ABSTRACT
We present the largest number of Milky Way sized dark matter halos simulated at very high mass (∼104/particle) and temporal resolution (5 Myr/snapshot) done to date, quadrupling what is currently available in the literature. This initial suite consists of the first 24 halos of the Caterpillar Project whose project goal of 60–70 halos will be made public when complete. We do not bias our halo selection by the size of the Lagrangian volume. We resolve ∼20,000 gravitationally bound subhalos within the virial radius of each host halo. Improvements were made upon current state-of-the-art halo finders to better identify substructure at such high resolutions, and on average we recover ∼4 subhalos in each host halo above 108
which would have otherwise not been found. The density profiles of relaxed host halos are reasonably fit by Einasto profiles (α = 0.169 ± 0.023) with dependence on the assembly history of a given halo. Averaging over all halos, the substructure mass fraction is
, and mass function slope is dN/d
. We find concentration-dependent scatter in the normalizations at fixed halo mass. Our detailed contamination study of 264 low-resolution halos has resulted in unprecedentedly large high-resolution regions around our host halos for our fiducial resolution (sphere of radius
Mpc). This suite will allow detailed studies of low mass dwarf galaxies out to large galactocentric radii and the very first stellar systems at high redshift (z
15).
Export citation and abstract BibTeX RIS
1. INTRODUCTION
Under the current paradigm of structure formation (White & Rees 1978) stellar halos of large galaxies such as the Milky Way are believed to be primarily formed as a result of the accumulation of tidal debris associated with ancient as well as recent and ongoing accretion events (Helmi 2008; Pillepich et al. 2015). In principle, the entire merger and star formation history of our Galaxy and its satellites can be probed with their stellar contents (i.e., the "fossil record"; Freeman & Bland-Hawthorn 2002) because this information is not only encoded in the dynamical distribution of the different Galactic components, but also in the stellar chemical abundance patterns (e.g., Font et al. 2006; Gómez et al. 2010).
To further map out the structure and composition of the various components of the Milky Way, large scale observational efforts are now underway. Several surveys such as rave (Steinmetz et al. 2006), segue (Yanny et al. 2009), apogee (Majewski et al. 2010), lamost (Deng et al. 2012), and galah (Freeman 2012) have collected medium-resolution spectroscopic data on some four million stars, primarily in the Galactic disk and stellar halo. There are also ongoing large-scale photometric surveys such as Pan-STARRS (Kaiser et al. 2010) and SkyMapper Southern Sky Survey (Keller et al. 2013) mapping nearly the entire sky. Soon, the gaia satellite (Perryman et al. 2001) will provide precise photometry and astrometry for another one billion stars.
Studies of individual metal-poor halo stars have long been used to establish properties of the Galactic halo, such as the metallicity distribution function, to learn about its history and evolution. More recently, the discoveries of the ultra-faint dwarf galaxies (with ) in the northern Sloan Digital Sky Survey and the southern Dark Energy Survey have shown them to be extremely metal-deficient systems which lack metal-rich stars with
. To some extent they can be considered counterparts to the most metal-poor halo stars. They extend the metallicity-luminosity relationship of the classical dwarf spheroidal galaxies down to
(Kirby et al. 2008), and due to their relatively simple nature, they retain signatures of the earliest stages of chemical enrichment in their stellar population(s). Indeed, the chemical abundances of individual stars in the faintest galaxies suggest a close connection to metal-poor halo stars in the Galaxy (Frebel & Norris 2015).
This comes at a time when there is still uncertainty over what role dwarf galaxies play in the assembly of old stellar halos because the true nature of the building blocks of large galaxies (e.g., Helmi & de Zeeuw 2000; Johnston et al. 2008; Gómez et al. 2010) are not yet fully understood. Nevertheless, observations of the, e.g., the Segue 1 ultra-faint dwarf suggest that these faintest satellites could be some of the the universe's first galaxies (presumably the building blocks) that survived until today (Frebel & Bromm 2012; Frebel et al. 2014). They would thus be responsible for the Milky Way's oldest and most metal-poor stars.
This wealth of observational results offers unique opportunities to study galaxy assembly and evolution and will thus strongly inform our understanding of the formation of the Milky Way. Along with it, the current dark energy plus cold dark matter paradigm (ΛCDM) can be tested at the scales of the Milky Way and within the Local Group. But to fully unravel the Galaxy's past and properties, theoretical and statistical tools need to be in place to make efficient use of data.
For over three decades now, numerical simulations of structure formation have consistently increased in precision and physical realism (see Somerville & Davé 2014 for a review). Originally, they began as a way to study the evolution of simple N -body systems (e.g., merging galaxies; Aarseth 1963; Toomre & Toomre 1972; White 1978 and globular clusters; Hénon 1961) but with the advent of better processing power and more sophisticated codes (e.g., Springel 2010; Bryan et al. 2014; Hopkins 2015), N-body solvers are now fully coupled to hydrodynamic solvers allowing for a comprehensive treatment of the evolution of the visible universe (e.g., Vogelsberger et al. 2014; Schaye et al. 2015).
The most efficient method of studying volumes comparable to the Local Group while maintaining accurate large scale, low-frequency cosmological modes is via the zoom-in technique (Katz et al. 1994; Navarro & White 1994). This technique allows one to efficiently model a limited volume of the universe at an extremely high resolution. Owing to the extreme dynamic range offered by such simulations, the inside of extremely low mass, gravitationally bound satellite systems can be studied alongside the hierarchical assembly of their host galaxy (e.g., Stadel et al. 2009). Gravity solvers which use hybrid tree-particle-mesh techniques (e.g., Gadget-2; Springel 2005) are ideally suited to carrying out such calculations on these scales. In addition to tailored codes for studying Milky Way sized halos, halo finders used for identifying substructure contained within them have also drastically improved over the past 30 years. Simple friends-of-friends (FoF) algorithms (e.g., Davis et al. 1985) have now evolved into parallel, fully hierarchical FoFs algorithms adopting six phase-space dimensions and one time dimension allowing shape-independent, and noise-reduced identification of substructure (Behroozi et al. 2013). These tools are very robust methods for accurately identifying bound substructures (e.g., Onions et al. 2012), though Behroozi et al. (2015) has recently highlighted the difficulty in connecting halos during merger events. These efforts demonstrate that only algorithms that combine phase-space and temporal information should be used.
Two primary groups have performed zoom-in N -body simulations of the growth of Milky Way sized halos in extremely high resolution—the Aquarius project of Springel et al. (2008) and the Via Lactea simulations of Diemand et al. (2008). While these works have been thoroughly successful and made it possible to quantify the formation of the stellar halo, for example, both the Aquarius and Via Lactea projects are limited in a number of respects.
The first of these is that they adopted the now observationally disfavored Wilkinson Microwave Anisotropy Probe's first set of cosmological parameters (WMAP-1, Spergel et al. 2003). The advent of the Planck satellite (Planck Collaboration et al. 2014) with three times higher resolution and better treatment of the astrophysical foreground (owing in large part to using nine frequency bands instead of five with WMAP) has allowed even more precise estimates of key cosmological parameters. In particular, the most crucial of these for accurate cosmological simulations are the baryon density (), the matter density (
), the dark energy density (
), the density fluctuations at 8
Mpc (
), and the scalar spectral index (ns). Dooley et al. (2014) showed through a systematic studies of structure formation using different cosmologies that the maximum circular velocities, formation, and accretion times of a given host's substructure are noticeably different between cosmologies.
in WMAP-1, for example, is much higher (
= 0.9 versus
= 0.83) which shifts the peak in cosmic star formation rate to lower redshift, resulting in slightly bluer galaxies at z = 0 (Jarosik et al. 2011; Guo et al. 2013; Larson et al. 2015).
The second major drawback and perhaps more significant is that the Aquarius and Via Lactea simulations were simply limited in number. The Aquarius project consists of six well-resolved Milky Way mass halos, while the Via Lactea study focused on only one such halo.
There exists significant halo-to-halo scatter in, e.g., the substructure shape and abundance owing to variations in accretion history and environment, (Springel et al. 2008; Boylan-Kolchin et al. 2010; Cooper et al. 2010), with the dispersion appearing significant (a factor , Lunnan et al. 2012). But based on such a small sample, the extent cannot be well-quantified, although determining the distributions of substructure properties of galaxy halos is critical for interpreting the various observations of dwarf galaxy populations of all large galaxies, including the Milky Way and Andromeda.
More recently, Garrison-Kimmel et al. (2014b) have produced a suite of 36 Milky Way halos (24 isolated analogs, 12 Local Group analogs; ELVIS suite) at a resolution of ∼105 per particle (∼Aquarius level-3). Studies using this suite have again highlighted the case for the too big to fail problem (Boylan-Kolchin et al. 2011) by showing that the so called "massive failures" (i.e., halos with
≥ 25 km s−1 that became massive enough to have formed stars in the presence of an ionizing background,
≥ 30 km s−1) do not disappear when larger numbers of halos across a range of host masses are simulated (Garrison-Kimmel et al. 2014b). Despite the ELVIS suite's utility, it unfortunately lacks the extra mass resolution required to study the formation of minihalos and very small dwarf galaxies (∼106
), both at the present day and their evolution since the epoch of reionization. Also, ELVIS is not suitable5
for using the particle tagging technique whereby a few percent of the central dark matter particles of accreting systems are assigned stellar properties to study the assembly of the stellar halo (e.g., Cooper et al. 2015). If we are to understand the origin of the first stellar systems (including their chemical constituents) and to locate their descendants at the present day, higher resolution as well as particle tagging is of critical importance.
While previous simulations all have their own merits and drawbacks, one issue prevalent across nearly all previous studies is that they introduced bias in selecting their halos. Usually halo candidates studied using the zoom-in technique meet three criteria: isolation, merger history, and Lagrangian volume. From a computational standpoint, if one can obtain a compact Lagrangian region, a quiet merger history, and keep the halo relatively isolated, the savings in CPU-hours can be immense. Ultimately, however, this three-pronged approach introduces a selection bias. While constructing a simulation with these three key criteria in place will generate an approximate Milky Way analogue, one will not gain an understanding of how the results from studying this halo will compare to halos more generally selected from a pool in the desired mass range (e.g., 1–2 × 1012).
The first requirement is that the halos have a quiescent merger history, which is usually defined by the host having no major merger since a given redshift, e.g., z = 1 (e.g., Springel et al. 2008). Constraining the merger history of a simulation suite severely limits the capabilities of reconstructing the formation history of the Milky Way. Indeed, by statistically contrasting observational data sets to mock data extracted from a set of Milky Way-like dark matter halos, coupled to a semi-analytical model of Galaxy formation Tumlinson (2009) and Gómez et al. (2012) showed the best-fitting input parameter selection strongly depends on the underlying merger history of the Milky Way-like galaxy. For example, even though for every dark matter halo it is always possible to find a best-fitting model that tightly reproduces the Milky Way satellite luminosity function, these best-fitting models generally fail to reproduce a second and independent set of observables (see Gómez et al. 2014). It is thus critical to sample a wide range of evolutionary histories. The second requirement that the Lagrangian volume of the halo's particles be compact also in part biases the merger history of the halo. For a fixed z = 0 virial mass, the smaller the Lagrangian volume of a halo, the less likely that halo will have a late major-merger event. This bias further compounds the aforementioned issues of selecting halos with quiet merger histories. Lastly, the isolation criteria preferentially selects halos in low density environments, resulting in decreased substructure (Ragone-Figueroa & Plionis 2007) and higher angular momentum (Avila-Reese et al. 2005; Lee 2006).
In light of all of these issues, we are motivated to create a comprehensive data set consisting of 60–70 dark matter halos of approximately Milky Way mass in extremely high spatial and temporal resolution with a more relaxed selection criteria to not just understand the origin and evolution of the Milky Way, but additionally how it differs to other galaxies of similar mass in general. Moreover, this new simulation set (unlike the Aquarius and Via Lactea which were very specific in nature) lends itself well to studying the substructure and stellar halos of ∼1012 galaxies such as those being studied in the recently completed ghosts survey (de Jong et al. 2007; Monachesi et al. 2013, 2015).
We call this simulation suite The Caterpillar Project6
owing to the similarity between each of the individual halos and how they work together toward a common purpose. Due to the extreme computational requirement for a project of this size (∼14M CPU hours and ∼700TB of storage), we are staggering our release. For this first paper, we focus on the general z = 0 properties of the first 24 halos of the Caterpillar suite in order to clearly demonstrate data integrity and utility. In Section 2, we outline the simulation suite parameters, numerical techniques, and halo properties. In Section 3 we present a variety of initial results drawn from the suite. In Section 4, we present our primary conclusions from our initial subset of halos. Lastly, we present an
2. THE CATERPILLAR SUITE
2.1. Simulation and Numerical Techniques
The Caterpillar suite was run using P-Gadget3 and Gadget4, tree-based N-body codes based on Gadget2 (Springel 2005). For the underlying cosmological model we adopt the ΛCDM parameter set characterized by a Planck cosmology given by, ,
,
, ns=0.96,
, and Hubble constant, H0 = 100 h km s−1 Mpc−1 = 67.11 km s−1 Mpc−1 (Planck Collaboration et al. 2014). All initial conditions were constructed using music (Hahn & Abel 2011). We identify dark matter halos via rockstar (Behroozi et al. 2013) and construct merger trees using Consistent-Trees (Behroozi et al. 2012). rockstar assigns virial masses to halos,
, using the evolution of the virial relation from Bryan & Norman (1998) for our particular cosmology. At z = 0, this definition corresponds to an overdensity of 104× the critical density of the universe. We have modified rockstar to output all particles belonging to each halo so we can reconstruct any halo property in post-processing if required. We have also improved the code to include iterative unbinding (see Section 2.5). In this work, we restrict our definition of virial mass to include only those particles which are bound to the halo.
2.2. Parent Simulation, Zoom-ins, and Contamination
Initially a parent simulation box (see Figure 1) of width 100 Mpc was run at
(
) effective resolution (see music/P-Gadget3 parameter files on project website) to select viable candidate halos for re-simulation (i.e., ∼10,000 particles per host). The candidates for re-simulation were selected via the following mass and isolation criteria.
- 1.
- 2.
- 3.No halos with
≥ 0.5 × Mhost within 2.8 Mpc (Karachentsev et al. 2004; Tikhonov & Klypin 2009). We currently avoid pairs in our sample owing to the difficulty of running them at our desired resolution at the present time. We have nevertheless selected equivalent pairs of our current isolated sample but will be examining those in future work.
Figure 1. Projected dark matter density at z = 0 of the parent simulation, from which the 70 Caterpillar halos were selected. The box width is 100 Mpc and the color represents the logarithm of the dark matter density. The colored circles correspond to the location of the first 24 Caterpillar halos. The color for a given halo is kept identical for all figures throughout this work.
Download figure:
Standard image High-resolution imageThis results in 2122 candidates being found (from an original sample of 6564 within the specified mass range). We use an extremely weak selection over merger history such that we require no halo to have had a major merger (1:3 mass ratio) since z = 0.05 (<5%). Our overall aim is to construct a representative sample of 1012 halos and not specifically require Milky Way analogs a priori as has been done in previous studies (Diemand et al. 2007; Springel et al. 2008; Stadel et al. 2009; Garrison-Kimmel et al. 2014b; Sawala et al. 2014). This also allows us to apply statistical tools to constrain semi-analytic models in future work (e.g., Gómez et al. 2014). We place our halos into three mass bins, with the largest number of halos centered on the most likely mass for the Milky Way (M200 =
, Piffl et al. 2014);
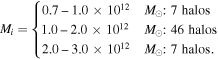
For this paper we are only considering a subset of the total sample in preparation, specifically 21 halos within the 1–2 × 1012 mass range and 3 halos within the
mass range.
2.3. Contamination Study
As has been highlighted by Onorbe et al. (2014), a great deal of care has to be taken when carrying out re-simulations of this kind so as to avoid contamination of the main halo of interest by low-resolution particles at z = 0. If mass from low resolution particles contributes more than ∼2% of the total host mass there can be offsets to estimates of the halo profile, shape, spin, and especially gas properties in hydrodynamic runs. To avoid contamination in our sample we custom built a Python GUI (using TraitsUI), Caterpillar Made Easy (cme), for running and analyzing cosmological simulations (for both single and multi-mass simulations). This tool allowed us to carry out an extensive contamination study (i.e., using ∼264 low resolution test halos with a particle mass of ∼107 ) specifically for the halos to be re-simulated. We have automated the monotony of constructing hundreds of qualitatively similar but quantitatively distinct cosmological simulations with the added benefit of being able to interactively select over initial condition parameters, cosmologies, halo finders and merger trees. This procedure was carried out self-consistently across all runs, allowing for a systematic study of which simulation parameters produce the most computationally inexpensive to run, uncontaminated halos.
Using cme we tested 11 Lagrangian geometries (e.g., convex hull, ellipsoid, expanded ellipsoids, cuboids, and expanded cuboids) so as to ensure a sphere of radius ∼1 Mpc exists of purely uncontaminated (high-resolution) particles centered on the host halo at z = 0. Our need to run 11 different Lagrangian geometries for each halo is motivated by the fact that the geometries vary substantially from halo to halo (due partially to their varied merger histories) and we wished to minimize the computation cost while achieving our contamination goals. It must also be highlighted that unlike many other studies, we did not select one Lagrangian geometry for all halos but used a specific geometry for a given halo depending on the needs of its simulation.
In Table 1 we show the various geometries we used for constructing our initial conditions. We modified music to be able to produce expanded Lagrangian volumes rather than the bounded volumes with which it was originally published. In Figure 2 we show four examples of Lagrangian geometries for halos selected for re-simulation. In some cases the geometries are reasonably compact, allowing for the traditional minimum cuboid enclosing to be used. Some larger regions, however, are extremely non-spherical (e.g., bottom right of Figure 2) and so a minimum ellipsoid was used. For each geometry we take the enclosed volume at z = 0 denoted by either and
. We run each of these halos to z = 0, run our modified rockstar and determine at what distance the closest low resolution or contamination particle (type = 2) resides in each case. With the knowledge that the high-resolution volume distance decreases at higher levels of refinement (Onorbe et al. 2014), we ensure a minimum contamination distance of ∼1
Mpc at z = 0 at our lowest resolution re-simulation, with the desire to have uncontaminated spheres of radius, ∼1 Mpc at our highest resolution re-simulation. In cases where four times the virial radius enclosure created contaminated halos but five times the virial radius created too large a simulation to run, we opted for an expanded ellipsoid of the minimum enclosing ellipsoid. In some cases there were a handful of offending particles far away from the primary Lagrangian volume (e.g., Figure 2 top right and bottom left) making no standard geometry enclosure feasible, expanded or otherwise. Here we trimmed the Lagrangian volume by hand and simulated the new geometry to z = 0 to ensure it had no contamination. Traditionally these types of halos are avoided but since we do not want to bias our sample, we dealt with complicated geometries in this specialized manner and have included them in our sample. Using this tailored approach, our highest resolution runs obtain very large, high-resolution regions with spheres of radius ∼1.4 ± 0.4 Mpc of solely high-resolution particles.
Figure 2. Sample Lagrangian volumes (at z = 127) of halos from the parent simulation. Some geometries are easily bounded by a minimum cuboid (e.g., top left) but others require an ellipsoid (e.g., bottom right). In some cases, there reside particles well away from the primary Lagrangian volume (e.g., top right and bottom left). For these difficult situations, we carefully trimmed the Lagrangian geometry to ensure no contamination of low resolution particles within 1 Mpc of the host at z = 0. Particles are color-coded by distance from the host at z = 0 where red particles are 5 ×
and blue particles are within the virial radius.
Download figure:
Standard image High-resolution imageTable 1. The Contamination Suite Used on the First Refinement Level (i.e., levelmax = 11) for Every Halo in the Caterpillar Suite
Name |
![]() |
Geometry | Factorb |
---|---|---|---|
CA4 | 4 | Convex Hull | ⋯ |
CA5 | 5 | Convex Hull | ⋯ |
EA4 | 4 | Bounded Ellipsoid | ⋯ |
EA5 | 5 | Bounded Ellipsoid | ⋯ |
EX4 | 4 | Expanded Ellipsoid | 1.05 |
EX5 | 5 | Expanded Ellipsoid | 1.05 |
EB4 | 4 | Expanded Ellipsoid | 1.1 |
EC4 | 4 | Expanded Ellipsoid | 1.2 |
BA4 | 4 | Minimum Cuboid | ⋯ |
BA5 | 5 | Minimum Cuboid | ⋯ |
BB4 | 4 | Expanded Cuboid | 1.1 |
Notes.
aThe multiple of the z = 0 virial radius that we used to construct the Lagrangian volume. bThe factor by which we increased the original volume (e.g., 1.2 means the ellipsoid was expanded by 20% in size. A dash represents the minimum ellipsoid/cuboid/hull exactly). These values were arbitrarily chosen with the only requirement being that the initial condition files were not overly large in size (i.e., a few hundred megabytes at lx11).Download table as: ASCIITypeset image
In Figure 3 we show box plots of the median contamination distance and respective quartiles for all 264 of our test halos using each of our selected geometries. Typically, the best performing geometry (i.e., the largest uncontaminated volume with the cheapest computational expense) was the expanded ellipsoid which enclosed all particles within 4 or 5 times the virial radius of the host in the parent simulation at z = 0.
Figure 3. Box plot of the distances to the first low resolution particle (particle type 2) for each of the prospective initial condition geometries (i.e., all run at levelmax = 11 in music, one level above our parent simulation). Table 1 contains the key for each geometry. The red lines indicate the median, edges of the box represent 25% and 75% quartiles and the outer tick marks represent the maximum and minimum distance. The dashed line represents the threshold we used to determine if a geometry was viable for a higher level re-simulation, though this was balanced against computational cost. We select the geometry which used the fewest CPU hours but maintained the largest uncontaminated volume.
Download figure:
Standard image High-resolution image2.4. Zoom-in Simulations
Starting from our parent simulation resolution, we re-simulated each halo at iteratively higher resolutions (a factor of 8x increase in particle number for each level) to ensure we did not obtain contaminated particles within the host halo (the uncontaminated volume shrinks with an increase in the ratio of the zoom-in resolution to that of the parent simulation resolution). In Figure 4, we show the dark matter distributions for iteratively higher resolutions of the same halo. One can clearly identify the same subhalos across all resolutions, indicating the qualitative success of our numerical techniques. Regarding computational resources, each halo at our highest resolution took between 150 and 300 K hours on TACC/Stampede and occupies ∼5–10 TB of storage for both the raw hdf5 snapshots and halo catalog. Table 2 shows the mass and spatial resolution for each of our refinement levels. Our softening length is 76
pc for our fiducial resolution.
Figure 4. Projected dark matter density at z = 0 of Cat-1 at successively higher resolutions (increasing by a factor of 8 in mass resolution each time) from left to right. The left panel is lx = 11 () and the rightmost panel is lx = 14 (
). The white circle represents the virial radius,
. The image brightness is proportional to the logarithm of the dark matter density squared (i.e., log(
).
Download figure:
Standard image High-resolution imageTable 2. The Resolution Levels of the Caterpillar Suite
lx | Np | mp | ![]() |
---|---|---|---|
(![]() |
(![]() |
||
15 | 327683 |
![]() |
38 |
14 | 163843 |
![]() |
76 |
13 | 80963 |
![]() |
152 |
12 | 40963 |
![]() |
228 |
11 | 20483 |
![]() |
452 |
10 | 10243 |
![]() |
904 |
Note.
lx represents represents the effective resolution (Np =(2X)3) of the high resolution region given by parameter, levelmax in music. mp is the particle mass and is the Plummer equivalent gravitational softening length. The parent simulation parameters are also shown in the last row. Only a select sample of halos are run at resolution level lx15. These runs will be presented in future works.
Download table as: ASCIITypeset image
We space our snapshots (320 per simulation) in the logarithm of the expansion factor until z = 6 (∼5 Myr/snapshot) and then linear in expansion factor down to z = 0 (∼50 Myr/snapshot). The motivation for this piecewise stitching of the two temporal schemes is twofold. At z > 6, we enter the era of mini-halo formation and the reionization epoch. If we wish to model the transport of Lyman–Werner (LW) radiation semi-analytically from the first mini-halos (e.g., Agarwal et al. 2012), we require a temporal resolution on par with the mean free path of LW photons in the intergalactic medium and the lifetime of a massive Population III star ( Myr). Second, we also wish to resolve the disruption of low mass dwarf galaxies at low redshift, which requires a temporal resolution of order ∼50 Myr (e.g., Segue I has a disruption timescale of ∼50 Myr). These timescales are also required if one is attempting to determine subhalo orbital pericenters that can be input into semi-analytic models of tidal disruption (e.g., Baumgardt & Makino 2003). While we intend to use the capabilities offered by finely sampled snapshots in future work, this initial paper primarily focuses on the z = 0 halo properties.
2.5. Iterative Unbinding In rockstar
rockstar is able to find any overdensity in 6D phase space, including both halos and streams. To distinguish gravitationally bound halos from other phase space structures, rockstar performs a single-pass energy calculation to determine which particles are gravitationally bound to the halo. Overdensities where at least 50% of the mass is gravitationally bound are considered halos, with the exact fraction a tuneable parameter (unbound_threshold) of the algorithm (Behroozi et al. 2013).
This definition is generally very effective at identifying halos and subhalos—but it fails in two important situations. First, if a subhalo is experiencing significant tidal stripping, the 50% cutoff can remove a subhalo from the catalog that should actually exist. We have found that changing the cutoff can recover the missing subhalos, but the best value of the cutoff is not easily determined. Second, rockstar is occasionally too effective at finding substructure in our high resolution simulations. In particular, it often finds velocity substructures in the cores of our halos that are clearly spurious based on their mass accretion histories and density profiles. Importantly, these two issues do not just affect low mass subhalos, but they can also add or remove halos with > 25 km s−1.
Both of these problems can be alleviated by applying an iterative unbinding procedure. We have implemented such an iterative unbinding procedure within rockstar. At each iteration, we remove particles whose kinetic energy exceeds the potential energy from other particles in that iteration. The potential is computed with the rockstar Barnes-Hut method (see Appendix B of Behroozi et al. 2013). We iterate the unbinding until we obtain a self-bound set of particles. Halos are only considered resolved if they contain at least 20 self-bound particles. All halo properties are then computed as usual, but with the self-bound particles instead of the one-pass bound particles. The iterative unbinding recovers the missing subhalos and removes most but not all of the spurious subhalos. Across 13 of our Caterpillar halos, we recover 52 halos with subhalo masses above 108 which would have otherwise been lost using the conventional rockstar. Figure 5 demonstrates how these large haloes can be recovered when our iterative unbinding procedure is used.
Figure 5. Density projection of the Cat-1 halo with subhalos with > 30 km s−1 highlighted by circles. The size of the circles corresponds to 3 ×
(i.e., the radius at which the velocity profile reaches its maximum). The white circles indicate subhalos found by the default parameters of the halo finding algorithm rockstar (i.e., without iterative unbinding). The three red circles indicate halos which are recovered when complete iterative unbinding is used. The largest outer white circle indicates the virial radius of the host halo.
Download figure:
Standard image High-resolution imageTo remove the remaining spurious subhalos, we also remove halos if (i.e., the radius at which the velocity profile reaches its maximum) of the subhalo is larger than the distance between the subhalo and host halo centers. The downside to adding iterative unbinding is that it increases the run time for rockstar by ∼50%. In the rest of this paper, we only consider subhalos with at least 20 self-bound particles passing the
cut. We define the subhalo mass,
, as the total gravitational bound mass of a subhalo which is obtained after the complete iterative unbinding procedure has been carried out.
3. RESULTS
3.1. Host Halo Properties
In Table 3, we provide the basic properties of our first 24 re-simulated halos. This includes the simulation name, the halo virial mass, the halo virial radius, concentration, maximum circular velocity, the radius at which the maximum circular velocity occurs, the formation time (defined as when the halo reaches half its present day mass), the redshift of the last major merger (1:3 mass ratio), the fraction of the host mass contained within subhalos, the axis ratios defining the halo shape, and the distance to the closest contamination particle from the host. We adopt a simple naming convention based on when the halos were post processed (1 – ). Where required, we use a shorthand reference to the resolution of the simulation. These refer to the parameter levelmax inside the IC generation code music (e.g., levelmax = 14 is simply lx14). This means lx14 represents an effective resolution of
(
level-2), lx13
(
level-3 or ∼ELVIS resolution), lx12
and lx11
. Unless otherwise stated, all halos in the analysis of this paper are the lx14 halos (i.e., our flagship resolution). All halos have similar z = 0 properties except Cat-7 whose properties can be tied to the fact that it has recently undergone a massive merger (1:3 mass ratio at z = 0.03). We obtain extremely large uncontaminated volumes (∼1.4 Mpc) in all but one of our halos (Cat-18 is ∼3% contaminated by mass). The fraction of mass held in subhalos across our sample is
(1σ), though this excludes Cat-7.
Table 3. The Halo Properties of the First 24 Caterpillar Halos
Halo |
![]() |
![]() |
ca |
![]() |
![]() |
zformc | zlmmd |
![]() |
c/a | b/a |
![]() |
---|---|---|---|---|---|---|---|---|---|---|---|
Name | (![]() ![]() |
(kpc) | (km s−1) | (kpc) | (Mpc) | ||||||
Cat-1 | 1.559 | 306.378 | 7.492 | 169.756 | 34.083 | 0.894 | 2.157 | 0.207 | 0.841 | 0.869 | 0.998 |
Cat-2 | 1.791 | 320.907 | 8.374 | 178.851 | 55.268 | 0.742 | 0.731 | 0.148 | 0.636 | 0.719 | 1.463 |
Cat-3 | 1.354 | 292.300 | 10.170 | 172.440 | 31.701 | 0.802 | 0.802 | 0.136 | 0.865 | 0.927 | 1.894 |
Cat-4 | 1.424 | 297.295 | 8.573 | 164.344 | 53.466 | 0.936 | 0.922 | 0.175 | 0.671 | 0.739 | 1.531 |
Cat-5 | 1.309 | 289.079 | 12.108 | 176.399 | 32.103 | 0.564 | 0.510 | 0.069 | 0.552 | 0.815 | 1.608 |
Cat-6 | 1.363 | 292.946 | 10.196 | 171.647 | 33.632 | 1.161 | 1.295 | 0.153 | 0.508 | 0.528 | 1.295 |
Cat-7 | 1.092 | 272.099 | 1.757 | 134.148 | 157.438 | 0.070 | 0.032 | 0.735 | 0.151 | 0.207 | 1.477 |
Cat-8 | 1.702 | 315.466 | 13.507 | 198.564 | 40.819 | 1.516 | 2.235 | 0.078 | 0.605 | 0.787 | 1.540 |
Cat-9 | 1.322 | 289.987 | 12.401 | 177.414 | 30.336 | 1.255 | 1.236 | 0.094 | 0.513 | 0.762 | 2.080 |
Cat-10 | 1.323 | 290.119 | 11.714 | 174.989 | 39.721 | 1.644 | 2.010 | 0.103 | 0.559 | 0.703 | 1.775 |
Cat-11 | 1.179 | 279.187 | 12.522 | 172.723 | 53.187 | 1.059 | 4.368 | 0.215 | 0.597 | 0.867 | 1.135 |
Cat-12 | 1.763 | 319.209 | 11.402 | 191.259 | 52.717 | 1.336 | 9.616 | 0.073 | 0.584 | 0.645 | 1.162 |
Cat-13 | 1.164 | 277.938 | 12.850 | 171.222 | 33.757 | 1.161 | 11.092 | 0.090 | 0.578 | 0.645 | 1.566 |
Cat-14 | 0.750 | 240.119 | 9.135 | 137.437 | 26.660 | 1.144 | 4.258 | 0.113 | 0.705 | 0.859 | 2.178 |
Cat-15 | 1.505 | 302.787 | 8.983 | 174.124 | 37.043 | 1.144 | 3.165 | 0.126 | 0.849 | 0.877 | 1.119 |
Cat-16 | 0.982 | 262.608 | 11.737 | 155.362 | 28.768 | 1.315 | 3.165 | 0.106 | 0.618 | 0.792 | 0.671 |
Cat-17 | 1.319 | 289.800 | 12.765 | 179.056 | 38.329 | 1.846 | 1.976 | 0.093 | 0.664 | 0.881 | 1.299 |
Cat-18 | 1.407 | 296.099 | 7.887 | 163.920 | 57.217 | 0.493 | 0.435 | 0.159 | 0.676 | 0.816 | 0.397 |
Cat-19 | 1.174 | 278.770 | 10.468 | 164.726 | 29.112 | 1.541 | 2.118 | 0.169 | 0.664 | 0.937 | 1.712 |
Cat-20 | 0.763 | 241.484 | 13.324 | 149.672 | 30.417 | 1.492 | 5.427 | 0.099 | 0.601 | 0.733 | 1.311 |
Cat-21 | 1.881 | 326.206 | 10.618 | 190.683 | 50.954 | 1.126 | 1.198 | 0.118 | 0.482 | 0.611 | 1.453 |
Cat-22 | 1.495 | 302.116 | 10.666 | 180.647 | 35.860 | 0.841 | 29.488 | 0.080 | 0.512 | 0.694 | 1.744 |
Cat-23 | 1.607 | 309.524 | 12.489 | 190.705 | 32.421 | 1.161 | 9.616 | 0.094 | 0.607 | 0.784 | 1.207 |
Cat-24 | 1.334 | 290.866 | 11.378 | 176.911 | 36.800 | 1.144 | 3.608 | 0.090 | 0.689 | 0.734 | 1.102 |
Meang | 1.368 | 291.791 | 10.903 | 173.167 | 38.886 | 1.144 | 4.410 | 0.121 | 0.634 | 0.771 | 1.402 |
±1σ | 0.285 | 21.610 | 1.761 | 13.441 | 9.530 | 0.329 | 6.112 | 0.041 | 0.103 | 0.102 | 0.409 |
Notes.
aConcentration defined by ratio of the virial radius and the scale radius;


Download table as: ASCIITypeset image
In Figure 6 we plot the concentration–mass (c–M) relation of the parent simulation for similarly sized halos (, gray band indicating the 1σ dispersion) and overlay the concentration (
/
) and host mass of the high resolution halos. This shows that for nearly all of the halos, we are sampling within 68% of the average c–M relation at a fixed halo mass. Again, Cat-7 is an outlier with an extremely low concentration because it recently underwent a major merger and has an extremely large substructure mass fraction, so its concentration is not meaningful. For this reason we do not include it in the quantitative analysis in terms of determining average halo profile shapes or the mass function slopes. Its properties are still shown and plotted in the various tables and figures, however. Recently, Buck et al. (2015) found that the thickness of planes of satellites depends on the concentration of the host halo. Specifically, they found the thinnest planes are only found in the most concentrated, and hence earliest formed halos. The fact that we sample relatively average concentrations for halos of this mass range means that it is less likely that these hosts will contain planes of satellites, or if they do, their thicknesses will be quite large (B. Griffin et al. 2016a, in preparation). As the Caterpillar sample grows, we will eventually sample overly concentrated halos, enabling us to see in better detail how this concentration-plane relation holds.
Figure 6. Concentration–mass relation for the 24 Caterpillar halos relative to those found in the parent simulation of similar mass. The concentration is defined as . Solid circles are the zoom-in simulations and the black line represents the concentration–mass relation drawn from the parent simulation for relaxed halos. The gray band is the 1σ dispersion in the c–M relation for halos in the parent simulation between
.
Download figure:
Standard image High-resolution image3.2. Visualizing The Halos and Their Assembly Histories
In Figures 7 and 8 we show images of the dark matter distribution in each of our 24 high-resolution halos at redshift z = 0. The brightness of each pixel is proportional to the logarithm of the dark matter density squared (i.e., log()projected along the line of sight. To enhance the density contrast, each panel has a different maximum density. We note that a similarly colored pixel in one panel does not necessarily mean the density is the same for another panel. The panel width is 1 Mpc and the local dark matter density of the particles in each pixel is estimated with an SPH kernel interpolation scheme based on the 64 nearest neighbor particles. Upon first inspection it is clear that each halo is littered with an abundance of dark matter substructures of varied shapes and sizes. In some cases, there are reasonably large neighbors (e.g., Cat-4, 7, 11, 24). By virtue of our selection criteria these neighbors are no larger than 0.5 ×
of the central host. In any case, in under a Gyr, these SMC/LMC sized systems (
) will likely undergo a major merger with the host galaxy.
Figure 7. Projected dark matter density at z = 0 of the first 12 Caterpillar halos with a box width of 1 Mpc. The image brightness is proportional to the logarithm of the dark matter density squared. Higher resolution images and animations are available at www.caterpillarproject.org.
Download figure:
Standard image High-resolution imageFigure 8. Projected dark matter density at z = 0 of each of the second set of 12 Caterpilar halos with a box width of 1 Mpc. The image brightness is proportional to the logarithm of the dark matter density squared. Higher resolution images and animations are available at www.caterpillarproject.org.
Download figure:
Standard image High-resolution imageIn Figure 9 we show the mass evolution of each of the halos. As highlighted by the inset which shows the normalized mass evolution, there is a wide variety of formation histories. In our initial catalog of 24 halos, 6 halos (Cat-2, 3, 4, 5, 7, 18) have had major mergers since z = 1. The halos going above a normalized mass ratio of 1.0 have had a halo pass through them relatively recently, which momentarily gives them extra mass such that it is larger than their z = 0 mass (e.g., Cat-2). This indicates that many of the halos are yet to reach an equilibrium state.
Figure 9. Mass evolution of the first 24 Caterpillar halos. The top panel shows each halo evolution along with the mean (black dashed) evolution for each of the halos. The inset panel shows the mass evolution normalized to the halo mass at z = 0. In the lower panel we show the mass evolution divided by the mean evolution to enhance individual features for each halo. We sample a diverse assembly history, from extremely quiet through to extremely violent (redshift of last major merger, z ∼ 0.07).
Download figure:
Standard image High-resolution imageAdopting the same criteria as Neto et al. (2007) we assess whether the host halos are relaxed. If their substructure mass fraction is below 0.1, their normalized offset between the center of mass of the halo (i.e., computed using all particles within ) and the potential center (
,
center of the potential well,
center of mass) is below 0.07 and their virial ratio (
) is below 1.35, then the host is considered relaxed. In Table 4 we provide the relaxed state of the halo. Many of the halos are in fact unrelaxed under this definition which is by design—we are sampling a wide range of assembly histories and so halos with recent merger events that prevent the halos from being fully virialized naturally make up part of our sample.
Table 4. The Relaxed Nature and Einasto Profile Parameters for the First 24 Caterpillar Halos
Halo | Relaxeda |
![]() |
![]() |
αd | Qmin | Qmin |
---|---|---|---|---|---|---|
Name | (×105) | Ein. | NFW | |||
Cat-1 | ⨯ | 9.929 | 27.182 | 0.128 | 0.039 | 0.103 |
Cat-2 | ✓ | 3.531 | 46.846 | 0.185 | 0.040 | 0.033 |
Cat-3 | ✓ | 11.604 | 25.309 | 0.151 | 0.028 | 0.067 |
Cat-4 | ⨯ | 5.504 | 34.962 | 0.154 | 0.039 | 0.080 |
Cat-5 | ✓ | 13.305 | 24.152 | 0.167 | 0.018 | 0.050 |
Cat-6 | ⨯ | 9.254 | 28.299 | 0.164 | 0.027 | 0.058 |
Cat-7 | ⨯ | 0.482 | 90.528 | 0.075 | 0.082 | 0.162 |
Cat-8 | ✓ | 8.027 | 34.555 | 0.236 | 0.033 | 0.036 |
Cat-9 | ✓ | 12.159 | 25.543 | 0.186 | 0.011 | 0.034 |
Cat-10 | ✓ | 14.482 | 23.492 | 0.168 | 0.029 | 0.049 |
Cat-11 | ⨯ | 10.617 | 25.450 | 0.173 | 0.049 | 0.071 |
Cat-12 | ✓ | 8.897 | 31.157 | 0.160 | 0.030 | 0.062 |
Cat-13 | ✓ | 11.842 | 25.010 | 0.187 | 0.009 | 0.036 |
Cat-14 | ✓ | 10.455 | 21.347 | 0.139 | 0.018 | 0.078 |
Cat-15 | ✓ | 8.905 | 28.744 | 0.139 | 0.025 | 0.078 |
Cat-16 | ✓ | 10.445 | 24.162 | 0.166 | 0.026 | 0.056 |
Cat-17 | ✓ | 11.283 | 26.799 | 0.195 | 0.019 | 0.026 |
Cat-18 | ⨯ | 6.998 | 31.012 | 0.150 | 0.023 | 0.082 |
Cat-19 | ⨯ | 9.079 | 26.881 | 0.164 | 0.032 | 0.054 |
Cat-20 | ✓ | 11.415 | 22.158 | 0.199 | 0.018 | 0.020 |
Cat-21 | ✓ | 6.682 | 36.486 | 0.175 | 0.025 | 0.043 |
Cat-22 | ✓ | 10.857 | 26.957 | 0.156 | 0.017 | 0.063 |
Cat-23 | ✓ | 13.486 | 26.024 | 0.172 | 0.018 | 0.039 |
Cat-24 | ✓ | 7.040 | 31.734 | 0.181 | 0.044 | 0.050 |
Meane | ⋯ | 9.817 | 28.446 | 0.169 | 0.027 | 0.055 |
±1σ | ⋯ | 2.6106 | 5.5677 | 0.023 | 0.010 | 0.020 |
Notes. For comparison, Qmin fits for NFW halo profiles are also listed.
aRelaxed criteria are based on those of Neto et al. (2007). If the substructure mass fraction is below 0.1, their center of mass displacement (






Download table as: ASCIITypeset image
3.3. Host Halo Profiles
In Figure 10 we plot the spherically averaged halo profiles for each of our 24 simulated halos. We draw the measured density profile as a thick line of a given color and continue the fit beyond the smallest radius possible set by Power et al. (2003) as a vertical black dashed line. We truncate each fit at this radius. There is a clear diversity in the profile shapes owing in part to the assembly histories of each halo. Halos which have undergone a recent major merger whose substructure mass fractions are higher than average are primarily dominated by a single subhalo (e.g., Cat-7 has a large subhalo at 200 kpc). The fitting formula we have used to describe the mass profile of our simulated halos follows the method of Navarro et al. (2010) and is given by the following Einasto form (over all particles within the virial radius):

Figure 10. Upper panel: normalized halo profiles for each of the host halos. Relaxed (solid lines) halos are better fit by Einasto profiles () over NFW profiles, though for halos that are unrelaxed (dashed lines), they are unable to be properly fit by NFW or Einasto profiles, which is in agreement with Neto et al. (2007) and Navarro et al. (2010). This inability stems purely from their definition, which a priori assumes that the halos are in virial equilibrium, which is clearly not the case for halos which have undergone a recent merger. The dashed black line indicates the Power et al. (2003) resolution limit as set by our softening length (also represented by a thinner density profile line). Lower panel: the log of the ratio between each of the Einasto fits and the data between the Power et al. (2003) radius and the virial radius.
Download figure:
Standard image High-resolution imageThe is the scale length of the halo which can be obtained without resorting to a particular fitting formula. We compute the logarithm of the slope profile and identify where a low-order polynomial fit to it intersects the isothermal value (
). Unlike the Navarro–Frenk–White profile (NFW) the peak parameter in the Einasto profile, α, is allowed to vary and thus provides a third parameter for the fitting formula. The best fitting parameters are found by minimizing the deviation between model and simulation at each bin. Specifically we minimize the function Q2, defined as

In this manner we find a function which clearly illustrates the deviation of the simulated and model profiles. In Table 4 we show our minimum Q2 parameter (Qmin), characteristic scale radius , and their corresponding densities for each halo. For our relaxed halos, Qmin for our Einasto fits are 0.027 ± 0.010 indicating reasonable agreement between the simulated and model Einasto profiles. This is better than our NFW profile fits for which we obtain
. The peak parameters for our Einasto fits are 0.169 ± 0.023, which is comparable to those of the Aquarius halos (α = 0.145–0.173) studied in Navarro et al. (2010). For halos which significantly deviate from the mean, it is important to note that those halos are not relaxed and so by definition will not provide meaningful Einasto/NFW fits. A more detailed study of the halo density profiles is reserved for future work.
3.4. Subhalo Properties
In Figure 11(a) we show the cumulative abundance of subhalos as a function of their maximum circular velocity for each Caterpillar halo. Since we achieve excellent convergence (see the end we find a variety of different sized subhalos for each host. Some hosts have only one 10 km s−1 subhalo, whereas another host halo has a large 70 km s−1 subhalo within the virial radius. Between 5–20 km s−1 all halos are very similar in their
function slopes within a slight offset owing to normalization stemming from the differences in host halo mass. At low
values (∼3 km s−1) we begin to lose completeness of our host subhalo sample due to lack of resolution. We additionally include in this figure the
function for subhalos at infall (i.e., when a subhalo first crosses the virial radius of the host). Since dynamical friction affects the highest mass subhalos the fastest, the biggest difference in the functions occurs at the high-mass end whereby several LMC sized systems (
>
) have been destroyed (over a timescale of 1–2 Gyr) between infall and z = 0. These large LMC sized-systems at infall can host anywhere from 4%–30% of the Milky Way sized halo's subhalos at z = 0 depending on their orbit and infall time (B. Griffen et al. 2016b, in preparation). In solid black we also plot the Aquarius Aq-A2 halo from Springel et al. (2008) (using the same version of rockstar that we used for the Caterpillar halos). We find the differences in the cosmology (
) and the slightly higher resolution of Aquarius leads to systematic differences in subhalo abundance.
Figure 11. (a) The cumulative abundance of subhalos as a function of their maximum circular velocity () for each of the Caterpillar halos. The solid lines represent subhalos at z = 0 and the dashed lines represent halos at infall (i.e., when they first cross the virial radius of the host). Many of the SMC/LMC sized systems (
) are destroyed by z = 0, though some large subhalos do survive. The Caterpillar suite is complete down to ∼4 km s−1. See our convergence study in the
there is small scatter in the subhalo abundances, as all of our hosts are very similar in mass. Over the range 106–8
we obtain a median mass function slope of −1.88 ± 0.1, slightly shallower than Aquarius (
). As we move to higher and lower mass regimes, there are differing abundances of large and small subhalos for each host. There are a number of systems with ∼109–10
halos within the virial radius of the host, making them possible Large/Small Magellanic Cloud analogs. Again, the solid black line is that of the Aquarius-A. (c) The subhalo mass fraction as a function of radius scaled by the virial radius of the host. The substructure mass is distributed similarly in nearly all halos, with the exception of Cat-7, which has undergone a major merger. There is more variability in the substructure mass fraction at low radii owing to the ongoing disruption most prevalent in the innermost dense regions of each host. (d) The normalized number of subhalos as function of radius scaled by the virial radius of the host. The number of subhalos as a function of distance is extremely self-similar across all halos except within the inner 10% of the virial radius of each host. This is in agreement with the findings of Springel et al. (2008) when factoring in their slightly higher resolution of the Aquarius suite and use of the observationally disfavored high value of
(
= 0.9).
Download figure:
Standard image High-resolution imageIn Figure 11(b), we show the subhalo mass functions for each of the halos. Our results are best fit by the power law dN/d, which is less steep than that found in the Aquarius halos of Springel et al. (2008). This slope is the best fit over the ranges
. We do observe a scatter in the subhalo abundances. This can be explained by the subtle concentration-subhalo-abundance relation whereby for fixed halo mass, there are more (less) subhalos belonging to hosts which are less (more) concentrated (e.g., Zentner et al. 2005; Watson et al. 2011; Mao et al. 2015). Indeed, we find halos that are more concentrated (see Figure 6) have lower normalizations than those less concentrated at fixed
. This is simply because halos that are more concentrated have formed earlier and so subhalos have spent substantially longer undergoing dynamical disruption within the host compared to similar sized subhalos orbiting less concentrated hosts.
In Figure 11(c) we show the subhalo radial mass fraction which indicates high variability in the contribution to the total halo mass from substructure as a function of galactocentric distance. For example, at 0.1, the total mass contributing to the host halo mass from substructure varies by a factor of 10 or more when normalized by mass. At
our substructure mass fraction varies by
(see Table 3 for exact fractions). Cat-7 has a large component of the halo mass in substructure at low radii because it has recently undergone a major merger (z = 0.03). Those halos with a large substructure mass fraction generally have had a recent major merger and are in the process of disrupting the recently accreted systems. On average, for a fixed fraction of the virial radius, the Caterpillar halos have less mass in substructure than that found in the Aq-A Aquarius halo (see solid black line, calculated using the exact same code). In Figure 11(d) we plot the normalized number of subhalos as a function of radius scaled by the virial radius of the host. We find the scatter in the number of subhalos as a function of galactocentric distance is a factor of 3 across all halos except within the inner 10% of the host halo where we are subject to noise in the halo finding produced by rockstar. Again, in solid black we also plot the Aquarius Aq-A2 halo from Springel et al. (2008). We find the differences in the cosmology (
) and in particular the slightly higher resolution of Aquarius lead to this systematic difference in the subhalo number density.
3.5. Too Big To Fail
We also examine halos which are massive enough to form stars but have no luminous counterpart in the nearby universe (i.e., the too big to fail problem, hereafter TBTF. Boylan-Kolchin et al. 2011). To do this we select halos with > 30 km s−1, which are subhalos large enough to retain substantial gas in the presence of an ionizing background and therefore theoretically should form stars. We follow the same definition as in Garrison-Kimmel et al. (2014a) to count two classes of halos. Strong massive failures (SMFs) are too dense to host any of the currently known bright MW classical dwarf spherioidals (dSph) galaxies. MFs include all SMFs as well as all massive subhalos which have densities consistent with the high-density dSphs (i.e., Draco and Ursa Minor) but cannot be associated with them without allowing a single dwarf galaxy to be hosted by multiple halos (i.e., assuming every observable dSph galaxy is hosted by exactly one halo). Most subhalos in the range of
= 25–30 km s−1 could host a low-density dwarf and as such are not defined as MFs.
In Figure 12, we plot a sample of the rotation curves for three different Caterpillar halos (Cat-19, Cat-13 and Cat-18). We adopt the Boylan-Kolchin et al. (2012) Einasto correction for pc, which differ from the ELVIS profile fits in that they extrapolate their entire profile from
with various density profile shapes. Black squares depict circular velocities of the classical dwarf galaxies (with luminosities above 2 × 105
), as measured by Wolf et al. (2010). Dashed–dotted cyan lines are LMC analogs (i.e.,
> 60 km s−1, which are excluded from our failure analysis), and blue dashed lines are MFs and red solid lines are SMFs. Thin black solid lines are subhalos which pass the test of having at least one observed dwarf with a comparable circular velocity, i.e., a circular profile goes through one of the observed dwarf galaxy data points. The cumulative numbers of profiles above and below the observed classical dwarfs for Cat-19 are 10 MFs, 5 SMFs and 1 LMC analogue. Similarly we find Cat-13 has 11 MFs and 8 SMFs. Cat-18 has the most failures of any halo with 21 MFs and 14 SMFs with 1 LMC analogue.
Figure 12. The rotation curves for all subhalos identified with a peak maximum circular velocity , above 30 km s−1 within 300 kpc of three different Caterpillar hosts. The black squares indicate observational constraints from dwarf galaxies from Wolf et al. (2010). We only include those observed systems whose luminosities are above 2 × 105
(i.e., showing only classical dwarfs and excluding ultra-faint dwarf galaxies). Dashed–dotted cyan lines are LMC analogs (i.e.,
> 60 km s−1), blue dashed lines are massive failures (MFs) and red solid lines are strong massive failures (SMFs). Thin black solid lines are subhalos that pass the check and have at least one observed dwarf with a comparable circular velocity (i.e., one halo passes through the circular velocity of a known classical dwarf determined by Wolf et al. 2010).
Download figure:
Standard image High-resolution imageIn Figure 13 we plot the number of strong and massive failures across all Caterpillar halos. Specifically we plot the fraction of hosts with fewer than N MFs and N SMFs within 300 kpc of each host as a function of N (black lines). Averaging over the entire Caterpillar sample (excluding Cat-7 as it has recently had a massive major merger), we predict 8 ± 3 (1σ) SMFs and 16 ± 5 (1σ) MFs within 300 kpc. If the Milky Way was well described by such an average we would expect to have these failures.
Figure 13. Cumulative fraction of massive failures (MFs) and strong massive failures (SMFs) across all Caterpillar halos (excluding Cat-7 which has undergone a recent massive major merger). We predict 8 ± 3 (1σ) strong massive failures and 16 ± 5 (1σ) massive failures within 300 kpc of the Milky Way. We also include the result from Garrison-Kimmel et al. (2014a) (i.e., using Einasto profiles) as a reference. The black lines are all failures that are detected by our version of rockstar that includes iterative unbinding. The blue lines are all failures found using a cut which mimics rockstar without iterative unbinding (see Section 3.5 for details). This indicates a fraction of failures are undergoing tidal disruption which may have been unaccounted for in the ELVIS subhalo catalogs.
Download figure:
Standard image High-resolution imageFor comparison, we plot the ELVIS MF and SMF counts (red lines). The lower resolution in these simulations requires an extrapolation of the velocity profile from and
using an analytic Einasto profile. Qualitatively, both simulation suites agree that there are a significant number of both MFs and SMFs. Quantitatively, there are several differences, which we now describe. The Caterpillar suite has many more MFs. This is due to our ability to better resolve high
subhalos that have been tidally stripped. In particular, the iterative unbinding procedure described in Section 2.5 removes the need for the rockstar unbound_threshold parameter (Behroozi et al. 2013). We can simulate the effect of the standard unbound_threshold = 0.5 cut by removing halos whose bound masses are less than 50% of their mass prior to unbinding. The MF counts with this cut are shown in Figure 13 by the blue lines, which are very similar to the ELVIS MF counts.
Figure 14. Convergence test for the Cat-2 and Cat-9 halos illustrating convergent halo profiles and functions, respectively. Panel (a): normalized halo profiles where the thick line represents the density profile above the radius defined by Power et al. (2003). The thin dashed line of the same color is the profile extrapolated down to the softening length. Panel (b): the cumulative abundance of subhalos as a function of their maximum circular velocity (
). These halos are representative of the convergence we achieve for all relaxed Caterpillar halos.
Download figure:
Standard image High-resolution imageThe Caterpillar suite also has significantly fewer SMFs compared to ELVIS. This discrepancy is likely due to the fact that we have measured rather than extrapolated the subhalo density profiles. Variations in the Einasto shape parameter (α) greatly affect the MF count (see Figure 4 of Garrison-Kimmel et al. 2014a). The Einasto fits to our density profiles have α typically closer to 0.2, which is less discrepant.
While TBTF is a prevalent problem in pure N-body simulations, many authors have indicated that the tension between the circular velocities of observed classical dwarfs and simulated subhalos can be alleviated with the addition of supernovae feedback and ram pressure stripping (e.g., Pontzen & Governato 2012; Zolotov et al. 2012; Brooks et al. 2013; Gritschneder & Lin 2013; Arraki et al. 2014; Del Popolo et al. 2014; Elbert et al. 2015; Maxwell et al. 2015) or by making dark matter self-interacting (e.g., Vogelsberger et al. 2012; Zavala et al. 2013). Our results are within 1σ of the number of failures found by Garrison-Kimmel et al. (2014a) (i.e., 12 MFs within 300 kpc), even when using a better density profile estimation.
Table 5. Halo Properties for the First Set of 12 Caterpillar Halos at Each Resolution
Name | Geometry | n![]() |
lx |
![]() |
![]() |
c |
![]() |
![]() |
![]() |
zlmm |
![]() |
b/c | c/a |
![]() |
---|---|---|---|---|---|---|---|---|---|---|---|---|---|---|
(1012![]() |
(kpc) | (km s−1) | (kpc) | (Mpc) | ||||||||||
Cat-1 | EA | 5 | 11 | 1.579 | 307.690 | 7.762 | 172.293 | 35.049 | 0.881 | 2.118 | 0.161 | 0.810 | 0.828 | 1.391 |
12 | 1.560 | 306.491 | 7.494 | 171.060 | 34.292 | 0.894 | 2.118 | 0.171 | 0.824 | 0.869 | 1.248 | |||
13 | 1.560 | 306.458 | 7.647 | 170.707 | 36.451 | 0.894 | 2.157 | 0.197 | 0.842 | 0.883 | 1.138 | |||
14 | 1.559 | 306.378 | 7.492 | 169.756 | 34.083 | 0.894 | 2.157 | 0.207 | 0.841 | 0.869 | 0.998 | |||
Cat-2 | EB | 4 | 11 | 1.807 | 321.876 | 7.621 | 176.924 | 64.931 | 0.742 | 0.719 | 0.092 | 0.596 | 0.724 | 1.577 |
12 | 1.782 | 320.357 | 8.575 | 179.069 | 53.856 | 0.742 | 0.719 | 0.112 | 0.607 | 0.716 | 1.522 | |||
13 | 1.792 | 320.970 | 8.382 | 178.753 | 54.360 | 0.742 | 0.731 | 0.137 | 0.643 | 0.731 | 1.480 | |||
14 | 1.791 | 320.907 | 8.374 | 178.851 | 55.268 | 0.742 | 0.731 | 0.148 | 0.636 | 0.719 | 1.463 | |||
Cat-3 | EB | 4 | 11 | 1.343 | 291.538 | 10.763 | 175.066 | 26.554 | 0.802 | 0.790 | 0.079 | 0.961 | 0.971 | 1.966 |
12 | 1.355 | 292.387 | 10.489 | 175.142 | 29.083 | 0.802 | 0.790 | 0.100 | 0.868 | 0.915 | 1.926 | |||
13 | 1.355 | 292.400 | 10.523 | 172.946 | 31.565 | 0.802 | 0.802 | 0.117 | 0.850 | 0.905 | 1.906 | |||
14 | 1.354 | 292.300 | 10.170 | 172.440 | 31.701 | 0.802 | 0.802 | 0.136 | 0.865 | 0.927 | 1.894 | |||
Cat-4 | EB | 4 | 11 | 1.503 | 302.676 | 8.308 | 169.309 | 91.132 | 0.894 | 0.908 | 0.128 | 0.749 | 0.825 | 1.791 |
12 | 1.415 | 296.632 | 9.208 | 168.170 | 85.989 | 0.922 | 0.922 | 0.120 | 0.681 | 0.762 | 1.594 | |||
13 | 1.434 | 298.009 | 8.434 | 164.999 | 59.225 | 0.936 | 0.922 | 0.156 | 0.673 | 0.743 | 1.561 | |||
14 | 1.424 | 297.295 | 8.573 | 164.344 | 53.466 | 0.936 | 0.922 | 0.175 | 0.671 | 0.739 | 1.531 | |||
Cat-5 | EB | 4 | 11 | 1.306 | 288.846 | 11.897 | 173.913 | 33.844 | 0.584 | 0.519 | 0.025 | 0.551 | 0.835 | 1.676 |
12 | 1.318 | 289.714 | 11.896 | 174.223 | 36.356 | 0.574 | 0.519 | 0.041 | 0.547 | 0.765 | 1.657 | |||
13 | 1.314 | 289.450 | 12.324 | 176.818 | 29.346 | 0.574 | 0.519 | 0.055 | 0.556 | 0.825 | 1.617 | |||
14 | 1.309 | 289.079 | 12.108 | 176.399 | 32.103 | 0.564 | 0.510 | 0.069 | 0.552 | 0.815 | 1.608 | |||
Cat-6 | EB | 4 | 11 | 1.371 | 293.516 | 10.373 | 172.100 | 32.944 | 1.144 | 1.275 | 0.094 | 0.495 | 0.534 | 1.708 |
12 | 1.347 | 291.848 | 10.522 | 172.873 | 30.622 | 1.161 | 1.275 | 0.116 | 0.496 | 0.525 | 1.495 | |||
13 | 1.366 | 293.186 | 10.086 | 170.858 | 32.794 | 1.161 | 1.295 | 0.138 | 0.510 | 0.529 | 1.300 | |||
14 | 1.363 | 292.946 | 10.196 | 171.647 | 33.632 | 1.161 | 1.295 | 0.153 | 0.508 | 0.528 | 1.295 | |||
Cat-7 | EB | 4 | 11 | 1.142 | 276.168 | 2.513 | 139.055 | 140.859 | 0.065 | 0.057 | 0.693 | 0.191 | 0.301 | 1.756 |
12 | 1.111 | 273.686 | 2.487 | 136.803 | 145.085 | 0.074 | 0.057 | 0.615 | 0.170 | 0.288 | 1.520 | |||
13 | 1.091 | 272.009 | 1.674 | 133.574 | 162.291 | 0.065 | 0.036 | 0.693 | 0.168 | 0.235 | 1.510 | |||
14 | 1.092 | 272.099 | 1.757 | 134.148 | 157.438 | 0.070 | 0.032 | 0.735 | 0.151 | 0.207 | 1.477 | |||
Cat-8 | EB | 4 | 11 | 1.729 | 317.150 | 13.081 | 198.577 | 46.800 | 1.541 | 2.195 | 0.032 | 0.602 | 0.768 | 1.690 |
12 | 1.716 | 316.337 | 13.154 | 198.229 | 39.671 | 1.315 | 2.195 | 0.053 | 0.594 | 0.775 | 1.597 | |||
13 | 1.701 | 315.450 | 13.340 | 197.637 | 39.810 | 1.516 | 2.235 | 0.066 | 0.599 | 0.791 | 1.550 | |||
14 | 1.702 | 315.466 | 13.507 | 198.564 | 40.819 | 1.516 | 2.235 | 0.078 | 0.605 | 0.787 | 1.540 | |||
Cat-9 | EB | 4 | 11 | 1.330 | 290.616 | 12.568 | 177.522 | 32.309 | 1.236 | 1.217 | 0.050 | 0.493 | 0.762 | 2.383 |
12 | 1.331 | 290.654 | 11.616 | 175.047 | 27.903 | 1.236 | 1.236 | 0.070 | 0.486 | 0.754 | 2.101 | |||
13 | 1.329 | 290.538 | 12.132 | 176.808 | 30.297 | 1.255 | 1.236 | 0.085 | 0.500 | 0.754 | 1.833 | |||
14 | 1.322 | 289.987 | 12.401 | 177.414 | 30.336 | 1.255 | 1.236 | 0.094 | 0.513 | 0.762 | 2.080 | |||
Cat-10 | EB | 4 | 11 | 1.319 | 289.809 | 11.902 | 175.553 | 41.894 | 1.699 | 2.010 | 0.052 | 0.561 | 0.709 | 1.983 |
12 | 1.332 | 290.764 | 11.439 | 174.479 | 29.806 | 1.516 | 2.010 | 0.069 | 0.551 | 0.679 | 1.870 | |||
13 | 1.328 | 290.477 | 11.714 | 175.124 | 25.839 | 1.644 | 2.010 | 0.088 | 0.559 | 0.703 | 1.740 | |||
14 | 1.323 | 290.119 | 11.714 | 174.989 | 39.721 | 1.644 | 2.010 | 0.103 | 0.559 | 0.703 | 1.775 | |||
Cat-11 | EB | 4 | 11 | 1.194 | 280.361 | 10.551 | 165.980 | 62.881 | 1.059 | 4.368 | 0.175 | 0.527 | 0.719 | 1.490 |
12 | 1.196 | 280.471 | 10.044 | 163.290 | 70.202 | 1.043 | 4.368 | 0.200 | 0.525 | 0.703 | 1.408 | |||
13 | 1.190 | 280.043 | 12.272 | 173.893 | 45.727 | 1.059 | 1.644 | 0.199 | 0.590 | 0.868 | 1.192 | |||
14 | 1.179 | 279.187 | 12.522 | 172.723 | 53.187 | 1.059 | 4.368 | 0.215 | 0.597 | 0.867 | 1.135 | |||
Cat-12 | EA | 5 | 11 | 1.786 | 320.627 | 11.723 | 191.564 | 59.256 | 1.336 | 2.542 | 0.034 | 0.592 | 0.724 | 1.664 |
12 | 1.749 | 318.388 | 11.824 | 192.085 | 56.859 | 1.336 | 2.542 | 0.042 | 0.572 | 0.686 | 1.342 | |||
13 | 1.767 | 319.441 | 11.663 | 191.320 | 49.435 | 1.336 | 2.542 | 0.062 | 0.571 | 0.703 | 1.239 | |||
14 | 1.763 | 319.209 | 11.402 | 191.259 | 52.717 | 1.336 | 9.616 | 0.073 | 0.584 | 0.645 | 1.162 |
Note. The resolution details for each refinement level (i.e., 11, 12, 13, 14) can be found in Table 2 and the geometry definitions can be found in Table 1.
Download table as: ASCIITypeset image
4. CONCLUSION
In this work we have presented the first results of the Caterpillar simulation project, whose goal is to better understand the formation of Milky Way-sized galaxies and their satellite companions at both high and low redshift. We have carried out 24 initial simulations in a Planck based ΛCDM cosmology. Although the total halo number will increase to 60–70 shortly, these first 24 halos provide us with an exquisite initial set of data to achieve our first set of science goals. In our approach, we have taken exceptional care to validate our numerical techniques. We quadruple the current number of halos available in the literature at this extremely high mass and temporal resolution, allowing for detailed statistical studies of the assembly of Milky Way-sized galaxies. We additionally have adjusted our simulation parameters to be more inclusive of potential scientific questions not yet studied in simulations of this size (i.e., decreasing the temporal resolution to ∼5 Myr/snapshot and increasing the volume resolved by high-resolution particles to 1–2 Mpc). The results presented above demonstrate our data quality and give initial clues at how halo properties vary across large numbers of realizations. Our initial key results can be summarized as follows.
- 1.Key halo properties such as the halo profile, mass functions, and substructure fractions are intimately connected to each halo's overall assembly history. Halos which have undergone recent major mergers have profiles which are poorly fit by either the NFW or Einasto profile. For those halos which are well fit by Einasto profiles, they have peak α values of 0.169 ± 0.023. Excluding the Cat-7 halo, we find a
, indicating reasonable agreement with Einasto fits of the Aquarius halos.
- 2.The abundance of dark matter subhalos remains relatively similar across our sample when normalized to host halo. As such, our halo mass functions are best fit by a simple power law, dN/d
. The scatter in the normalizations of the mass functions is due to the concentration-subhalo abundance relation for fixed halo mass (i.e., our more concentrated halos exhibit lower normalizations for fixed
).
- 3.Regarding TBTF, dividing halos into two categories of MFs and SMFs we predict 8 ± 3 (1σ) SMFs and 16 ± 5 (1σ) MFs within 300 kpc of the Milky Way.
- 4.Iterative unbinding in rockstar must be included to properly recover all bound subhalos this resolution. We recover 52 halos above 108
across a sample of 13 Caterpillar halos (∼4 per host halo) using iterative unbinding which would have otherwise been unaccounted for using traditional rockstar. This means that a small fraction of massive subhalos undergoing heavy tidal disruption may be unaccounted for in studies using traditional rockstar (e.g., the ELVIS halo catalogs).
This paper outlines the data products of the Caterpillar simulations and sets the foundation of many upcoming in-depth studies of the Local Group. Through our statistical approach to the assembly of Milky Way-sized halos we will gain a more fundamental insight into the origin and formation of the Galaxy, its similarly sized cousins, and their respective satellites.
BG would like to thank Paul Hsi for assistance with the compute cluster at MKI. The collaboration would also like to thank Phillip Zukin for his early contributions to the Caterpillar project. The collaboration also would like to thank Paul Hsi for assistance with the compute cluster at MKI. B.G. would like to thank Paul Torrey for helpful discussions. The authors thank Oliver Hahn for making the initial conditions code, music, publicly available. The authors also thank Volker Springel for making gadget-2 publicly available and for providing a version of gadget-3/gadget-4 for our use. The authors thank Peter Behroozi for making rockstar and consistent-trees publicly available and additionally thank him for technical support in modifying rockstar. Support for this work was provided by XSEDE through the grants TG-AST120022, TG-AST110038. B.G. and A.F. acknowledges support of the compute cluster of the Astrophysics Division which was built with support from the Kavli Investment Fund, administered by the MIT Kavli Institute for Astrophysics and Space Research. G.D. acknowledges support by NSF Grant 1122374. B.W.O. and F.G. were supported through the NSF Office of Cyberinfrastructure by grant PHY-0941373 and by the Michigan State University Institute for Cyber-Enabled Research (ICER). B.W.O. was supported in part by by NSF grant PHY 08-22648 (Physics Frontiers Center/Joint Institute for Nuclear Astrophysics) and NSF Grant PHY-1430152 (JINA Center for the Evolution of the Elements). A.F. acknowledges support from the Silverman (1968) Family Career Development professorship.
APPENDIX: CONVERGENCE STUDY
In Figure 14 we plot the halo profiles (Cat-2) and maximum circular velocity functions (Cat-9) at all our resolutions. We find our halos are well converged down to ∼0.2% of . In the case of the
functions, we find we are converged down to ∼4 km s−1 at our highest resolution. When normalized to the host halo virial velocity, the halos are in excellent agreement with one another. Halos were re-simulated at successively higher and higher mass and spatial resolution from the initial parent volume. In each instance, care was taken to ensure all halo properties were numerically converged (provided that quantity was not resolution limited, e.g., halo shape). In Tables 5 and 6 we show the same quantities as in Table 3 from the text but this time include the lower resolution halo properties. A full resolution study will be provided at the website, http://www.caterpillarproject.org when the LX15 runs have been completed.
Table 6. Halo Properties for the Second Set of 12 Caterpillar Halos at Each Resolution
Name | Geometry | n![]() |
lx |
![]() |
![]() |
c |
![]() |
![]() |
![]() |
zlmm |
![]() |
b/c | c/a |
![]() |
---|---|---|---|---|---|---|---|---|---|---|---|---|---|---|
(1012![]() |
(kpc) | (km/s) | (kpc) | (Mpc) | ||||||||||
Cat-13 | EB | 4 | 11 | 1.168 | 278.303 | 12.664 | 169.603 | 31.214 | 1.180 | 11.092 | 0.042 | 0.595 | 0.652 | 2.069 |
12 | 1.171 | 278.509 | 12.979 | 170.750 | 31.408 | 1.161 | 14.748 | 0.063 | 0.575 | 0.634 | 1.742 | |||
13 | 1.163 | 277.896 | 13.052 | 171.892 | 34.163 | 1.161 | 15.750 | 0.073 | 0.580 | 0.655 | 1.634 | |||
14 | 1.164 | 277.938 | 12.850 | 171.222 | 33.757 | 1.161 | 11.092 | 0.090 | 0.578 | 0.645 | 1.566 | |||
Cat-14 | EC | 4 | 11 | 0.744 | 239.430 | 9.526 | 137.580 | 42.772 | 1.180 | 4.155 | 0.060 | 0.714 | 0.851 | 2.516 |
12 | 0.757 | 240.865 | 8.854 | 136.512 | 27.875 | 1.144 | 4.258 | 0.086 | 0.709 | 0.849 | 2.301 | |||
13 | 0.754 | 240.529 | 9.148 | 137.266 | 44.395 | 1.144 | 4.258 | 0.097 | 0.694 | 0.842 | 2.234 | |||
14 | 0.750 | 240.119 | 9.135 | 137.437 | 26.660 | 1.144 | 4.258 | 0.113 | 0.705 | 0.859 | 2.178 | |||
Cat-15 | EX | 5 | 11 | 1.501 | 302.562 | 8.950 | 173.834 | 31.210 | 1.144 | 3.165 | 0.072 | 0.897 | 0.912 | 1.669 |
12 | 1.497 | 302.281 | 9.223 | 174.792 | 33.832 | 1.144 | 3.165 | 0.089 | 0.897 | 0.926 | 1.630 | |||
13 | 1.504 | 302.755 | 9.077 | 174.431 | 36.520 | 1.144 | 3.165 | 0.111 | 0.837 | 0.861 | 1.597 | |||
14 | 1.505 | 302.787 | 8.983 | 174.124 | 37.043 | 1.144 | 3.165 | 0.126 | 0.849 | 0.877 | 1.119 | |||
Cat-16 | EB | 4 | 11 | 0.993 | 263.614 | 10.997 | 154.748 | 42.280 | 1.315 | 3.165 | 0.053 | 0.567 | 0.765 | 1.406 |
12 | 0.976 | 262.082 | 12.099 | 156.589 | 28.820 | 1.315 | 3.165 | 0.072 | 0.593 | 0.791 | 1.393 | |||
13 | 0.980 | 262.447 | 11.888 | 156.193 | 29.498 | 1.315 | 3.165 | 0.088 | 0.597 | 0.766 | 1.384 | |||
14 | 0.982 | 262.608 | 11.737 | 155.362 | 28.768 | 1.315 | 3.165 | 0.106 | 0.618 | 0.792 | 0.671 | |||
Cat-17 | EX | 4 | 11 | 1.311 | 289.204 | 13.216 | 178.671 | 38.818 | 1.846 | 1.943 | 0.038 | 0.646 | 0.794 | 1.525 |
12 | 1.314 | 289.456 | 12.906 | 178.676 | 39.713 | 1.846 | 1.943 | 0.057 | 0.680 | 0.875 | 1.427 | |||
13 | 1.329 | 290.487 | 12.505 | 178.763 | 38.717 | 1.846 | 1.976 | 0.084 | 0.657 | 0.863 | 1.333 | |||
14 | 1.319 | 289.800 | 12.765 | 179.056 | 38.329 | 1.846 | 1.976 | 0.093 | 0.664 | 0.881 | 1.299 | |||
Cat-18 | EX | 4 | 11 | 1.428 | 297.536 | 7.909 | 167.184 | 32.058 | 0.451 | 0.427 | 0.100 | 0.677 | 0.847 | 1.491 |
12 | 1.414 | 296.559 | 7.861 | 164.702 | 48.041 | 0.459 | 0.412 | 0.123 | 0.720 | 0.840 | 1.397 | |||
13 | 1.400 | 295.596 | 7.823 | 165.164 | 40.766 | 0.493 | 0.435 | 0.141 | 0.622 | 0.712 | 1.228 | |||
14 | 1.407 | 296.099 | 7.887 | 163.920 | 57.217 | 0.493 | 0.435 | 0.159 | 0.676 | 0.816 | 0.397 | |||
Cat-19 | EX | 5 | 11 | 1.179 | 279.143 | 10.467 | 164.816 | 34.292 | 1.566 | 2.693 | 0.113 | 0.640 | 0.857 | 1.933 |
12 | 1.174 | 278.788 | 10.158 | 163.679 | 34.514 | 1.566 | 2.693 | 0.132 | 0.668 | 0.919 | 1.861 | |||
13 | 1.177 | 279.002 | 10.139 | 163.868 | 30.433 | 1.541 | 2.118 | 0.149 | 0.672 | 0.933 | 1.800 | |||
14 | 1.174 | 278.770 | 10.468 | 164.726 | 29.112 | 1.541 | 2.118 | 0.169 | 0.664 | 0.937 | 1.712 | |||
Cat-20 | BB | 4 | 11 | 0.765 | 241.720 | 13.409 | 150.030 | 25.189 | 1.516 | 5.588 | 0.045 | 0.608 | 0.743 | 1.677 |
12 | 0.756 | 240.683 | 13.443 | 148.881 | 27.312 | 1.541 | 5.588 | 0.053 | 0.634 | 0.775 | 1.521 | |||
13 | 0.761 | 241.208 | 13.456 | 149.682 | 29.340 | 1.516 | 5.761 | 0.084 | 0.613 | 0.752 | 1.377 | |||
14 | 0.763 | 241.484 | 13.324 | 149.672 | 30.417 | 1.492 | 5.427 | 0.099 | 0.601 | 0.733 | 1.311 | |||
Cat-21 | EX | 4 | 11 | 1.865 | 325.250 | 11.820 | 193.253 | 42.842 | 1.144 | 1.198 | 0.042 | 0.456 | 0.584 | 1.551 |
12 | 1.876 | 325.890 | 10.950 | 191.015 | 54.116 | 1.109 | 1.161 | 0.075 | 0.475 | 0.637 | 1.426 | |||
13 | 1.889 | 326.663 | 10.465 | 189.607 | 57.507 | 1.126 | 1.198 | 0.103 | 0.472 | 0.590 | 1.342 | |||
14 | 1.881 | 326.206 | 10.618 | 190.683 | 50.954 | 1.126 | 1.198 | 0.118 | 0.482 | 0.611 | 1.453 | |||
Cat-22 | EX | 5 | 11 | 1.560 | 306.489 | 9.356 | 177.811 | 33.807 | 0.828 | 5.940 | 0.044 | 0.496 | 0.643 | 2.003 |
12 | 1.594 | 308.677 | 9.799 | 181.703 | 44.919 | 0.790 | 5.940 | 0.052 | 0.461 | 0.637 | 1.903 | |||
13 | 1.497 | 302.257 | 10.655 | 180.773 | 37.743 | 0.854 | 5.940 | 0.068 | 0.518 | 0.695 | 1.837 | |||
14 | 1.495 | 302.116 | 10.666 | 180.647 | 35.860 | 0.841 | 29.488 | 0.080 | 0.512 | 0.694 | 1.744 | |||
Cat-23 | EX | 4 | 11 | 1.608 | 309.596 | 11.989 | 189.267 | 33.023 | 1.180 | 10.062 | 0.051 | 0.635 | 0.845 | 1.623 |
12 | 1.604 | 309.328 | 12.865 | 191.457 | 32.232 | 1.180 | 9.616 | 0.071 | 0.589 | 0.729 | 1.236 | |||
13 | 1.613 | 309.926 | 12.135 | 190.191 | 31.524 | 1.161 | 9.616 | 0.080 | 0.602 | 0.763 | 1.245 | |||
14 | 1.607 | 309.524 | 12.489 | 190.705 | 32.421 | 1.161 | 9.616 | 0.094 | 0.607 | 0.784 | 1.207 | |||
Cat-24 | EB | 4 | 11 | 1.329 | 290.537 | 11.152 | 174.259 | 43.136 | 1.217 | 2.801 | 0.038 | 0.651 | 0.705 | 1.260 |
12 | 1.323 | 290.054 | 11.326 | 175.088 | 48.435 | 1.217 | 2.801 | 0.052 | 0.645 | 0.674 | 1.396 | |||
13 | 1.335 | 290.969 | 11.490 | 177.313 | 34.438 | 1.144 | 2.801 | 0.077 | 0.675 | 0.721 | 1.190 | |||
14 | 1.334 | 290.866 | 11.378 | 176.911 | 36.800 | 1.144 | 3.608 | 0.090 | 0.689 | 0.734 | 1.102 |
Note. The resolution details for each refinement level (i.e., 11, 12, 13, 14) can be found in Table 2 and the geometry definitions can be found in Table 1.
Download table as: ASCIITypeset image
Footnotes
- 5
Particle tagging usually requires 1%–5% of the most bound particles of a satellite to be tagged. For a simulation which resolves 108
hosts with ∼1000 particles (i.e., ELVIS), this means one can only use a single particle to contain all the baryonic information, which is insufficient for modeling multiple stellar populations.
- 6
Project Website: http://www.caterpillarproject.org