Stanley and Kucera, 2021). Content analysis of social media is often applied in the analysis of travel decisions. Pop et al. (2022) showed that consumers’ trust in social media influencers had a positive impact on all stages of travel decision-making. Hou et al. (2019) extracted subject terms from reviews of three major online travel agencies in China and constructed semantic association networks to distill five aspects of tour guides, hotels, services, scenic spots, and experiences that were of concern to tourists. All these studies have reflected the importance of social media for travel decisions, and tourism companies, online travel agencies, and hotels could use social media to guide consumption decisions and achieve greater benefits.
In addition, text mining has often been applied to the study of social media discussions of emergencies. Kim et al. (2018) conducted a textual analysis of Twitter content before and after Storm Cindy in the United States in 2017 and found that negative words such as “threatening” and “scary” appeared more frequently after the event than before it, thus assuming that evolving words over time can suggest the extent of the event. Pourebrahim et al. (2019) studied Twitter texts before, during, and after Hurricane Sandy and further conducted a sentiment analysis, reaching similar conclusions. Furthermore, their analysis of word co-occurrence revealed that during disasters, emotional tweets are increasingly used to release anxiety and provide a sense of connection between people experiencing similar situations, while relief and donation-related clusters become major topics of discussion in the aftermath of disasters. In response to public health events, Hellsten et al. (2019) examined Twitter discussions on avian influenza in the Netherlands from 2015 to 2017, applying automated network analysis to examine the co-occurrence of passive stakeholders and thematic networks. In particular, the global pandemic of COVID-19 since 2020 has been followed by social media discussions during the epidemic. Some studies have focused on social media discussions of specific issues during the outbreak, including willingness to vaccinate (Griffith et al., 2021; Hussain et al., 2021) and discussions of older adults and COVID-19 (Jimenez-Sotomayor et al., 2020; Xiang et al., 2021). Others were concerned about topics of public concern and changes in sentiment in social media discussions, and these studies helped government and public health agencies to better communicate with the public, stabilize public sentiment, and combat the spread of fake news (Abd-Alrazaq et al., 2020; Han et al., 2020; Zhao et al., 2020; Jang et al., 2021).
Network Analysis of Social Media Discussions
Social media communication patterns have also attracted significant recent research attention (Nair et al., 2017; Kim et al., 2018; Vijaykumar et al., 2018), with the nodes in a social media network representing the users and the connections, such as following, commenting, reposting or retweeting, representing the user relationships. Centrality has also been highlighted as an important social network concept, with the higher the centrality, the more important the node’s role in the network, that is, users with high centrality generally attract higher social media network attention. For example, Kim et al. (2018) used social network analysis to investigate the information network and diffusion during Hurricane Cindy in the United States in 2017 and found that news and meteorological agencies were the main information sources, while the public and organizations were the main information disseminators. Similarly, Pourebrahim et al. (2019) studied Twitter discussion of Hurricane Sandy and found that the connections between key influencers from different fields such as politics, news, weather and relief organizations and their followers played a crucial role in information sharing and dissemination, which provide an effective tool for emergency managers to establish two-way communication during disasters. Closely related to this study, Sun et al. (2020) similarly studied the information dissemination patterns of Weibo during the African swine fever in 2018 and the results showed that different types of users play different roles, such as providing information, amplifying information, delivering information and engaging other users, and its beneficial for government agencies and public health authorities to effectively disseminate information.
Agricultural Product Safety Incident Social Media Subjects/Stakeholders
Social media discussion research on emergencies/crises often involve three main subjects: the government, the network media and the netizens. Emergencies/crises tend to generate public sentiment, with the network media promoting the discussion development; however, at the same time, the government attempts to supervise and actively guide public sentiment (Li et al., 2020). Therefore, research into the different subjects/stakeholders can elucidate various responses. Past research has tended to adopt manual coding to identify social media subjects/stakeholders. For example, Hellsten et al. (2019) divided 122 Twitter users into media, citizens, environmental organizations, conventional industries, eco-industries, public organizations, political actors, and others using manual coding. However, manual coding has a certain subjectivity and can only be used if the number of users is relatively small; therefore, automatic coding has been found to be more scientific and efficient for large amounts of data. As Weibo authentication divides users into institutional users (official), verified individual users (well-known figures) and unverified individual users (ordinary users), the information can be easily divided into three groups when information mining.
To sum up, first of all, the existing studies on social media discussion of emergencies mostly focus on disaster events. Since the outbreak of COVID-19, public health events have attracted increasing attention. However, there are still few studies on agricultural product safety events. Therefore, this study focuses on the social media discussion of African Swine fever (ASF) in China, an agricultural product safety event, based on Weibo platform. Secondly, existing social media discussions on agricultural product safety incidents have tended to adopt manual content analysis, which had resulted in problems such as small sample sizes and subjective classifications. In this study, automatic coding was applied using information mining on the Weibo ASF related users, as well as automatic content analysis methods such as topic analysis, semantic network analysis, and sentiment analysis were applied to study the ASF discussion developments in the three user groups. Finally, in addition to the estimation and prediction of cases through social media data (Signorini et al., 2011; Chen et al., 2019; Masri et al., 2019), there was a lack of research into the social media propagation patterns of such incidents. From the perspective of communication network, a network analysis method was applied to study the ASF public opinion network dissemination characteristics and identify the opinion leaders and dissemination bridges.
Research Background
Since the first case of ASF was detected in Shenyang, Liaoning province on 3 August 2018, it continued to spread in China, with 75 cases identified by the end of 2019 in all of China’s 31 mainland provinces/autonomous regions/municipalities. Because of its high lethality, from the initial outbreak, ASF has had a significant impact on the market price of pigs and related products in China (Hu and Guo, 2018). The price trends for dressed pork nationwide since May 2018 are shown in Figure 1.
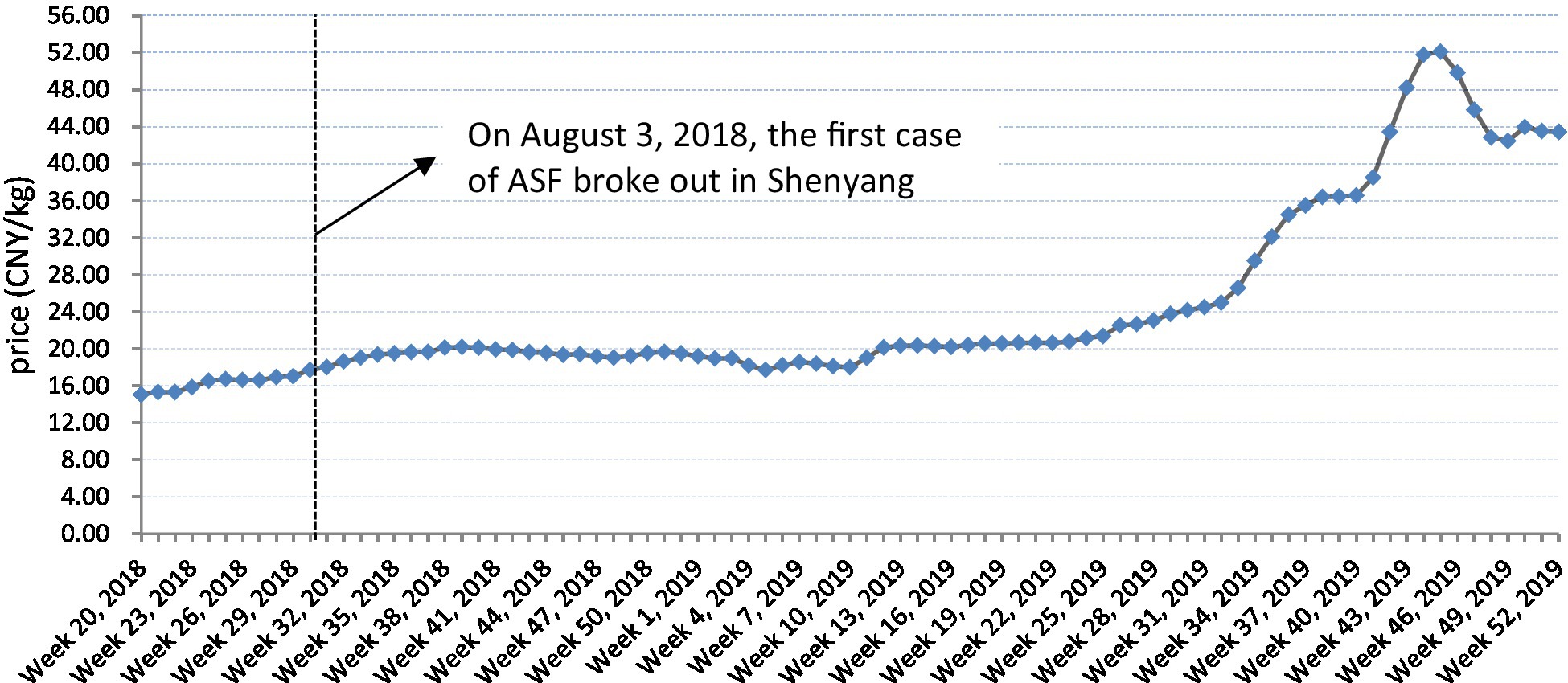
Figure 1. Changes in pork prices in mainland China since May 2018. Data source: China animal husbandry information network.
As can be seen, during the initial ASF outbreak, there was no significant change in the national pork prices, which was probably because of regional short-term price fluctuation differences. Farmers in the main live pig producing areas sold a great deal of their stock to avoid the risks, with this sudden oversupply leading to price decreases; while the prices in the main sales areas for live pigs increased because of transportation restrictions. However, over the longer term, pork prices in late 2019 were about three times as high as before the ASF outbreak because of the lack of supply and the pull of demand.
The Baidu index (like google search index), which reflects the number of Baidu searches, can be used to measure public interest in the ASF outbreak (Gu et al., 2014; Towers et al., 2015; Yi et al., 2019a). The results from a search-term index (keyword: African swine fever) from 2 August 2018 to 2 August 2019 were examined and the events corresponding to each peak identified, as shown in Figure 2.
Research Design and Method
In order to fully grasp the online public discussion and communication characteristics on ASF, to implement more targeted public opinion guidance and control measures and maintain a healthy social network environment, content analysis, and network analysis methods were applied to online public opinion content to identify the ASF public opinion transmission characteristics. Figure 3 summarizes the study research process. First, a web crawler to identify ASF-related content in Weibo and Weibo-reposting-network. Then, pre-processed textual data: data cleansing, tokenization, stop word removal, and translation: using Jieba, the Python Chinese word segmentation natural language processing (NLP) module (Hou et al., 2019), after which classified subject words and statistically analyzed, semantic association analysis, and sentiment analysis performed on the processed textual data, and social network analysis performed on the reposted network data.
Data Collection and Pre-processing
Data Collection
To analyze the online public discussion contents and the public opinion communication network structure, data need to be collected from the following two parts: Weibo texts containing the key “African swine fever” phrase; and user network data from the reposting of the Weibo posts.
Search “African Swine Fever” Weibo Post Information
To compare the differences between different user groups in the discussion focus on ASF events, the discussion content, users participating in the discussion and relevant information were collected. First, temporal logic was used to search for the original Weibo African swine fever keyword information and acquire the corresponding microblog content addresses. The time period was set from 2 August 2018, when the first ASF occurred in China, to 2 August 2019. Then, a public free Python Crawler Technology was used to automatically collect the information from the web pages and extract the microblog information: poster ID, authentication information, repost number, content, release date, poster’s gender, location, and other information.
Collect the Weibo Reposting Relationships
To explore the status and role of different user groups in online public opinion communication, reposting relationships data were collected. If all reposted posts were collected, there was a high possibility that most would have come from a discussion high heat period. Therefore, to ensure the data reliability, first, the search indexes were ranked from large to small, with the first third of the search indexes corresponding to discussion high heat periods and the last third corresponding to discussion low heat periods (Figure 2). Based on the proportion of the total Weibo posts in each period, 25, 8, and 4 microblogs were, respectively, randomly selected from the top 3% Weibo reposted posts in the discussion high, medium, and low heat periods. Then, the Python crawler technology was allowed to retrieve the original posters’ IDs and their re-posts (Bird et al., 2009).
Data Pre-processing
It is necessary to pre-process data to obtain standard-format data for further analysis because the collected data is often cluttered and nonstandard (Martínez-Rojas et al., 2018; Song et al., 2020). On the one hand, the Weibo posts were divided into three categories based on the origins, after which each category was pre-processed over four steps: data cleaning, word segmentation, stop word removal and translation. First, the purpose of data cleansing is to filter out useless information to reduce its impact on analysis results. After the data cleaning, 82,203 Weibo posts were collected: 12,659 from verified individual users; 21,015 from institutional users; and 48,529 from ordinary users. Second, as Chinese has no obvious blanks in sentences (Shuang et al., 2018), word segmentation was then required on the collected Weibo texts. Jieba, the most widely used Chinese word segmentation component, was used to implement the tokenization and stop word removal, which are words that plays a cohesive role in a sentence and has no real value to the text meaning. Finally, the first 200 Chinese keywords for each kind of user were selected and translated into English. On the other hand, the reposting relationships between users were calculated. For example, once A reposted B’s post, a line with a weight of 1 was created pointing from B to A. Therefore, the many reposting relationships between the Weibo users constituted the public opinion communication network. To avoid multiple calculations, only direct reposts were calculated. For example, in A → B → C relation, A → B, B → C direct reposts were calculated, while A → C indirect repost was not counted.
Content Analysis
The purpose of content analysis is to identify the focus and emotional changes of online users on ASF events. Content analysis method, which is an extensive set of natural language processing (NLP) and text mining techniques (Chau and Xu, 2012), was used to analyze “unstructured” text data from the perspective of topic analysis and sentiment analysis and to compare the differences in discussion and sentiment among different user groups.
Topic Analysis
The purpose of topic analysis is to reveal the different user groups discussion focus. The first part of the ASF Weibo data analysis was to identify the thematic words, which were the concise, timely and informative words that had certain meanings (Hou et al., 2019), and apply the thematic analysis to calculate the keyword frequencies in the texts. The second part of the ASF Weibo data analysis was the semantic association analysis. This study used Bigrams in NLTK Chinese corpus to generate semantically related binary co-occurrence phrases. After tokenization, the binary co-occurrence frequencies of the adjacent words from the three users’ microblog texts were, respectively, calculated to identify the key phrases and the main discussion topics (Chae, 2015).
Sentiment Analysis
The purpose of sentiment analysis is to reveal the sentiment evolution trend of different user groups. The subjective information in Weibo posts was classified (Feldman, 2013) and each post was classified as negative, neutral, or positive. While there are different techniques and tools for sentiment analysis (Pang and Lee, 2008), SentiStrength was chosen as the sentiment analysis algorithm because it was originally designed for informal texts such as tweets (Thelwall et al., 2011). Therefore, every post sentiment was measured, with scores greater than 0 representing positive emotions, those equal to 0 representing neutral emotions, and those less than 0 representing negative emotions.
Social Network Analysis
In order to guide network users’ communication behavior, social network analysis is necessary. Social network analysis, which is based on mathematical methods and graph theory (Otte and Rousseau, 2002; Prell, 2012) and can identify the position and role of different user groups in public opinion communication according to the reposting relationship between users, was specifically developed to construct ASF public opinion communication network, reveal network structure, and visualize these network relationships using software programs such as “Ucinet” and “Gephi,” thereby allowing for an intuitive analysis of the node behavior and a deeper understanding of the network structure. Various network metrics provide a detailed description of the network, the specific descriptions and calculation methods for which are shown in Table 1.
Results and Discussion
General Overview
The number of posts reflect the public attention on the incident (Mollema et al., 2015; Househ, 2016). However, as gender differences could have a significant impact on attention to public health events, and the number of posts from different regions reflects the regional discussion heat, the collected gender and address information of the 82,203 Weibo posters was used to determine the gender differences and the ASF discussion concentrations. As shown in Figure 4, the number of Weibo discussion users decreased from east to west. As the eastern region was more involved in the discussion region, Beijing, Liaoning, Sichuan, Guangdong, Jiangsu, and Shanghai were placed on the first level of discussion heat. Because of the more developed economies, the denser populations and the more advanced information and communication technologies in the eastern coastal region, data transmission is generally faster, which allows more information to be disseminated (Sepehrdoust, 2018). Beijing had the greater ASF discussion volume, possibly because it is the capital of China and has many official registered Weibo accounts, which meant that great deal of ASF information was generated from here. However, there were also dense discussions in Liaoning, where the first ASF case in China was confirmed on 3 August 2018, and in Sichuan, which is a major pig breeding province and also one of the core pork supply areas. On 15 November 2018, the first ASF case in Sichuan was recorded, which had a significant impact on the pig industry and severely affected pork prices.
It was also observed that the number of Weibo posts by male users was significantly higher than posts by female users (Strekalova, 2015). There were several possible reasons for this finding. First, the 2018 Weibo user development report released by the Sina Weibo data center reported that there was a higher proportion of male Weibo users than female users. Second, men tend to have more confident, dominant discourse styles, while women are more cooperative and supportive (Hayat et al., 2017), which means that men are more likely to express their own opinions through their original posts, while women would tend to express their feelings more frequently in comments. These results, however, were contrary to Xie et al. (2017), possibly because males and females have different online discussion participation levels for different types of emergencies, that is, women are more likely than men to express their opinions on social media due to their strong sympathy for catastrophic events that cause harm to people’s lives (Liu et al., 2020).
Involvement of Different User Groups in the Discussion: Content Analysis
The content analysis addressed the following four research questions: (1) What were the keywords discussed and what were the keyword differences between the three user types? (2) What were the main topics discussed by different user types? (3) What were the similarities and differences between the topics discussed during the ASF and similar public health events? and (4) What were the emotional tendencies of different users and how did they change over time?
ASF Online Public Opinion Discussion Topic Analysis
Keyword Classification and Statistical Analysis
Word frequency is used to measure the importance of words in the text, with higher frequency words being more likely to represent issues of concern (Lam et al., 2019). As simple statistical key word frequency analyses are unable to explain the meaning of the words, the keywords in the previous studies (Corrales-Garay et al., 2019) have often classified epidemic related online public opinion into categories such as health risks, prevention, symptoms, transmission and treatment (Vijaykumar et al., 2018; Khatua et al., 2019). Therefore, in this study, the context factors were taken into account and four different categories identified: prevention, epidemic, disposal, and effect. First, the keywords from the three user groups were combined and the top 500 highest frequencies selected. However, “African swine fever” and words of the same or similar meaning were not included as these referred to the event itself and did not require any specific classification. Second, the key word classification was conducted simultaneously and independently by two graduate student groups familiar with the ASF event, after which the classification results were compared, discussed and finally determined, as shown in Table 2, with the associated value representing the word frequency as a percentage of the total frequency.
As can be seen, the public were most concerned about the “epidemic” topic in the ASF event, among which “epidemic” and “pig” accounted for more than 1%, and “occur” and “virus” accounted for more than 0.5%, which indicated that these keywords were the most discussed. For the two disposal and effect topics, the “Ministry of Rural Affairs” and “pork” were the most popular words with public ratios of 1.20% and 1.11%, which indicated that the response and handling measures by the Ministry of Rural Affairs toward pork safety and pricing were of public concern. To study the differences between the ASF social media discussions and other agricultural product safety incidents, the Weibo data related to this avian influenza in 2017 was mined and analyzed in the same way. The difference is that the avian influenza was most focused on “infect” and “case.” In contrast to the ASF, the avian influenza users paid less attention to the “effect” theme than to the “prevent” theme, possibly because ASF is not a zoonotic disease, which meant that users were more concerned about the living pigs being infected as well as the price of pork and other livelihood issues. However, avian influenza is a zoonotic disease that has a high death rate in humans (Mcaleer et al., 2010) and therefore requires strict prevention measures such as vaccines.
To better understand and compare the distribution differences between the four topics in the three user groups, the high-frequency words from the three user types were statistically analyzed. The threshold values for the high-frequency words were calculated based on Donohue (1973) classification method for high-frequency and low-frequency words, the formula for which is , where T is a boundary value for the high-frequency and low-frequency words, which is also known as the threshold value for the high-frequency words and represents the frequency of the last word in the high-frequency words, and is the number of keywords with a frequency of 1. The calculation results are shown in Table 3, from which it can be seen that the threshold values for the high-frequency words for the verified individual users, institutional users and ordinary users were 155.03, 153.23, and 240.76, respectively, and the corresponding high frequency words were 151, 226, and 351.
Based on the calculated threshold values for the high-frequency words, the distribution differences for the four topics by the three user groups were determined, as shown in Figure 5, in which the horizontal axis represents the percentage of high-frequency words for the four topics in the three user groups; the larger the percentage, the more attention the topic received; and the vertical axis represents the four topics that users paid most attention to.
As can be seen, the three-user group discussion topics were similar. First, the “epidemic” percentage was significantly higher than the other three topics at greater than 50%, which indicated that all three user groups were most concerned about the epidemic itself and the control of the development and spread of the epidemic by the relevant institutions (Vijaykumar et al., 2018). Second, the “effect,” “disposal” and “prevention” percentages were relatively low, with “effect” and “disposal” being only slightly higher than “prevent.” This may have been because the sudden first occurrence of the epidemic meant that no prevention work had been carried out on time, and also because as the ASF virus impacted pigs rather than people, less attention was paid to prevention work. The results also indicated how quickly and effectively the correct information was being disseminated compared to the Twitter content swine flu discussions in 2009, which revealed that Twitter users at that time believed that pigs and pork could host and/or transmit the virus (Ahmed et al., 2019).
The topic distribution varied slightly between the three user groups. The distributions for “effect” and “disposal” in the three user groups were similar, but there were some differences in the “epidemic” and “prevention” distributions. The “epidemic” percentage by institutional users (71.05%) was significantly higher than by ordinary users (57.72%), which indicated that the institutional users paid more attention to the ASF virus pig infection morbidity and death rate than the ordinary users, possibly because the institutional users were worried about the effects on China’s pig industry. Compared with the ordinary users (11.21%) and the verified individual users (8.84%), the “prevention” topic was of little concern to the institutional users (4.98%), which was in sharp contrast to their focus on the “epidemic.”
Semantic Correlation Analysis
1. Construct semantic association co-occurrence phrases
Words appearing simultaneously in Weibo texts possibly have some correlations. It is difficult to accurately grasp the public discussion real focus and the internal connection and correlation degree of each discussion topic simply by statistics of text subject words. If two words often appear together in different texts, it indicates that these texts have some common characteristics, with the higher the co-occurrence frequency, the higher the correlation degree. To explore the relationships between the keywords, the semantic association co-occurrence phrases were constructed for the three user types from which 210, 220 and 750 thousand co-occurrence phrases were, respectively, identified for the verified individual users, the institutional users, and the ordinary users. To better analyze the semantic correlations between the keywords, the top 200 co-occurrence phrases, all of which had over 40 co-occurrence frequencies, for each topic were selected. The top 10 co-occurrence phrases for each topic are shown in Table 4.
As can be seen, the concerns of the three user groups were similar, with semantic association co-occurrence phrases such as “Africa--swine fever,” “swine fever--epidemic” and “agriculture--Ministry of Rural Affairs” being common to all users and having a co-occurrence frequency greater than 2,800. This indicated that all users were focused on the ASF epidemic development and had paid attention to the ASF response and treatment measures by the Agricultural Ministry of Rural Affairs. It is worth noting that the viral spread of online information has led to the widespread monitoring of government actions, which puts enormous pressure on the government to respond swiftly. However, there were some focus differences between the three user groups; for example, the co-occurrence phrase “Shuanghui-- respond” was on the list for the verified individual users, which indicated that these users were concerned about the swine fever virus that had been detected in Shuanghui ham, a common food, and were paying close attention to the Shuanghui Group responses. However, institutional users and ordinary users appeared to be more concerned about the treatment of the pigs infected with ASF as evidenced in the co-occurrence phrase “harmless--dispose,” which, respectively, appeared over 3,800 and 5,000 times.
2. Visual analysis of the semantic association
To illustrate the concern differences in the three user groups more intuitively, semantic association visualizations were drawn, as shown in Figure 6, in which the nodes represent the keywords the users had paid attention to: the larger and darker the node, the higher the concern; with the edges representing the connections between the two words: the thicker and darker the edge, the more frequent the co-occurrence of phrases, and the stronger the connection.
From the discussions similarity among the three user groups, “ASF” occupied a core position in the semantic association graph, indicating that ASF had received a lot of attention from online users. The risk and uncertainty of a public health emergency may cause changes in public mood and behavior, causing unnecessary fear and anxiety, and thus triggering widespread discussion. The Weibo openness, communication and immediacy extended such events communication efficiency and audience channels (Yu et al., 2017). Another common concern among the three user groups was the “pig.” This is because pork accounts for a large portion of people’s daily diet (about two-thirds of the total meat consumption; Xie et al., 2020), and the pigs health and the pork safety had aroused widespread public concern. Another important topic associated with “ASF” was “epidemic,” which was similar to the research results in Xie et al. (2021). Because the social healthy development and economic life is closely related to every public, the ASF outbreak significant impact cannot be ignored. Controlling epidemic developments is fundamental to reducing the spread of rumors and reassuring the people. Therefore, it is urgent for relevant institutions to actively take effective measures to deal with the epidemic development.
From the discussion differences among the three user groups, as can be seen from Figure 6A, the “prevention and control” node was the second largest and strongly associated with “ASF,” which indicated that the verified individual users were most concerned about the prevention and control of ASF and hoped that the relevant institutions would take measures to eliminate or reduce the harm and spread of ASF. Xia et al. (2022) also found that the “epidemic prevention” topic received a lot of attention during the COVID-19 pandemic. Different from the verified individual users concern, “pig” was the core section concern of the institutional users, as shown in Figure 6B. The core nodes associated with “pig” were “number,” “death,” “diagnosis” and “morbidity,” which indicated that the institutional users were more concerned with the swine fever virus diagnosis, morbidity and death rate. This may have been because as the ASF resulted in significant losses to the pig industry, the government, the media and businesses were deeply concerned and were closely following the developments. As shown in Figure 6C, the keywords the ordinary user group most paid attention to were scattered. The main phrases associated with “pig” included “pig--breed,” “pig--product,” “pig--price,” and “cull—pig,” and the keywords associated with “pork” were mainly “price,” “product,” and “eat,” which indicated that the ordinary users were concerned about livelihood issues such as the sharp rise in pork prices caused by ASF and the safety of pork products. The small community composed of “Sanquan--dumplings,” “Sanquan--respond,” “dumplings--suspected,” and “Sanquan--food” was a new focus in the visualization network diagram for the ordinary users. This was because the detection of ASF in Sanquan dumplings resulted in a heated discussion by Weibo users. As Sanquan dumplings are widely sold across China and are eaten by ordinary users, it is not surprising that they paid significant attention to the event.
Evolution of User Emotions: Sentiment Analysis
Although subject analysis can identify the different user groups’ focus, it cannot reveal the different user groups emotional evolution trend, leading to the failure to effectively guide the online public opinion development. Therefore, after counting the sentiment analysis results over time, the ratio of negative emotion posts to all emotional posts from the different user groups were calculated and are shown in Figure 7, in which the horizontal axis represents time, the vertical axis represents the proportion of negative emotions, and the dotted line represents the negative emotion trends for the different user groups.
Over time, the negative emotions in all three user groups had a downward trend, which was similar to the research results in previous studies (Liu et al., 2022; Yang et al., 2022). With the implementation of prevention and control measures and the popularization of knowledge related to the epidemic, people’s fear was also being alleviated. The institutional users tended to have a lower proportion of negative emotions, possibly because they had access to the government agencies that were releasing the often positive and neutral messages. As the verified individual users tended to have fan bases and were opinion leaders in various fields of interest, although the proportion of negative emotions was high at the beginning of the outbreak, it decreased to a level similar to the institutional users as greater attention was being paid to response measures. However, due to the ordinary users’ varying knowledge and education, they tended to express their views only in relation to their own feelings, which meant there was a higher proportion of negative emotions (Vijaykumar et al., 2018).
Network Structure of the Users Participating in the Discussion: Social Network Analysis
The network analysis was focused on the following three research questions: (1) What was the structure of the ASF information dissemination network? (2) Which users were the main network opinion leaders? and (3) Which users were the network communication bridges?
Characteristics of the ASF Information Dissemination Network
To grasp the transmission characteristics of ASF online public opinion network as a whole, the overall network structure index was adopted. The original Weibo posters and their re-posters were imported into the social network analysis tool Gephi, and a network that had 33,666 nodes and 33,114 edges obtained, in which the nodes represented the users who sent out or received a reply, and the edges represented the relationships between those users. On this basis, the statistical function was used to calculate the average path length and network diameter. The average path length is used to measure the network connectivity. If it is less than 6, the network is considered to be closely connected. The results showed that the average path length was 1.47, which is significantly smaller than the boundary value 6, which indicated that the participants were about 1.5 nodes away from each other, which suggested that the participants discussing these themes were very close knit and had close professional proximity. The network diameter, which is the longest distance between any two nodes in the network, was used to measure the network public opinion influence. The network diameter was less than 11, indicating that the public opinion spread was wider and the impact was larger. The community detection method embedded in Gephi was used (Blondel et al., 2008) to reveal that there were more than 1,000 communities in the network, most of which were small. There were three large communities identified: the largest was the “headlines” community, with 39.23% of total users; the second largest was the “People’s Daily,” with 14.93% of total users; and the third largest was the “Xinhua viewpoint,” with 4.22% of users. To further analyze each user role in the public opinion communication network, each user was classified as either “News,” “Government official,” “Organization,” “Leader,” “Interest,” “Public” and “Unknown,” which were defined as in the following.
•News (N): news and media
•Government official (G): official news release platform
•Organization (O): official microblog of organization/institution
•Leader (L): elites of various communities
•Interest (I): Users focused on specific areas of interest
•Public (P): Individual Weibo users
•Unknown (UNK): Account closed or not accessible to retrieve the information
Opinion Leaders
In public opinion dissemination networks, the more reposted a user’s views, the more the user is recognized, and the more the user is being seen as an opinion leader in the public opinion network. Identifying opinion leaders in the public opinion communication network is conducive to guiding the public opinion development. Degree has often been used to measure the importance of a node in social network analysis (Hansen et al., 2011). The higher the degree, the more relationships a node has, the more it is in the network core position. Therefore, it is reasonable that degree was used to identify opinion leaders in public opinion communication network. As the large number of nodes and the out-degree of most nodes was 0, for a better visual representation, only the nodes with greater than 10 out-degrees are shown, with those having a greater than 100 out-degrees being marked (Table 5). The two most conspicuous nodes were “N 1” and “N 2,” which were media that had a huge fan base. Media were the most popular sources of information, followed by government and health agencies (Chew and Eysenbach, 2010; Mcneill et al., 2016; Guidry et al., 2017; Vijaykumar et al., 2018; Hellsten et al., 2019). Zhang et al. (2021) reached a similar conclusion, and Zhao et al. (2022b) also proved the opinion leader role in public opinion communication. In addition to the institutional users, the elites from various communities, such as the hosts, lawyers and food safety personnel, also act as opinion leaders in public opinion dissemination, most of which have certain fan bases and social reputations, which meant that their views are more likely to be recognized by ordinary users.
Communication Bridges
In public opinion dissemination networks, if the information needs to be transmitted to other users through some users, then these users act as network bridges or gatekeepers. To effectively identify the communication bridge in the network is conducive to controlling the public opinion trend. Betweenness centrality is often used to measure the ability of a node to control the communication between other nodes (Freeman, 1977; Oliveira and Gama, 2012). The higher the value is, the more obvious the bridge role is, so it was used to measure the bridge role in public opinion network. Similar to the opinion leader identification, nodes with a betweenness centrality greater than 10 are shown, and those greater than 100 marked in Table 6. The study of these nodes showed that star fans played very important roles in the dissemination of public opinion as they often paid attention to the Weibo messages and received the news very quickly. Besides, these star fans usually have a certain fan base and are the leaders in their fan group, other fans obtain relevant information through these leaders and then express their own opinions. Bloggers in different fields with a certain fan base and influential celebrities were also found to be the main information disseminators. People come together because of the same interests in such areas as movies, cartoons, and animals. When elites from different fields paid close attention to ASF events, they would publicly express their opinions, and fans would often agree with others’ views by reposting their posts, which further elevates their status as significant information diffusers.
Conclusions and Recommendations
In this study, content analysis was employed to analyze the online public opinions of different Weibo user groups during the “African swine fever” incident, and network analysis was used to analyze the online public opinion network dissemination characteristics, which contributed to the research field in three ways. First, automatic coding was applied using information mining on the Weibo ASF related users. Second, automatic content analysis methods: topic analysis, semantic network analysis, and sentiment analysis: were applied to study the ASF discussion developments in the three user groups and comparative analysis (three-user groups) of ASF with avian influenza in 2017(content analysis). Third, a network analysis method was applied to study the ASF public opinion network dissemination characteristics and identify the opinion leaders and dissemination bridges.
The following conclusions about the social media discussion on agricultural product safety incidents were made.
Generally speaking, the first occurrence place of agricultural product safety incident and the origin of related agricultural products generated the most intense discussions on the event, and there were more male commenters than female.
The subject word analysis found that the epidemic itself received the highest attention among the four topics, and the prevention topic gained less attention than the disposal and effect topic, which was different from other agricultural product safety incidents (such as avian influenza) that may infect people, which also showed that the dissemination of correct information was fast and effective. The semantic association analysis showed that all three user groups were very concerned about the epidemic development and the response and treatment measures being instigated by the Ministry of Agriculture and Rural Affairs. The verified individual users were most concerned about the prevention and control of the epidemic and play a supervisory role, the content discussed by the institutional users had a high degree of similarity, with the greatest concern being issues related to agricultural production such as morbidity and mortality. In addition to the prevention and control of the epidemic, the ordinary users were also very concerned about the price fluctuations of related agricultural products. What is more, both verified individual users and ordinary users were very concerned about food safety issues, the detection of a virus in the food would deal a serious blow to the brand, leading to the decline of consumer confidence. The emotional polarity found that the proportion of negative emotions was highest in the ordinary user group, decreased over time, and did not exceed 50%.
The network analysis found that media was the main sources of information about agricultural product safety incidents, and only a small amount of the information came from the government and public health institution. Presenters, lawyers and other elites from all walks of life, especially the food safety personnel acted as opinion leaders in the public opinion dissemination. Celebrity fans and bloggers with a certain fan base in different fields of interest such as movies and pets were also found to play a very important role in the dissemination of public opinion.
Therefore, from these results, the following recommendations are given.
First, it is suggested to determine the nature of the agricultural product safety incidents. When an agricultural product safety incident occurs, it is necessary to first make clear whether the consumption of agricultural products with virus will lead to human infection, and timely transmit the results to the public so that they can find a balance between a certain sense of tension and excessive worry. At the same time, information about the measures being taken by the relevant institutions (such as Ministry of Agriculture and Rural Affairs) should be released quickly to gain public trust.
Second, it is advised to take measures to stabilize prices of related agricultural products. Specifically, subsidies and compensations need to be provided to farmers/farms hit by the epidemic, so that they can survive the crisis; agricultural product reserve should be released to avoid a sustained rise in prices caused by demand exceeding supply. In addition, enterprises that use related agricultural products as raw materials should conduct strict quality checks to avoid losing public trust as results of agricultural product safety incidents.
Third, it is suggested to pay attention to the information dissemination role of institutions related to agricultural product safety incidents. When agricultural product safety incidents occur, the public gets most of the latest news from the media since institutions related to agricultural product safety incidents (such as the Ministry of Agriculture and Rural Affairs, public health organizations) own only several social media accounts and much fewer followers than major online media accounts. Therefore, it is necessary to spend effort on the daily operation of these accounts, so that they can become a two-way communication place for obtaining official news and receiving public opinions when agricultural product safety incidents occur. What is more, because the public tends to believe and agree with opinion leaders related to agricultural product safety incidents (such as food safety personnel), they can be used to correctly guide the discussion atmosphere.
The study has the following limitations. First, the analysis of social media discussions of different types of users was built on the basis of information collected about the senders of Weibo. Although users were classified as institutional users, certified individual users, and ordinary users, the definition of their identities was not very accurate because this certification was submitted for modification by users themselves on the basis of certain thresholds, e.g., certified individual users do not necessarily have more influence than ordinary users. Second, in the collection of repost relationships of Weibo users, due to the large span of the study period and the huge number of Weibo posts, they were divided into high, medium, and low heat periods, from which a social network based on repost relationships was established by random sampling, respectively. Such an approach only included a small portion of Weibo reposts, and thus may produce omissions in the subsequent analysis of key users of the spread of agricultural safety events through the network. Future research can address the above issues and improve the accuracy of data collection to obtain social media discussion results that are closer to the reality.
Data Availability Statement
The original contributions presented in the study are included in the article/supplementary material further inquiries can be directed to the corresponding author.
Author Contributions
YL: conceptualization, funding acquisition, and methodology QJ: data curation and software. QJ and YX: formal analysis, and writing—original draft. YH and YL: supervision and writing—review and editing. All authors contributed to the article and approved the submitted version.
Funding
This work was funded by: National Social Science Fund Project of China, grant number 20XZS020; Scientific Research Innovation Team Project of Neijiang Normal University, grant number 2021TD01; and Scientific Research Project of Tuojiang River Basin High-quality Development Research Center, grant number TJGZL2021-06.
Conflict of Interest
The authors declare that the research was conducted in the absence of any commercial or financial relationships that could be construed as a potential conflict of interest.
Publisher’s Note
All claims expressed in this article are solely those of the authors and do not necessarily represent those of their affiliated organizations, or those of the publisher, the editors and the reviewers. Any product that may be evaluated in this article, or claim that may be made by its manufacturer, is not guaranteed or endorsed by the publisher.
References
Abd-Alrazaq, A., Alhuwail, D., Househ, M., Hamdi, M., and Shah, Z. (2020). Top concerns of tweeters during the COVID-19 pandemic: infoveillance study. J. Med. Internet Res. 22:e19016. doi: 10.2196/19016
Ahmed, W., Bath, P. A., Sbaffi, L., and Demartini, G. (2019). Novel insights into views towards H1N1 during the 2009 pandemic: a thematic analysis of twitter data. Health Inf. Libr. J. 36, 60–72. doi: 10.1111/hir.12247
Assenov, Y., Ramírez, F., Schelhorn, S.-E., Lengauer, T., and Albrecht, M. (2007). Computing topological parameters of biological networks. Bioinformatics 24, 282–284. doi: 10.1093/bioinformatics/btm554
Aswani, R., Kar, A. K., Ilavarasan, P. V., and Dwivedi, Y. K. (2018). Search engine marketing is not all gold: insights from twitter and SEOClerks. Int. J. Inf. Manag. 38, 107–116. doi: 10.1016/j.ijinfomgt.2017.07.005
Attavanich, W., Mccarl, B. A., and Bessler, D. (2011). The effect of H1N1 (swine flu) media coverage on agricultural commodity markets. Appl. Econ. Perspect. Policy 33, 241–259. doi: 10.1093/aepp/ppr008
Bird, S., Klein, E., and Loper, E. (2009). Natural Language Processing With Python Cambridge, O'Reilly Media.
Blondel, V. D., Guillaume, J.-L., Lambiotte, R., and Lefebvre, E. (2008). Fast unfolding of communities in large networks. J. Stat. Mech. 10:10008. doi: 10.1088/1742-5468/2008/10/P10008
Carducci, A., Alfani, S., Sassi, M., Cinini, A., and Calamusa, A. (2011). Mass media health information: quantitative and qualitative analysis of daily press coverage and its relation with public perceptions. Patient Educ. Couns. 82, 475–478. doi: 10.1016/j.pec.2010.12.025
Chae, B. (2015). Insights from hashtag #supplychain and twitter analytics: considering twitter and twitter data for supply chain practice and research. Int. J. Product. Econ. 165, 247–259. doi: 10.1016/j.ijpe.2014.12.037
Chau, M., and Xu, J. (2012). Business intelligence in blogs: understanding consumer interactions and communities business intelligence in blogs: understanding consumer interactions and communities introduction. MIS Q. 36:1189. doi: 10.2307/41703504
Chen, Y., Zhang, Y. Z., Xu, Z. W., Wang, X. Z., Lu, J. H., and Hu, W. B. (2019). Avian influenza A (H7N9) and related internet search query data in China. Sci. Rep. 9:10434. doi: 10.1038/s41598-019-46898-y
Chew, C., and Eysenbach, G. (2010). Pandemics in the age of twitter: content analysis of tweets during the 2009 H1N1 outbreak. PLoS One 5:e14118. doi: 10.1371/journal.pone.0014118
Chmielewski, R., and Swayne, D. E. (2011). Avian Influenza: Public Health and Food Safety Concerns Annu. Rev. Food Sci. Technol. 2, 37–57. doi: 10.1146/annurev-food-022510-133710
Collier, N., Nguyen, S., and Ngoc, M. (2010). OMG U got flu? Analysis of shared health messages for bio-surveillance 2:S9. doi: 10.1186/2041-1480-2-S5-S9,
Corrales-Garay, D., Ortiz-De-Urbina-Criado, M., and Mora-Valentín, E.-M. (2019). Knowledge areas, themes and future research on open data: a co-word analysis. Gov. Inf. Q. 36, 77–87. doi: 10.1016/j.giq.2018.10.008
De Jonge, J., Van Trijp, H., Renes, R. J., and Frewer, L. J. (2010). Consumer confidence in the safety of food and newspaper coverage of food safety issues: a longitudinal perspective. Risk Anal. 30, 125–142. doi: 10.1111/j.1539-6924.2009.01320.x
Ding, H., and Zhang, J. (2010). Social media and participatory risk communication during the H1N1 flu epidemic: A comparative study. China Media Res. 6, 80–91.
Donohue, J. C. (1973). Understanding Scientific Literatures: A Bibliometric Approach. Massachusetts: MIT Press.
Feldman, R. (2013). Techniques and applications for sentiment analysis. Commun. ACM 56, 82–89. doi: 10.1145/2436256.2436274
Freberg, K., Palenchar, M. J., and Veil, S. R. (2013). Managing and sharing H1N1 crisis information using social media bookmarking services. Public Relat. Rev. 39, 178–184. doi: 10.1016/j.pubrev.2013.02.007
Freeman, L. C. (1977). A set of measures of centrality based on betweenness. Sociometry 40, 35–41. doi: 10.2307/3033543
Freeman, L. C., Roeder, D., and Mulholland, R. R. (1979). Centrality in social networks: II. Experimental results. Social. Netwks. 2, 119–141. doi: 10.1016/0378-8733(79)90002-9
Fung, I. C. H., Fu, K. W., Ying, Y. C., Schaible, B., Hao, Y., Chan, C. H., et al. (2013). Chinese social media reaction to the MERS-CoV and avian influenza A(H7N9) outbreaks. Infect. Dis. Poverty 2:31. doi: 10.1186/2049-9957-2-31
Gan, X., Chang, R., and Wen, T. (2018). Overcoming barriers to off-site construction through engaging stakeholders: A two-mode social network analysis. J. Clean. Prod. 201, 735–747. doi: 10.1016/j.jclepro.2018.07.299
Gao, R. B., Cao, B., Hu, Y. W., Feng, Z. J., Wang, D. Y., Hu, W. F., et al. (2013). Human infection with a novel avian-origin influenza A (H7N9) virus. N. Engl. J. Med. 368, 1888–1897. doi: 10.1056/NEJMoa1304459
Gao, F., Zhang, M., and Sadri, S. (2011). Newspapers use more sources compared to health blogs in H1N1/swine flu coverage. Newsp. Res. J. 32, 89–96. doi: 10.1177/073953291103200209
Garten Rebecca, J., Davis, C. T., Russell Colin, A., Shu, B., Lindstrom, S., Balish, A., et al. (2009). Antigenic and genetic characteristics of swine-origin 2009 A(H1N1) influenza viruses circulating in humans. Science 325, 197–201. doi: 10.1126/science.1176225
Griffith, J., Marani, H., and Monkman, H. (2021). COVID-19 vaccine hesitancy in Canada: content analysis of tweets using the theoretical domains framework. J. Med. Internet Res. 23:e26874. doi: 10.2196/26874
Gu, H., Chen, B., Zhu, H. H., Jiang, T., Wang, X. Y., Chen, L., et al. (2014). Importance of internet surveillance in public health emergency control and prevention: evidence from a digital epidemiologic study during avian influenza A H7N9 outbreaks. J. Med. Internet Res. 16:e20. doi: 10.2196/jmir.2911
Guidry, J. P. D., Jin, Y., Orr, C. A., Messner, M., and Meganck, S. (2017). Ebola on Instagram and twitter: how health organizations address the health crisis in their social media engagement. Public Relat. Rev. 43, 477–486. doi: 10.1016/j.pubrev.2017.04.009
Han, X. H., Wang, J. L., Zhang, M., and Wang, X. J. (2020). Using social media to mine and analyze public opinion related to COVID-19 in China. Int. J. Environ. Res. Public Health 17:2788. doi: 10.3390/ijerph17082788
Hansen, D. L., Shneiderman, B., and Smith, M. A. (eds.) (2011). “Chapter 3-Social Network Analysis: Measuring, Mapping, and Modeling Collections of Connections,” in Analyzing Social Media Networks with NodeXL (Boston: Morgan Kaufmann
Hassouneh, I., Radwan, A., Serra, T., and Gil, J. M. (2012). Food scare crises and developing countries: The impact of avian influenza on vertical price transmission in the Egyptian poultry sector. Food Policy 37, 264–274. doi: 10.1016/j.foodpol.2012.02.012
Hayat, T., Lesser, O., and Samuel-Azran, T. (2017). Gendered discourse patterns on online social networks: A social network analysis perspective. Comput. Human Behav. 77, 132–139. doi: 10.1016/j.chb.2017.08.041
Hellsten, I., Jacobs, S., and Wonneberger, A. (2019). Active and passive stakeholders in issue arenas: A communication network approach to the bird flu debate on twitter. Public Relat. Rev. 45, 35–48. doi: 10.1016/j.pubrev.2018.12.009
Hilton, S., and Hunt, K. (2011). UK newspapers' representations of the 2009-10 outbreak of swine flu: one health scare not over-hyped by the media? J. Epidemiol. Community Health 65, 941–946. doi: 10.1136/jech.2010.119875
Hou, Z., Cui, F., Meng, Y., Lian, T., and Yu, C. (2019). Opinion mining from online travel reviews: A comparative analysis of Chinese major OTAs using semantic association analysis. Tour. Manag. 74, 276–289. doi: 10.1016/j.tourman.2019.03.009
Househ, M. (2016). Communicating Ebola through social media and electronic news media outlets: A cross-sectional study. Health Informatics J. 22, 470–478. doi: 10.1177/1460458214568037
Hu, X., and Guo, S. (2018). Influences of major epidemic on pig market price—analysis of industrial shocks of African swine fever and the countermeasures. Price 12, 51–55. doi: 10.19851/j.cnki.cn11-1010/f.2018.12.011
Hussain, A., Tahir, A., Hussain, Z., Sheikh, Z., Gogate, M., Dashtipour, K., et al. (2021). Artificial intelligence-enabled analysis of public attitudes on Facebook and twitter toward COVID-19 vaccines in the United Kingdom and the United States: observational study. J. Med. Internet Res. 23:e26627. doi: 10.2196/26627
Jang, H. J., Rempel, E., Roth, D., Carenini, G., and Janjua, N. Z. (2021). Tracking COVID-19 discourse on twitter in North America: infodemiology study using topic modeling and aspect-based sentiment analysis. J. Med. Internet Res. 23:e25431. doi: 10.2196/25431
Jimenez-Sotomayor, M. R., Gomez-Moreno, C., and Soto-Perez-De-Celis, E. (2020). Coronavirus, ageism, and twitter: an evaluation of tweets about older adults and COVID-19. J. Am. Geriatr. Soc. 68, 1661–1665. doi: 10.1111/jgs.16508
Khatua, A., Khatua, A., and Cambria, E. (2019). A tale of two epidemics: contextual Word2Vec for classifying twitter streams during outbreaks. Inf. Process. Manag. 56, 247–257. doi: 10.1016/j.ipm.2018.10.010
Kim, J., Bae, J., and Hastak, M. (2018). Emergency information diffusion on online social media during storm Cindy in U.S. Int. J. Inf. Manag. 40, 153–165. doi: 10.1016/j.ijinfomgt.2018.02.003
Krippendorff, K. (2004). Content Analysis: An Introduction to its Methodology, Thousand Oaks, California, Sage.
Lam, J. C. K., Cheung, L. Y. L., Wang, S., and Li, V. O. K. (2019). Stakeholder concerns of air pollution in Hong Kong and policy implications: a big-data computational text analysis approach. Environ. Sci. Pol. 101, 374–382. doi: 10.1016/j.envsci.2019.07.007
Li, S., Liu, Z., and Li, Y. (2020). Temporal and spatial evolution of online public sentiment on emergencies. Inf. Process. Manag. 57:102177. doi: 10.1016/j.ipm.2019.102177
Lipizzi, C., Iandoli, L., and Ramirez Marquez, J. E. (2015). Extracting and evaluating conversational patterns in social media: A socio-semantic analysis of customers’ reactions to the launch of new products using twitter streams. Int. J. Inf. Manag. 35, 490–503. doi: 10.1016/j.ijinfomgt.2015.04.001
Liu, Y., Liu, S., Ye, D., Tang, H., and Wang, F. (2022). Dynamic impact of negative public sentiment on agricultural product prices during COVID-19. J. Retail. Consum. Serv. 64:102790. doi: 10.1016/j.jretconser.2021.102790
Liu, Y., Zhu, J., Shao, X., Adusumilli, N. C., and Wang, F. (2020). Diffusion patterns in disaster-induced internet public opinion: based on a Sina Weibo online discussion about the ‘Liangshan fire’ in China. Environ. Hazards 20, 163–187. doi: 10.1080/17477891.2020.1758608
Luoma-Aho, V., Tampere, P., and Vos, M. (2013). Monitoring the arenas of the swine flu discussion. J. Commun. Manag. 17, 239–251. doi: 10.1108/JCOM-11-2010-0069
Lyons, N., and Lăzăroiu, G. (2020). Addressing the COVID-19 crisis by harnessing internet of things sensors and machine learning algorithms in data-driven smart sustainable cities. Geopolit. Hist. Int. Relat. 12, 65–71. doi: 10.22381/GHIR12220209
Martínez-Rojas, M., Pardo-Ferreira, M. D. C., and Rubio-Romero, J. C. (2018). Twitter as a tool for the management and analysis of emergency situations: a systematic literature review. Int. J. Inf. Manag. 43, 196–208. doi: 10.1016/j.ijinfomgt.2018.07.008
Masri, S., Jia, J. F., Li, C., Zhou, G. F., Lee, M. C., Yan, G. Y., et al. (2019). Use of twitter data to improve Zika virus surveillance in the United States during the 2016 epidemic. BMC Public Health 19:761. doi: 10.1186/s12889-019-7103-8
Mcaleer, M., Huang, B.-W., Kuo, H.-I., Chen, C.-C., and Chang, C.-L. (2010). An econometric analysis of SARS and avian flu on international tourist arrivals to Asia. Environ. Model Softw. 25, 100–106. doi: 10.1016/j.envsoft.2009.07.015
Mcneill, A., Harris, P. R., and Briggs, P. (2016). Twitter influence on UK vaccination and antiviral uptake during the 2009 H1N1 pandemic. Front. Public Health 4:26. doi: 10.3389/fpubh.2016.00026
Mircică, N. (2020). Restoring public trust in digital platform operations: machine learning algorithmic structuring of social media content. Rev. Contemp. Philos. 19:85. doi: 10.22381/RCP1920209
Mollema, L., Harmsen, I., Broekhuizen, E., Clijnk, R., Melker, H., Paulussen, T., et al. (2015). Disease detection or public opinion reflection? Content analysis of tweets, other social media, and online newspapers During the measles outbreak in the Netherlands in 2013. J. Med. Internet Res. 17:e128. doi: 10.2196/jmir.3863
Muñoz, A. L., and Tabarés, E. (2022). Characteristics of the major structural proteins of African swine fever virus: role as antigens in the induction of neutralizing antibodies. A review. Virology. 571, 46–51. doi: 10.1016/j.virol.2022.04.001
Nair, M. R., Ramya, G. R., and Sivakumar, P. B. (2017). Usage and analysis of twitter during 2015 Chennai flood towards disaster management. Procedia Comput. Sci. 115, 350–358. doi: 10.1016/j.procs.2017.09.089
Neuman, W. R., Guggenheim, L., Jang, S. M., and Bae, S. Y. (2014). The dynamics of public attention: agenda-setting theory meets big data. J. Commun. 64, 193–214. doi: 10.1111/jcom.12088
Oliveira, M., and Gama, J. (2012). An overview of social network analysis. Wiley Interdiscip. Rev.: Data Min. Knowl. Discov. 2, 99–115. doi: 10.1002/widm.1048
Otte, E., and Rousseau, R. (2002). Social network analysis: a powerful strategy, also for the information sciences. J. Inf. Sci. 28, 441–453. doi: 10.1177/016555150202800601
Pang, B., and Lee, L. (2008). Opinion mining and sentiment analysis. Found. Trends Inf. Retr. 2, 1–135. doi: 10.1561/1500000011
Pop, R.-A., Săplăcan, Z., Dabija, D.-C., and Alt, M.-A. (2022). The impact of social media influencers on travel decisions: the role of trust in consumer decision journey. Curr. Issue Tour. 25, 823–843. doi: 10.1080/13683500.2021.1895729
Pourebrahim, N., Sultana, S., Edwards, J., Gochanour, A., and Mohanty, S. (2019). Understanding communication dynamics on twitter during natural disasters: A case study of hurricane Sandy. Int. J. Disaster Risk Reduct. 37:101176. doi: 10.1016/j.ijdrr.2019.101176
Sepehrdoust, H. (2018). Impact of information and communication technology and financial development on economic growth of OPEC developing economies. Kasetsart J. Soc. Sci. 40, 546–551. doi: 10.1016/j.kjss.2018.01.008
Shuang, K., Zhang, Z., Guo, H., and Loo, J. (2018). A sentiment information Collector–Extractor architecture based neural network for sentiment analysis. Inf. Sci. 467, 549–558. doi: 10.1016/j.ins.2018.08.026
Signorini, A., Segre, A. M., and Polgreen, P. M. (2011). The use of twitter to track levels of disease activity and public concern in the US during the influenza A H1N1 pandemic. PLoS One 6:e19467. doi: 10.1371/journal.pone.0019467
Simon, T., Goldberg, A., and Adini, B. (2015). Socializing in emergencies—a review of the use of social media in emergency situations. Int. J. Inf. Manag. 35, 609–619. doi: 10.1016/j.ijinfomgt.2015.07.001
Song, C., Guo, C. Y., Hunt, K., and Zhuang, J. (2020). An analysis of public opinions regarding take-away food safety: a 2015–2018 case study on Sina Weibo. Foods 9:511. doi: 10.3390/foods9040511
Stanley, A., and Kucera, J. (2021). Smart healthcare devices and applications, machine learning-based automated diagnostic systems, and real-time medical data analytics in COVID-19 screening, testing, and treatment. Am. J. Med. Res. 8, 105–117. doi: 10.22381/ajmr8220218
Stieglitz, S., Mirbabaie, M., Ross, B., and Neuberger, C. (2018). Social media analytics—challenges in topic discovery, data collection, and data preparation. Int. J. Inf. Manag. 39, 156–168. doi: 10.1016/j.ijinfomgt.2017.12.002
Strekalova, Y. (2015). Emergent health risks and audience information engagement on social media. Am. J. Infect. Control 44, 363–365. doi: 10.1016/j.ajic.2015.09.024
Sun, L., Pang, P. C.-I., and Si, Y. W. (2020). Roles of information propagation of Chinese microblogging users in epidemics: a crisis management perspective. Internet Res. 31, 540–561. doi: 10.1108/INTR-11-2019-0490
Thelwall, M., Buckley, K., and Paltoglou, G. (2011). Sentiment in Twitter events. JASIS 62, 406–418. doi: 10.1002/asi.21462
Tirkkonen, P., and Luoma-Aho, V. (2011). Online authority communication during an epidemic: a Finnish example. Public Relat. Rev. 37, 172–174. doi: 10.1016/j.pubrev.2011.01.004
Towers, S., Afzal, S., Bernal, G., Bliss, N., Brown, S., Espinoza, B., et al. (2015). Mass media and the contagion of fear: the case of Ebola in America. PLoS One 10:e0129179. doi: 10.1371/journal.pone.0129179
Vasterman, P. L. M., and Ruigrok, N. (2013). Pandemic alarm in the Dutch media: media coverage of the 2009 influenza A (H1N1) pandemic and the role of the expert sources. Eur. J. Commun. 28, 436–453. doi: 10.1177/0267323113486235
Vijaykumar, S., Nowak, G., Himelboim, I., and Jin, Y. (2018). Virtual Zika transmission after the first U.S. case: who said what and how it spread on twitter. Am. J. Infect. Control 46, 549–557. doi: 10.1016/j.ajic.2017.10.015
Wen, X. W., Sun, S. L., Li, L., He, Q. Y., and Tsai, F. S. (2019). Avian influenza-factors affecting consumers' purchase intentions toward poultry products. Int. J. Environ. Res. Public Health 16:4139. doi: 10.3390/ijerph16214139
Xia, H., An, W., Li, J., and Zhang, Z. (2022). Outlier knowledge management for extreme public health events: understanding public opinions about COVID-19 based on microblog data. Socio Econ. Plan. Sci. 80:100941. doi: 10.1016/j.seps.2020.100941
Xiang, X. L., Lu, X., Halavanau, A., Xue, J., Sun, Y. H., Lai, P. H. L., et al. (2021). Modern senicide in the face of a pandemic: an examination of public discourse and sentiment about older adults and COVID-19 using machine learning. J. Gerontol. B Psychol. Sci. Soc. Sci. 76, E190–E200. doi: 10.1093/geronb/gbaa128
Xie, R., Chu, S. K. W., Chiu, D. K. W., and Wang, Y. (2021). Exploring public response to COVID-19 on Weibo with LDA topic modeling and sentiment analysis. Data Inf. Manag. 5, 86–99. doi: 10.2478/dim-2020-0023
Xie, Y., Qiao, R., Shao, G., and Chen, H. (2017). Research on Chinese social media users’ communication behaviors during public emergency events. Telematics Inform. 34, 740–754. doi: 10.1016/j.tele.2016.05.023
Xie, D., Zhuo, L., Xie, P., Liu, Y., Feng, B., and Wu, P. (2020). Spatiotemporal variations and developments of water footprints of pig feeding and pork production in China (2004–2013). Agric. Ecosyst. Environ. 297:106932. doi: 10.1016/j.agee.2020.106932
Xu, G., Sarkar, A., Qian, L., Shuxia, Z., Rahman, M. A., and Yongfeng, T. (2022). The impact of the epidemic experience on the recovery of production of pig farmers after the outbreak-evidence from the impact of African swine fever (ASF) in Chinese pig farming. Prev. Vet. Med. 199:105568. doi: 10.1016/j.prevetmed.2022.105568
Yang, Y., Zhang, Y., Zhang, X., Cao, Y., and Zhang, J. (2022). Spatial evolution patterns of public panic on Chinese social networks amidst the COVID-19 pandemic. Int. J. Disaster Risk Reduct. 70:102762. doi: 10.1016/j.ijdrr.2021.102762
Yi, L., Tao, J., Tan, C., and Zhu, Z. (2019a). Avian influenza, public opinion and risk spillover: measurement, theory and evidence from China’s broiler market. Sustainability 11:2358. doi: 10.3390/su11082358
Yi, L., Tao, J., Zhu, Z., Tan, C., and Qi, L. (2019b). Food safety incident, public health concern, and risk spillover heterogeneity: avian influenza shocks as natural experiments in China's consumer markets. Int. J. Environ. Res. Public Health 16:4182. doi: 10.3390/ijerph16214182
Yu, L., Li, L., and Tang, L. (2017). What can mass media do to control public panic in accidents of hazardous chemical leakage into rivers? A multi-agent-based online opinion dissemination model. J. Clean. Prod. 143, 1203–1214. doi: 10.1016/j.jclepro.2016.11.184
Yuan, J., Chen, K., Li, W., Ji, C., Wang, Z., and Skibniewski, M. J. (2018). Social network analysis for social risks of construction projects in high-density urban areas in China. J. Clean. Prod. 198, 940–961. doi: 10.1016/j.jclepro.2018.07.109
Zhang, M., Qin, S., and Zhu, X. (2021). Information diffusion under public crisis in BA scale-free network based on SEIR model—taking COVID-19 as an example. Phys. A: Stat. Mech. Appl. 571:125848. doi: 10.1016/j.physa.2021.125848
Zhao, Y. X., Cheng, S. X., Yu, X. Y., and Xu, H. L. (2020). Chinese public's attention to the COVID-19 epidemic on social media: observational descriptive study. J. Med. Internet Res. 22:e18825. doi: 10.2196/18825
Zhao, J., He, H., Zhao, X., and Lin, J. (2022b). Modeling and simulation of microblog-based public health emergency-associated public opinion communication. Inf. Process. Manag. 59:102846. doi: 10.1016/j.ipm.2021.102846
Zhao, G., Li, T., Liu, X., Zhang, T., Zhang, Z., Kang, L., et al. (2022a). African swine fever virus cysteine protease pS273R inhibits pyroptosis by noncanonically cleaving gasdermin D. J. Biol. Chem. 298:101480. doi: 10.1016/j.jbc.2021.101480
Keywords: agricultural product safety incidents, African swine fever, social media, information dissemination, diffusion patterns
Citation: Jiang Q, Xue Y, Hu Y and Li Y (2022) Public Social Media Discussions on Agricultural Product Safety Incidents: Chinese African Swine Fever Debate on Weibo. Front. Psychol. 13:903760. doi: 10.3389/fpsyg.2022.903760
Edited by:
George Lazaroiu, Spiru Haret University, RomaniaReviewed by:
Aurel Pera, University of Craiova, RomaniaHelena De Carvalho Ferreira, Institute for Agricultural, Fisheries and Food Research (ILVO), Belgium
Fernando Costa Ferreira, University of Lisbon, Portugal
Copyright © 2022 Jiang, Xue, Hu and Li. This is an open-access article distributed under the terms of the Creative Commons Attribution License (CC BY). The use, distribution or reproduction in other forums is permitted, provided the original author(s) and the copyright owner(s) are credited and that the original publication in this journal is cited, in accordance with accepted academic practice. No use, distribution or reproduction is permitted which does not comply with these terms.
*Correspondence: Yibin Li, 20000429@njtc.edu.cn